Abstract
Purpose
Productivity is often cited as a key barrier to the adoption of metal laser-based powder bed fusion (ML-PBF) technology for mass production. Newer generations of this technology work to overcome this by introducing more lasers or dramatically different processing techniques. Current generation ML-PBF machines are typically not capable of taking on additional hardware to maximise productivity due to inherent design limitations. Thus, any increases to be found in this generation of machines need to be implemented through design or adjusting how the machine currently processes the material. The purpose of this paper is to identify the most beneficial existing methodologies for the optimisation of productivity in existing ML-PBF equipment so that current users have a framework upon which they can improve their processes.
Design/methodology/approach
The review method used here is the preferred reporting items for systematic review and meta-analysis (PRISMA). This is complemented by using an artificial intelligence-assisted literature review tool known as Elicit. Scopus, WEEE, Web of Science and Semantic Scholar databases were searched for articles using specific keywords and Boolean operators.
Findings
The PRIMSA and Elicit processes resulted in 51 papers that met the criteria. Of these, 24 indicated that by using a design of experiment approach, processing parameters could be created that would increase productivity. The other themes identified include scan strategy (11), surface alteration (11), changing of layer heights (17), artificial neural networks (3) and altering of the material (5). Due to the nature of the studies, quantifying the effect of these themes on productivity was not always possible. However, studies citing altering layer heights and processing parameters indicated the greatest quantifiable increase in productivity with values between 10% and 252% cited. The literature, though not always explicit, depicts several avenues for the improvement of productivity for current-generation ML-PBF machines.
Originality/value
This systematic literature review provides trends and themes that aim to influence and support future research directions for maximising the productivity of the ML-PBF machines.
Keywords
Citation
McConnell, S., Tanner, D. and Kourousis, K.I. (2024), "Productivity improvement opportunities for metal powder bed fusion technologies: a systematic literature review", Rapid Prototyping Journal, Vol. 30 No. 11, pp. 230-245. https://doi.org/10.1108/RPJ-09-2023-0333
Publisher
:Emerald Publishing Limited
Copyright © 2024, Sean McConnell, David Tanner and Kyriakos I. Kourousis.
License
Published by Emerald Publishing Limited. This article is published under the Creative Commons Attribution (CC BY 4.0) licence. Anyone may reproduce, distribute, translate and create derivative works of this article (for both commercial and non-commercial purposes), subject to full attribution to the original publication and authors. The full terms of this licence may be seen at http://creativecommons.org/licences/by/4.0/legalcode
List of Terminology
- Contour
-
= A defined closed path that traces the outermost region of a 2D slice;
- D10, D50, D90
-
= The respective percentile for describing the size distribution of powder particles;
- Design of Experiments (DOE)
-
= A systematic method to study relationships between multiple variables;
- Down-Skin
-
= Surfaces of a component facing downward relative to the print orientation;
- Hatch
-
= The path the laser takes to cover the desired area of the 2D slice;
- Island
-
= A closed region of a 2D slice;
- Neural network
-
= A process of using a series of algorithms to recognise relationships in data;
- Power
-
= The laser output at a system level, denoted in watts;
- Response surface methodology (RSM)
-
= A method to explore the relationships between variables that can define the maximum and minimum values of said relationship;
- Shell and Core
-
= Core describes the centre region of the component being produced; shell is the outer region;
- Sidewalls
-
= Any vertical sections at the edge of an island;
- Taguchi method
-
= Statistical method used to optimise a design; and
- Up-Skin
-
= Surfaces of a component facing upward relative to the print orientation.
Nomenclatures
- AM
-
= Additive manufacturing;
- ANN
-
= Artificial neural network;
- CCD
-
= Central composite design;
- CPTi
-
= Commercially pure titanium;
- DfAM
-
= Design for additive manufacturing;
- DOE
-
= Design of experiments;
- ED
-
= Energy density;
- FLG
-
= Few-layer graphene;
- IOB
-
= Iron oxide black;
- KW
-
= Keyword;
- LB-PBF
-
= Laser-based powder bed fusion;
- ML-PBF
-
= Metal laser-based powder bed fusion;
- OEM
-
= Original equipment manufacturer;
- PBF
-
= Powder bed fusion;
- RQ
-
= Research question;
- RSM
-
= Response surface method;
- SiC
-
= Silicon carbide;
- SLR
-
= Systematic literature review; and
- VED
-
= Volumetric energy density.
1. Introduction
Additive manufacturing (AM) is a technology that joins a material feedstock in a layer-by-layer process, resulting in a near-net shape part or product (Gibson et al., 2010). This technique has been around since the 1980s and has come to prominence in recent years as dependent technologies, such as lasers and computational power, have become sufficiently advanced and commercially viable to allow for it to be a practical means of production (Korpela et al., 2020). Metal laser-based powder bed fusion, ML-PBF, is a subset technology of AM, it uses a laser to deliver energy to the material and powder as the material feedstock.
ML-PBF machines have generated sales of over two thousand systems valued at €1.089bn in 2020. This was a reduction from 2019 and has been attributed to the COVID-19 pandemic (Wohler, 2021). Concerning conventional machining and forging applications, ML-PBF can be considered slower and thus more cost-prohibitive (Attaran, 2017; UKRI, 2020). This means it can be less competitive when producing simple geometric designs (Salem et al., 2020; Schnabel et al., 2017). In ML-PBF, the relatively poor surface finish (Clemens Lieberwirth et al., 2017; Obilanade et al., 2021; Udroiu et al., 2019) and the effect this has on material properties for certain applications (Ruppert et al., 2017; Zadpoor, 2019) can mean that this technique has high added costs due to the level of post-processing required (Gordon et al., 2016; Kumbhar and Mulay, 2018). This is also coupled with the perceived poor material mechanical properties that can occur as a result of poor processing. Thus, products produced will often require expensive thermal treatments to rectify any internal defects or undesirable microstructural characteristics (du Plessis and Macdonald, 2020; Harkin et al., 2018). Limited material choices with this technology are also seen as a barrier to adoption, but also as a market opportunity for new materials to be developed and become widely available for ML-PBF machines (Buranich et al., 2020; Chekotu et al., 2019; Sohrabi et al., 2021).
The above, coupled with the high acquisition cost for equipment, has led to the slow adoption of this technology by some industries, especially for mass production purposes (Marak et al., 2019; Sobota et al., 2020). Although the cost and efficiency of ML-PBF machines are improving, the amortisation of the capital cost is still a significant factor in determining if companies can adopt AM (Atzeni and Salmi, 2012; Di and Yang, 2021; Ruffo et al., 2006; Thomas and Gilbert, 2014).
The barriers outlined coalesce around a theme of the processing capabilities of ML-PBF. This is to say that both system limitations and user inputs will indicate the cost of production via throughput, the resultant material properties and the level of post-processing required. Based on this and the preceding paragraph, the most appropriate research question for this systematic literature review is:
How can further optimisation of the productivity of metal laser powder bed fusion machines be achieved for industry-specific metal alloys to overcome barriers to adoption?
This research question is formed around several keywords and intentions that will contribute significantly to understanding the opportunities for this technology. It narrows down the specific process, ML-PBF, highlights the success indicators and productivity, and then finally focuses on the processing of industrially relevant materials.
2. Methodology
From reviewing existing systematic literature reviews in this space and the research question; SCOPUS (Scopus, 2024), Web of Science (WoS) (Web of Science, 2024) and IEEE (IEEE Xplore, 2024) were selected as the most appropriate databases, due to their wide use in the research and academic community.
The method used to achieve this is the preferred reporting items for systematic review and meta-analysis (PRISMA) (Page et al., 2021). The research question was broken down into several keywords (KW) that drive the search string terms. The KW are listed in Table 1. KW1 is indicative of umbrella terms for the manufacturing process along with more specific acronyms. The terms in question were taken from the ISO standard for AM terminology (ISO/TC 261, 2021). KW2 was originally designated to isolate the material identified in the research question; however, given the niche nature of the technology and, in particular, the material, this keyword was expanded to include the most common materials processed by AM. KW3 is a collection of terms that the research question was centralised around. KW4 was added to the string to avoid returning papers that are irrelevant to the research question. The operators, fields and filters were altered to suit each database’s format.
Phase one of the process involved running the search string through the databases as indicated in Table 1, which returned 4,804 results. Phase two involved the removal of duplicates; as such, 1,725 results were removed. The majority of duplicates occurred between SCOPUS and WoS as a result of journals shared across both databases.
Phase three processed the remaining 3,079 results by screening the titles and abstracts for their relevance to the research question of this study. This filtered out 3,005 results. The reasons for this were that the material or manufacturing process not being related to the research question. Phase four then took the remaining 74 results and conducted the full-text screening. A total of 28 were filtered out by how closely they addressed the research question, and particularly how they addressed productivity.
Phase five took the remaining 46 results and analysed the themes within each with respect to the research question. The complete screening process is illustrated in Figure 1.
2.1 Artificial intelligence-assisted complimentary search
As the PRISMA process involves the interpretation of the research question by the researcher to ensure the KW and semantics of the questions are captured by the search string, there are possibilities for the string not to fully capture the intent of the question and thus miss out on literature that may be critical. Following the initial PRISMA review process, it became evident that tools such as artificial intelligence (AI) software may be able to cover any gaps caused by the interpretation of the research question into a search string. As such, several tools were investigated; these are listed and briefly described in Table 2. Following a review of the capabilities and accessibility of each, it was decided to use the Elicit tool (Elicit, 2024) as it offers transparency to the AI functions and algorithms used.
Taking the user’s research question, Elicit (Elicit, 2024) passes it through the Semantic Scholar Academic Graph API (Semantic Scholar Academic Graph API, 2024) and, in turn, uses both Open AI’s Chat GPT 3 (ChatGPT, 2024) and Google’s FLAN T5 (FLAN-T5,5, 2024) in various forms to deliver a literature review of the top eight ranked papers. This is complemented by other outputs such as summary extraction, intervention definition and outcomes measured. The processes are mapped in Figure 2.
The research question defined earlier was put into the Elicit engine with the appropriate filters applied. The top eight results were returned on the user interface with additional information. At this point, the number of results was extended to 21 to increase the chances of finding relevant papers that were missed by the PRISMA approach. The resulting data was then exported to a *.csv file for integration with the existing literature review files. This allowed for the removal of duplicates and further investigation.
The 21 results from the Elicit process were then processed through the PRISMA workflow outlined in Figure 1 to determine which of the returned papers should be included in this systematic literature review. Duplicates with the PRISMA systematic literature review results were initially removed (n = 5), followed by the removal of papers that did not pass the abstract and full-text screening (n = 11), leaving five further papers to be analysed.
3. Results and discussion
3.1 Thematic analysis
The year of publication for the resultant articles is shown in the summarised results of Figure 3. There has been a significant increase in the number of results in the last four years (2020–2023); the trend of results shows the beginning of a shift towards the productivity of ML-PBF systems as they mature and are adopted by industry.
The sources of these articles are predominately clustered around journals related to advanced manufacturing, material science and laser processing. This is consistent with what was expected during the development of the research question and the search string. As the research question is centred around particular material types, it was pertinent to take note of the material analysed in each paper. The materials used tended to be steel and titanium alloys, indicating that they are likely the most mature concerning developing a more productive manufacturing process.
Each of the resultant articles reviewed pertained to an increase in productivity, in many cases, the quantification of such was discussed qualitatively with only a few having quantifiable values placed on their increase in productivity. Table 3 summarises the build rate improvement quantified across 14 of the papers that include this information, the majority of which were published within the past three years. In line with the data presented in Figure 3, six of these papers focused on steel alloys, six on Ti6Al4V and two on aluminium alloys. As each of the papers based this improvement in productivity on various sources, across multiple platforms and several materials it is impossible to create a consistent benchmark. With an average increase in productivity of 35%, there is an indication that the work performed on existing ML-PBF machines has yielded an incremental improvement over conventional processing methods. Work that involves the alteration of the machine's laser beam shape (Lantzsch et al., 2022) or power (Huang et al., 2022) has shown build rate improvements of 150% and 450%, respectively.
During the full text and review process, each article was tagged with a theme that best represented or matched the content. Table 4 provides the leading themes, a description and the rate of occurrence across the articles read. The content of some articles led them to be placed in several categories. Thus, the total exceeds the number of articles reviewed.
3.2 Process
Given the ease of access to the parameters used in ML-PBF, a significant body of the literature reviewed exhibited evidence of altering the process to achieve a productivity increase. The process was an identified theme across 24 of the 51 results. This came across in three differing ways; the alteration of existing parameters, the adaption of downstream processing steps and the altering of the production equipment, all of which showed varying degrees of improvement in relation to the productivity of the process and are summarised in Table 5.
The results from the literature review indicate that productivity can be achieved by altering parameters outside of layer thickness to achieve faster build speeds. The majority of work accomplished here is based around a design of experiment (DOE) approach; these typically take on power, speed and hatch spacing as the parameters to alter (Arısoy et al., 2017; Kose et al., 2020; Laakso et al., 2016; Leicht et al., 2020; Liu et al., 2022; Sun et al., 2016; Wang et al., 2019). The majority of approaches used some optimisation model for the parameters, with Response Surface Methodology (RSM) being the most common model used. The model is compared favourably when used as a substitute for others, such as Taguchi and neural network methods (Fotovvati et al., 2020; Khaimovich et al., 2022; Nguyen et al., 2020). The most common approach for understanding the likelihood of success when creating a DOE for new parameters was that of quantifying the amount of energy applied to a given volumetric area that is volumetric energy density (VED), to establish a window where the print is likely to succeed. Although common, it was shown to have inherent flaws as the factors that can affect density and production speed may not be captured using VED. This is illustrated by some bodies of work having identical VEDs of 100 J/mm3, with one having half the build rate of the other (Laakso et al., 2016). As multiple factors are being altered, it is not straightforward to discern the effect of each of the factors on the process. Hatch distance is often used as a factor as an increase has a significant effect on the build rate; this being said, increasing it without compensating the spot size or scanning strategy can lead to an increase in the number and type of defects (Defanti et al., 2022; Makoana et al., 2018).
As components built via ML-PBF typically require heat treatment to alleviate internal stresses or to produce more desirable microstructures, there has been significant published work surrounding the alteration of the process, so that as-built components do not require substantial post-processing (Shipley et al., 2018). The nature of the ML-PBF process presents challenges for achieving repeatability across multiple machines. In effect, there have been several studies investigating the utilisation of more repeatable downstream processes, such as hot isostatic pressing (HIP), to compensate for issues caused by the ML-PBF process (Benzing et al., 2019; Cegan et al., 2020; Du Plessis et al., 2021). For example, if a component is fabricated as a shell of the final form, with metal powder remaining inside the part during the process, and designed to compensate for shrinkage, it has been shown that a HIP process can create fully dense components with similar properties to those fully processed by ML-PBF (Du Plessis et al., 2021). The success of taking this processing approach to improve productivity appears to be more conducive to components that do not have a large variation in cross-sectional area. Fabrication at high speeds allows for more variation and a lack of control in the production system. As this is not desirable when scaling industrial applications, processes such as HIP and vacuum furnace heat treatment can be used to homogenise the components produced, allowing for higher tolerance of porosity when designing parameter sets (Herzog et al., 2020; Kaletsch et al., 2023), an example of such process flow can be seen in Figure 4.
Methods for altering the process are not limited to the process parameters or downstream processes; in some cases, modifications were introduced to existing ML-PBF machines to allow them to process materials more efficiently. Approaches took the form of altering the focal size up to 500 µm to increase the hatch spacing (Antony et al., 2020), changing the laser beam shape to prevent keyholing at high energies and speeds (Grünewald et al., 2021; Lantzsch et al., 2022), as well as the introduction of high-powered lasers to allow the process to maintain at sufficient VED at high speeds (Huang et al., 2022). All these approaches represent an increase in the build rate, but as they require a physical modification of equipment, it limits the ability to scale these approaches to existing ML-PBF machines. Beyond physical manipulation of equipment, one approach is to make use of alternative gases that allow for processing speed increases of up to 37% on certain geometry due to the reduction in soot generated and increased ability for the processing gas to conduct heat away from the melt pool (Pauzon et al., 2020a; Pauzon et al., 2020b).
3.3 Layer
Of the literature reviewed, 17 papers addressed the possibility of increasing the layer thickness beyond the typical values recommended by the manufacturers of the ML-PBF machines, affording the ability to increase productivity. This is an apparent option for improving the productivity of existing ML-PBF machines; however, it comes with significant processing challenges as the materials, laser parameters and physical design of the machines have been designed only for the manufacturer-prescribed typical layer thickness values. In the studies reviewed, varying degrees of success have been reported by utilising higher layer thickness values across the four material groups under examination (steel, titanium, nickel and aluminium), as presented in Figure 3 with the interventions and effects of such summarised in Table 6.
As there are a large number of processing factors at play within any ML-PBF machine (typically over 50), the most common starting point for the development of increased layers is to design a series of experiments that vary the core laser parameters to establish a processing window for the material in question (Calignano, 2014; Pfaff et al., 2020). Within the published literature, there are studies where layer thicknesses up to 200 µm of steel 316 L were tested, with several of these reporting densities of 99.99% (Chen et al., 2022; de Souza et al., 2019; Laohaprapanon et al., 2012; Leicht et al., 2021; Liu et al., 2021; Sinico et al., 2022). Examples of the various parameters and resultant density from this approach can be seen in Figure 5. Given the increased thickness of successive layers, the penetration depth and melt pool dynamics have to be accounted for when developing the parameters (Hassine et al., 2021). As such, VED is often maintained or increased; thus, the scanning speed of parameters is often reduced as the power of existing ML-PBF machines can be a limiting factor to maintaining sufficient VED. Therefore, the reported processing speed for increased layer thickness for steel 316 L can be relatively low. The upper bound reported for 200 µm layers was a build rate of 12.4 mm3/s (Chen et al., 2022). Pending any other issues arising from fabricating parts at thicker layers, this speed demonstrates a tenfold improvement over typical build rates of steel 316 L on 400 W ML-PBF machines. The most common approach for designing the experiments here was to use VED as the indicator for an acceptable processing window whilst using DOE tools to discern the values for the parameters being altered. In some cases, the resulting mechanical properties of the materials were reported to surpass the requirements of ASTM A240M-18 (ASTM International, 2019; Leicht et al., 2021).
This direct parameter optimisation approach appears to be dominant for steel-based powders but was also shown to be applicable and adopted for Ti6Al4V, Inconel 718 and aluminium alloys, with an increase in productivity to be demonstrated across all of them (Del Guercio and Simonelli, 2023; Jing et al., 2021; Shoukr et al., 2023).
Beyond the method of altering the dominant laser parameters to achieve density at higher layer thicknesses outlined above, another approach to achieve higher productivity with altered layer heights is to adopt a technique known as “shell and core” or “hull and bulk”. This involves using different parameters for the interior of a part and the outer contours, as shown in Figure 6. By employing this technique, one can use differing layer heights for each parameter set, thus leaving the interior to be melted at intermittent layers. This can lead to higher build rates, whilst maintaining a reasonable surface on the outer faces. The process is often denoted by Shell(n0,n + 1) & Core(n0,n + 2) or (n0,n + 3), where n is the layer number, depending on the differential between the layer heights. Using this technique, Tool Steel H13 was produced at 99.97% density using 100 µm layer heights on a dual laser specialised setup from SLM solutions (Yonehara et al., 2020). This type of ML-PBF machine has been developed for the express purpose of shell and core strategies. The resultant improvement in processing speed over a typical 400 W power setup was found to be 30%. The limiting factor here was of lower scanning speed required to maintain sufficient energy density (Yonehara et al., 2020).
For Ti6Al4V, the “shell and core” strategy was used across layer thickness between 30 µm and 150 µm. All studies reviewed indicated that as a result of using this approach, the productivity could be improved over the baseline for the respective equipment without significant impact on the mechanical properties and dimensional accuracy of the produced components when the typical post-processing and heat treatment processes were followed (de Formanoir et al., 2020; Kan et al., 2022; Wang et al., 2023). The nature of this technique means that its effect on productivity is dependent on the surface-to-volume ratio of the components being produced. One of the studies indicated that for structures with a volume-to-surface ratio of less than 5 cm−1 an increase in productivity of 25% may be achieved, whilst it was not recommended for structures with a volume-to-surface ratio over 10 cm−1. Examples of such parts are lattice and gyroid structures (de Formanoir et al., 2020). It was noted that due to the experimental design of the studies, the interlayer time of specimens would not be representative of how they will be produced, potentially resulting in false indicators of the mechanical and dimensional properties of the samples tested. The effect of multiple layer thicknesses on a single part was noted as having a reducing effect on the elongation of Ti6Al4V (Gullane et al., 2021) suggesting that there may be a need for further investigation for the feasibility of this method.
Due to the complex nature of the ML-PBF process, the literature findings have indicated that it can be experimentally intensive to develop parameters for increased layer thicknesses. To overcome this, some experiments explored the development of models that would obtain the experimental data from simple single-line tracks to create simulations accounting for various factors and ultimately decrease the time taken to develop high-layer thickness parameter sets (Hassine et al., 2021; Ten et al., 2020). Models also offer some insight into the effect of parameters, such as laser focus, which are typically not altered, and may allow for processing at higher layer thicknesses without increasing the creation of inherent defects.
3.4 Surface
Two major drivers of the cost of parts produced by ML-PBF are the removal of supporting materials and the reduction of surface roughness (Baumers et al., 2016). Of the 51 papers reviewed, 11 are examining these aspects. These articles were marked with the surface theme falling into two major subcategories; characterisation of surface roughness improvements as a result of parameter development and the development of parameters that would allow for the reduction of supports required on down skins. The first subcategory showed that by taking both unstructured (Dong et al., 2021a) and structured experimental approaches (Deng et al., 2020; Fotovvati et al., 2020) modification of the process parameters from the standard approach can lead to an improvement in the surface roughness without compromising the mechanical properties or density (Tang et al., 2020; Wang et al., 2019). Some of the approaches taken here to reduce the experimental load and determine the optimum parameters were that of Taguchi and central composite design (CCD) (Deng et al., 2020; Fotovvati et al., 2020). The studies which utilised the process were successful in identifying the parameters that would affect the level of roughness as a result of altering parameters. In some cases, they were able to differentiate and optimise for roughness across the side, up and down faces, the level to which they affect various part properties can be seen in Table 7 (Fotovvati et al., 2020).
The studies that focused on improving the down faces through support removal or parameter changes were successful in that. By modifying laser power, speed and several down-facing layers, overhangs of 30° can be fabricated with densities over 99% (Mertens et al., 2014); doing so demonstrated that the application of normal energy densities to aluminium can create a warping effect in the material as a result of the reduction of material beneath that can act as a heat sink. This has also been shown as an effective approach for allowing for increased overhang angles in Ti6Al4V parts (Tang et al., 2020; Wang et al., 2013). In some cases, supporting structures cannot be avoided, such as a material’s propensity for residual stress build-up or to avoid the creation of undesirable microstructures due to the cooling profile(Wang et al., 2019). To accommodate this whilst allowing for the ease of removal of supports, the contact area between the components and supports can be modified in both geometry and density (Calignano, 2014).
Except for the general laser parameters provided in Table 7 (i.e. laser power, hatch spacing, scan speed, layer thickness and stripe width), altering other ML-PBF machine operational aspects may also lead to an improvement in the surface roughness of the produced components. In particular, past research studies have found that increasing the number of edge contours can reduce the surface roughness by 80%, to a value of 4.3 µm in standard Ti6Al4V powder, 15-45µm particle sizing with a D50 of 30 µm (Shi et al., 2022; Wan et al., 2020). Within the capabilities of ML-PBF machines, one can also employ secondary scanning of surface faces to allow for the re-melting of the material to reduce the surface roughness (Alrbaey et al., 2014). Employing this approach will lead to increased production times as the laser will be performing multiple passes of certain areas; as such, it needs to be taken into account when using this approach over conventional surface treatment processes.
Although difficult to quantify, it is clear that the improvement in surface roughness and reduction of supporting structures demonstrated in the literature review will mostly have a positive effect on the reduction of cost per component produced and ultimately will allow for further adoption of this technology. The interventions and effects have been summarised in Table 8.
3.5 Scan
The laser scan path, which fills in the hatch area of a part, can have an impact on the time required to produce the part. Typical hatch patterns used in ML-PBF are that of meander, stripe and checkerboard. As each of these patterns leads to varying interlayer time, number of hatch borders and internal stresses, the mechanical properties, such as ultimate tensile strength and hardness, can vary depending on the pattern used (Shi et al., 2022; Slodczyk et al., 2021).
For thin wall features, commonly seen in ML-PBF, lattices and gyroids, which have cross-sectional areas above the contouring distance, the laser scan path can lead to long production times as the number of jumps between when the laser is active is high with respect to the cross-sectional area. To avoid this, one can produce thin walls with single or multiple contour passes allowing the laser to scan an entire island without the laser turned off. In effect, this can lead not only to an increased build rate but possibly to a reduction in surface roughness (Cao et al., 2022; Wan et al., 2020).
Another scan strategy that can be adopted is that of using multiple layer heights within a part to reduce the time consumed on bulk areas which do not possess complex features. This approach is usually coupled with the “shell and core” strategy discussed in Section 3.3. It applies to the scan theme as it involves altering the scan pattern, thus creating a new zone between the contour and bulk hatching that can have differing mechanical properties (de Formanoir et al., 2020; Gullane et al., 2021; Kan et al., 2022; Shi et al., 2022; Yonehara et al., 2020). The interventions and effects have been summarised in Table 9.
3.6 Artificial neural networks
A trend seen across the literature reviewed is that of the application of increasingly sophisticated machine learning models to develop parameter sets. Given the goal is to optimise a process and that there are significant volumes of data available, the ML-PBF process is quite conducive to the use of tools such as artificial neural networks (ANNs). In some cases, ANNs were shown to be capable of using over 2048 data points(Nguyen et al., 2020). The resulting parameters were able to achieve 99.98% part density. Although the source of the training data is not disclosed by the authors, the achieved density value offers an indication of the ability of ANNs to reduce physical experimentation in the pursuit of optimal parameters. The efficacy of using ANNs for this process was determined by comparing its output to the results obtained via the Taguchi method and the RSM for the optimisation of Ti6Al4V parameters. In particular, it was found that the Taguchi method was the least accurate, with ANNs being the most accurate, across every mechanical performance predictor except microhardness, where the RSM was more accurate (Fotovvati et al., 2020). Given that the ANN models are trained on bodies of data, the accuracy of such a model will be dependent on the quality of the data given. As ML-PBF is a complex process, where repeatability across machines is challenging to achieve, ANNs may be limited in use for the final optimisation of a production process but may have an important role in the development of parameters beyond those currently used (Chen et al., 2022). The interventions and effects have been summarised in Table 10.
The papers identified by the SLR process discussed here represent a larger movement in the optimisation of ML-PBF through the use of ANNs (Goh et al., 2021). Work done in this area have focused on the optimisation of the material and mechanical performance of the outputted components but do illustrate that through the opportunities to derive more complex multi objective optimisation studies whilst reducing the cost for such. If fully realised in manufacturing environments, ANNs represent an opportunity to optimise not for just a machine or material, but for every build, leading to a more robust and repeatable manufacturing method.
3.7 Materials
Most materials processed by ML-PBF have been used for decades in mainstream (conventional) manufacturing methods. Since ML-PBF is a full melt powder-based process that induces heat using a laser, many material-specific mechanical and metallurgical issues can prevent ML-PBF from improving productivity. As most industries are hesitant to change the alloys they use, other methods of changing materials have been identified in the reviewed literature. One method in question is the introduction of nanoparticles to coat the base material. The premise is that by increasing the absorptivity of the material, the energy required to melt it will be reduced. Pannitz (2021) applied three nanocoatings to steel 1.2709 powder, silicon carbide (SIC), few-layer graphene (FLG) and iron oxide black (IOB), to increase the energy absorption rate of the material, SEM images of such are shown in Figure 7. It was also found that the increased roughness, as a result of the addition of the coating, creates beam traps which in turn resulted in less energy being reflected away. The materials processed as part of this study were capable of reaching a 99.99% density, with the coated materials achieving higher build rates (Pannitz et al., 2021). A similar technique was used on aluminium with TiC nanocoatings, which led to a laser efficiency increase of up to 512%, with the use of optimised parameters (Qu et al., 2022).
Currently, ML-PBF uses powder spherical particles which are subject to strict quality control standards and are produced using high-cost atomisation processes (Wang et al., 2015b; Wang et al., 2015a). Through the use of ball milling, in situ alloying and parameters optimisation, lower-grade powder can be processed leading to a cost reduction of over 85% for materials such as Ti6Al4V (Dong et al., 2021b). Although not a direct efficiency gain, the reduction in process cost may offset part of the overall component cost on current-generation ML-PBF machines.
Both approaches demonstrate opportunities for novel materials to improve ML-PBF productivity whilst also showing that the addition of such can affect the final part chemistry, thus possibly creating a new barrier to entry as a result of inertia towards the adoption of new material grades, the interventions and effects have been summarised in Table 11.
3.7 Efficacy of the elicit process
Using only the research question, the Elicit process was capable of contributing to the systematic literature review by adding 10.2% of the papers used in the final analysis. Of the 21 returned results put through the PRISMA framework, 55% passed. These breakdown as five new review papers and five duplicates of the 46 that had passed through the manual search. This demonstrates a very high affinity for the Elicit process to interpret the research question in a manner similar to that done by the researcher. Given the Elicit process used a single source (Semantic Scholar) and the manual systematic literature review used three (WEEE, WoS and SCOPUS), a divergence in the results of both searches is to be expected. Some results returned did semantically differ from the expected as lower results ranked lacked relevance. This is likely caused by not only the interpretation of the question being asked but also the result of the database being used and the requirement to return 21 results regardless of how well they answer the questions. As such, it is imperative that even with an AI-driven tool like this, the results are subsequently run through an agreed PRIMSA framework.
The area where the Elicit system worked and can later serve systematic literature reviews better is in its ability to summarise abstracts, extract interventions and results and give interpretations of how the top papers can answer the research question being asked. The ability to quickly see summaries of abstracts and interventions can expedite the manual review process used within the PRISMA framework. The top-ranked papers were summarised by the Elicit tool as the following:
The papers collectively suggest that optimizing the productivity of laser powder bed fusion machines for industry-specific metal alloys can overcome barriers to adoption. Herzog et al. (2020) proposes a combined approach of Laser Powder Bed Fusion and subsequent Hot Isostatic Pressing as a method to improve productivity. Defanti et al. (2022) pursues the combined optimization of part quality and process productivity for AlSi10Mg by going beyond the commonly used approach based solely on volumetric energy density. Lantzsch et al. (2021) presents a flexible dual-fibre laser array for LPBF processing, which allows for more dynamic laser beam movement across the powder bed. Vicario 2021 discusses a long-term European project aiming at the development of new optimized powders and LPB/SLM process parameters to maximize productivity, control roundness and roughness of cooling channels, maximize powder life, reduce the tendency to cracking, and increase thermal fatigue properties. Overall, these papers suggest that optimizing the productivity of laser powder bed fusion machines for industry-specific metal alloys can lead to increased productivity and cost-effectiveness, making the technology more attractive to cost-sensitive industries. (Literature review summary of top 4 papers from Elicit, Elicit.org)
4. Conclusions
The data extracted and analysed from the systematic literature review has given credence to the research question and broad applicability of such whilst also reinforcing the timing of it with regard to current research trends and the prominent materials being used in ML-PBF.
The thematic categories that emerged help in answering and developing solutions to the research question; improved productivity of current generation ML-PBF machines processing industrially relevant materials. Significant points of note are as follows:
Alternating scanning approaches can lead to an increase in productivity of up to 252% in situations where the geometry is suitable.
Using approaches such as a RSM DOE to develop higher thickness processing parameters can lead to productivity increases of 65% with a relatively negligible impact on mechanical performance, according to published literature.
Where data was available, it was found that applying the described productivity-increasing methods typically leads to poorer mechanical properties.
Although the SLR method appears to have generated sufficient relative literature to address the research question, the terminology used may exclude relevant work. As such, it should be noted that the efficacy of this method will depend on closely on the KW used and the numbers of participants engage in the methodology.
The concurrent generation of a systematic literature review through AI tools like Elicit can contribute to the traditional PRIMSA approach by interpreting the research question without the bias of a research team, as shown by the acceptable additional content discovered by the Elicit search.
Figures
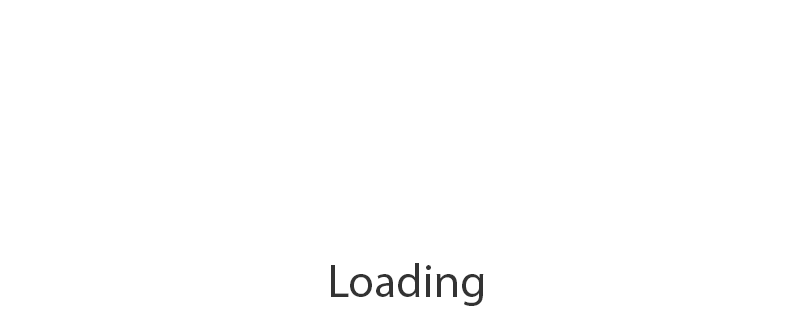
Figure 2
Elicit literature review process garnered from information on their website (Elicit, 2023)
Search string terms and filters used for the systematic literature review database search
Database | Scopus, web of science, IEEE |
---|---|
Search date | 17th July 2023 |
Keywords | KW1: “additive manufacturing” OR “metal additive manufacturing” OR “powder bed fusion” OR “laser based additive manufacturing” OR “selective laser melting” OR “SLM” OR “PBF” OR “3d printing" |
KW2: “titanium” OR “ti” OR “steel” OR “aluminium” OR “nickel" | |
KW3: “optimisation” OR “optimization” OR “efficiency” OR “productivity” OR “production" | |
KW4: “weld” OR “wire-arc” OR “DED” OR “wire” OR “cold spray” OR “electron” OR “deposition” Or “binder" | |
Boolean operators | "KW1” AND “KW2” AND “KW3” NOT “KW4" |
Fields | Title, abstract, authors keywords |
Filters | English |
Source: Table by authors
Literature review tools investigated
Tool | Capabilities | Database | Link |
---|---|---|---|
Elicit | Paper summary, reference extraction | Semantic scholar | www.elicit.org (Elicit, 2024) |
Silvi | PDF capture, document screening, PRIMSA creation | Pubmed, ClinicalTrials.gov | www.silvi.ai (Silvi.ai, 2024) |
Iris | Table extraction, paper summary, reference extraction, automated search | N/A | www.iris.ai (Iris.ai, 2024) |
Scholarcy | Table extraction, paper summary, reference extraction | N/A | www.scholarcy.com (Scholarcy, 2024) |
Rayyan | PRIMSA creation, Mendeley import | N/A | www.rayyan.ai (Rayyan, 2024) |
Enago | Paper summary, reference extraction | N/A | www.read.enago.com(Enago Read, 2024) |
Source: Table by authors
List of systematic literature review results that quantify the build rate increase as a result of the experimental interventions. Values reported by the studies are relative to the baseline values used by the studies' investigators as such are not interrelated
Author | Title | Year | Material | Build rate increase (%) |
---|---|---|---|---|
(Sun et al., 2016) | Selective laser melting of stainless steel 316 L with low porosity and high build rates | 2016 | Steel | 71 |
(de Souza et al., 2019) | Effect of laser speed, layer thickness and part position on the mechanical properties of maraging 300 parts manufactured by selective laser melting | 2019 | Steel | 7–40 |
(Herzog et al., 2020) | Productivity optimisation of laser powder bed fusion by hot isostatic pressing | 2020 | Titanium | 24.5 |
(Yonehara et al., 2020) | Parameter optimisation of the high-power laser powder bed fusion process for H13 tool steel | 2020 | Steel | 30 |
Pauzon et al., 2020b) | Effect of the process gas and scan speed on the properties and productivity of thin 316 L structures produced by laser-powder bed fusion | 2020 | Steel | 37 |
(Pauzon et al., 2020a) | Argon-helium mixtures as laser-powder bed fusion atmospheres: towards increased build rate of Ti-6Al-4V | 2020 | Titanium | 40 |
(de Formanoir et al., 2020) | Increasing the productivity of laser powder bed fusion: Influence of the hull-bulk strategy on part quality, microstructure and mechanical performance of Ti-6Al-4V | 2020 | Titanium | 25 |
(Du Plessis et al., 2021) | Productivity enhancement of laser powder bed fusion using compensated shelled geometries and hot isostatic pressing | 2021 | Titanium | 12 |
(Gullane et al., 2021) | On the use of multiple layer thicknesses within laser powder bed fusion and the effect on mechanical properties | 2021 | Titanium | 22 |
(Liu et al., 2021) | Study on performance optimisation of 316 L stainless steel parts by High-Efficiency Selective Laser Melting | 2021 | Steel | 3 |
(Defanti et al., 2022) | Boosting Productivity of Laser Powder Bed Fusion for AlSi10Mg | 2022 | Aluminium | 25 |
(Sinico et al., 2022) | High-speed laser powder bed fusion of M789 tool steel with an optimized 120 µm layer thickness approach | 2022 | Steel | 91 |
(Wang et al., 2023) | Study on the organisation and properties of Ti-6Al-4V fabricated by laser powder bed fusion based on the thickness of the gradient layer | 2023 | Titanium | 25 |
(Del Guercio and Simonelli, 2023) | Increasing the build rate of high-strength aluminium alloys produced by laser powder bed fusion | 2023 | Aluminium | 50 |
Source: Table by authors
Emergent themes following the analysis of articles resulting from the PRISMA process
Theme | Description | No. of articles |
---|---|---|
Process | Infers processing parameters were investigated to improve typical process porosity/microstructures | 24 |
Layer | Infers layer height changes were used to achieve higher productivity | 17 |
Scan | Infers’ alternative scanning strategy is being investigated | 11 |
Surface | Infers work done on the improvement of surfaces through scan strategies or parameter developments | 11 |
Material | Infers material changes to improve processing | 5 |
Artificial neural networks (ANN) | Infers the use of artificial neural networks (ANN) and machine learning to predict and optimize process parameters | 3 |
Source: Table by authors
Interventions and effects of such as a result of altering the process to increase productivity
Intervention | Effect |
---|---|
Alteration of printing parameters using the volumetric energy density (VED) as a guiding principle | Higher processing speeds were achieved |
Application of hot isostatic pressing to components produced outside of the optimised processing window | Reduction in porosity levels Geometric shrinkage of components Nominal mechanical properties maintained Expansion of processing window |
Modification of laser power or beam profile | Reduction of keyholing at high powers and speeds Expansion of processing window |
Introduction of alternative gas mixtures during the production process | Reduction of soot generation during the melting process Reduction in residual stress Expansion of processing window |
Source: Table by authors
Interventions and effects of such as a result of altering the layer thickness to increase productivity
Intervention | Effect |
---|---|
Increasing layer height beyond typical values | A general increase in porosity Reduction of mechanical properties Increased processing speed Increased surface roughness |
Application of shell and core strategies to allow for surface integrity whilst using increased layer heights | Generation of multiple grain structures within components Reduction of mechanical properties Maintenance of surface roughness values Increased processing speed |
Source: Table by authors
p-Values and contribution percentage of the process parameters in different responses based on ANOVA results
Parameter | Microhardness | Relative density | Top surface roughness | |||
---|---|---|---|---|---|---|
p-value | Contribution (%) | p-value | Contribution (%) | p-value | Contribution (%) | |
A (laser power) | 0.071 | 45.3 | 0.103 | 22.6 | 0.004 | 19 |
B (scan speed) | 0.185 | 23.3 | 0.197 | 14.1 | 0.002 | 27.7 |
C (hatch spacing) | 0.334 | 13.9 | 0.098 | 23.5 | 0.001 | 33.6 |
D (layer thickness) | 0.542 | 7.9 | 0.075 | 28 | 0.004 | 17.6 |
E (stripe width) | 0.984 | 0.7 | 0.464 | 6.2 | 0.282 | 1.3 |
Error | – | 8.8 | – | 5.6 | – | 0.7 |
Upskin surface | Upskin horizontal line | Upskin vertical line | ||||
p-value | Contribution (%) | p-value | Contribution (%) | p-value | Contribution (%) | |
A (laser power) | 0.304 | 11.4 | 0.192 | 22.6 | 0.035 | 20.4 |
B (scan speed) | 0.196 | 46.6 | 0.15 | 27.3 | 0.02 | 28.4 |
C (hatch spacing) | 0.114 | 24.8 | 0.131 | 30 | 0.034 | 20.8 |
D (layer thickness) | 0.065 | 35.9 | 0.5 | 8.8 | 0.029 | 22.8 |
E (stripe width) | 0.624 | 4.7 | 0.878 | 2.5 | 0.258 | 5.2 |
Error | 6.6 | 8.8 | 2.6 | |||
Downskin surface | Downskin horizontal line | Downskin vertical line | ||||
p-value | Contribution (%) | p-value | Contribution (%) | p-value | Contribution (%) | |
A (laser power) | 0.646 | 5.6 | 0.956 | 2.9 | 0.694 | 7.6 |
B (scan speed) | 0.996 | 0.3 | 0.99 | 1.2 | 0.879 | 3.6 |
C (hatch spacing) | 0.772 | 3.7 | 0.972 | 2.2 | 0.705 | 7.3 |
D (layer thickness) | 0.024 | 81.2 | 0.141 | 64.7 | 0.071 | 66.9 |
E (stripe width) | 0.974 | 0.9 | 0.769 | 9 | 0.966 | 1.6 |
Error | – | 8.3 | – | 20 | – | 13 |
Source: Table courtesy of Fotovvati et al., 2020
Interventions and effects of such as a result optimising the parameter sets for surface roughness
Intervention | Effect |
---|---|
Development of alternative parameter sets using various statistical techniques | Reduction of supports required to build overhung components Increased support free angle of 30o Reduction in downstream post-processing |
Increased number of contour passes | Reduction of surface roughness by 80% Reduction in downstream post-processing |
Application of re-melting laser pass | Reduction of surface roughness Reduction in downstream post-processing |
Source: Table by authors
Interventions and effects of such as a result of altering scan strategy to increase productivity
Intervention | Effect |
---|---|
Reduction or elimination of hatch scanning on thin wall features by increasing the number of contour passes or geometric alterations | Reduction in the laser scanner movement and downtime Higher processing speeds were achieved |
Altering of scanning pattern for bulk material | Reduction of residual stress with certain scanning patterns Higher processing speeds made possible |
Source: Table by authors
Interventions and effects of such as a result of the use of artificial neural networks to increase productivity
Intervention | Effect |
---|---|
Application of neural networks to determine the optimum processing window | Increased mechanical performance Higher processing speeds were achieved |
Source: Table by authors
Interventions and effects of altering the processed material to improve productivity
Intervention | Effect |
---|---|
Application of secondary materials to standard AM powders | Increased laser efficiency recorded Higher processing speeds were achieved |
Processing of sub-optimal ball-milled powders | Creation of fully dense components Cost reduction of 85% |
Source: Table by authors
References
Alrbaey, K., Wimpenny, D., Tosi, R., Manning, W. and Moroz, A. (2014), “On optimization of surface roughness of selective laser melted stainless steel parts: a statistical study”, Journal of Materials Engineering and Performance, Vol. 23 No. 6, pp. 2139-2148, doi: 10.1007/s11665-014-0993-9.
Antony, K., Clint, C. and Rakeshnath, R. (2020), “Study of porosity and build rate of the selective laser melting (SLM) of titanium and its statistical modelling for optimization”, Lasers in Eng, Vol. 47, pp. 95-111, available at: https://web-s-ebscohost-com.proxy.lib.ul.ie/ehost/pdfviewer/pdfviewer?vid=0&sid=e07053dc-9c49-44b8-90c8-c44cfd0bbc59%40redis
Arısoy, Y.M., Criales, L.E., Özel, T., Lane, B., Moylan, S. and Donmez, A. (2017), “Influence of scan strategy and process parameters on microstructure and its optimization in additively manufactured nickel alloy 625 via laser powder bed fusion”, The International Journal of Advanced Manufacturing Technology, Vol. 90 No. 5-8, pp. 1393-1417, doi: 10.1007/s00170-016-9429-z.
ASTM International (2019), “ASTM A240/A240M-18 - Standard specification for chromium and chromium-nickel stainless steel plate, sheet, and strip for pressure vessels and for general applications”, available at: https://webstore.ansi.org/standards/astm/astma240a240m18
Attaran, M. (2017), “The rise of 3-D printing: the advantages of additive manufacturing over traditional manufacturing”, Business Horizons, Vol. 60 No. 5, pp. 677-688, doi: 10.1016/j.bushor.2017.05.011.
Atzeni, E. and Salmi, A. (2012), “Economics of additive manufacturing for end-usable metal parts”, The International Journal of Advanced Manufacturing Technology, Vol. 62 No. 9-12, pp. 1147-1155, doi: 10.1007/s00170-011-3878-1.
Baumers, M., Dickens, P., Tuck, C. and Hague, R. (2016), “The cost of additive manufacturing: machine productivity, economies of scale and technology-push”, Technological Forecasting and Social Change, Vol. 102, pp. 193-201, doi: 10.1016/j.techfore.2015.02.015.
Benzing, J., Hrabe, N., Quinn, T., White, R., Rentz, R. and Ahlfors, M. (2019), “Hot isostatic pressing (HIP) to achieve isotropic microstructure and retain as-built strength in an additive manufacturing titanium alloy (Ti-6Al-4V)”, Materials Letters, Vol. 257, doi: 10.1016/j.matlet.2019.126690.
Buranich, V., Rogoz, V., Postolnyi, B. and Pogrebnjak, A. (2020), “Predicting the properties of the refractory high-entropy alloys for additive manufacturing-based fabrication and mechatronic applications”, 2020 IEEE 10th International Conference Nanomaterials: Applications & Properties (NAP), 1–5, doi: 10.1109/NAP51477.2020.9309720.
Calignano, F. (2014), “Design optimization of supports for overhanging structures in aluminum and titanium alloys by selective laser melting”, Materials & Design, Vol. 64, pp. 203-213, doi: 10.1016/j.matdes.2014.07.043.
Cao, X., Carter, L.N., Villapún, V.M., Cantaboni, F., de Sio, G., Lowther, M., Louth, S.E.T., Grover, L., Ginestra, P. and Cox, S.C. (2022), “Optimisation of single contour strategy in selective laser melting of Ti-6Al-4V lattices”, Rapid Prototyping Journal, Vol. 28 No. 5, doi: 10.1108/RPJ-04-2021-0103.
Cegan, T., Pagac, M., Jurica, J., Skotnicova, K., Hajnys, J., Horsak, L., Soucek, K. and Krpec, P. (2020), “Effect of hot isostatic pressing on porosity and mechanical properties of 316 l stainless steel prepared by the selective laser melting method”, Materials, Vol. 13 No. 19, pp. 1-26, doi: 10.3390/ma13194377.
ChatGPT (2024), available at: https://chat.openai.com/auth/login (accessed 12 September 2023).
Chekotu, J.C., Groarke, R., O’Toole, K. and Brabazon, D. (2019), “Advances in selective laser melting of nitinol shape memory alloy part production”, Materials, Vol. 12 No. 5, doi: 10.3390/MA12050809.
Chen, D., Wang, P., Pan, R., Zha, C., Fan, J., Li, S. and Cheng, K. (2022), “Process optimization of selective laser melting 316L stainless steel by a data-driven nonlinear system”, Welding in the World, Vol. 66 No. 3, pp. 409-422, doi: 10.1007/s40194-021-01205-0.
de Formanoir, C., Paggi, U., Colebrants, T., Thijs, L., Li, G., Vanmeensel, K. and Van Hooreweder, B. (2020), “Increasing the productivity of laser powder bed fusion: influence of the hull-bulk strategy on part quality, microstructure and mechanical performance of Ti-6Al-4V”, Additive Manufacturing, Vol. 33, p. 101129, doi: 10.1016/j.addma.2020.101129.
de Souza, A.F., Al-Rubaie, K.S., Marques, S., Zluhan, B. and Santos, E.C. (2019), “Effect of laser speed, layer thickness, and part position on the mechanical properties of maraging 300 parts manufactured by selective laser melting”, Materials Science and Engineering: A, Vol. 767, doi: 10.1016/j.msea.2019.138425.
Defanti, S., Cappelletti, C., Gatto, A., Tognoli, E. and Fabbri, F. (2022), “Boosting productivity of laser powder bed fusion for AlSi10Mg”, Journal of Manufacturing and Materials Processing, Vol. 6 No. 5, doi: 10.3390/jmmp6050112.
Del Guercio, G. and Simonelli, M. (2023), “Increasing the build rate of high-strength aluminium alloys produced by laser powder bed fusion”, Optics & Laser Technology, Vol. 161, p. 109133, doi: 10.1016/j.optlastec.2023.109133.
Deng, Y., Mao, Z., Yang, N., Niu, X. and Lu, X. (2020), “Collaborative optimization of density and surface roughness of 316L stainless steel in selective laser melting”, Materials, Vol. 13 No. 7, p. 1601, doi: 10.3390/ma13071601.
Di, L. and Yang, Y. (2021), “Cost modeling and evaluation of direct metal laser sintering with integrated dynamic process planning”, Sustainability, Vol. 13 No. 1, pp. 1-17, doi: 10.3390/su13010319.
Dong, Y.P., Li, Y.L., Zhou, S.Y., Zhou, Y.H., Dargusch, M.S., Peng, H.X. and Yan, M. (2021a), “Cost-affordable Ti-6Al-4V for additive manufacturing: powder modification, compositional modulation and laser in-situ alloying”, Additive Manufacturing, Vol. 37, p. 101699, doi: 10.1016/j.addma.2020.101699.
Dong, D., Chang, C., Wang, H., Yan, X., Ma, W., Liu, M., Deng, S., Gardan, J., Bolot, R. and Liao, H. (2021b), “Selective laser melting (SLM) of CX stainless steel: theoretical calculation, process optimization and strengthening mechanism”, Journal of Materials Science & Technology, Vol. 73, pp. 151-164, doi: 10.1016/j.jmst.2020.09.031.
Du Plessis, A. and Macdonald, E. (2020), “Hot isostatic pressing in metal additive manufacturing: x-ray tomography reveals details of pore closure”, Additive Manufacturing, Vol. 34, p. 101191, doi: 10.1016/J.ADDMA.2020.101191.
Du Plessis, A., Yelamanchi, B., Fischer, C., Miller, J., Beamer, C., Rogers, K., Cortes, P., Els, J. and MacDonald, E. (2021), “Productivity enhancement of laser powder bed fusion using compensated shelled geometries and hot isostatic pressing”, Advances in Industrial and Manufacturing Engineering, Vol. 2, p. 100031, doi: 10.1016/j.aime.2021.100031.
Elicit (2024), available at: https://elicit.org (accessed 12 September 2023).
Elicit (2023), The AI Research Assistant, available at: https://elicit.com (accessed 12 September 2023).
Enago Read (2024), available at: www.read.enago.com/ (accessed 12 September 2023).
FLAN-T5 (2024), available at: https://huggingface.co/docs/transformers/model_doc/flan-t5 (accessed 12 September 2023).
Fotovvati, B., Balasubramanian, M. and Asadi, E. (2020), “Modeling and optimization approaches of laser-based powder-bed fusion process for ti-6al-4v alloy”, Coatings, Vol. 10 No. 11, pp. 1-29, doi: 10.3390/coatings10111104.
Gibson, I., Rosen, D.W. and Stucker, B. (2010), “Additive manufacturing technologies: Rapid prototyping to direct digital manufacturing”, Additive Manufacturing Technologies: Rapid Prototyping to Direct Digital Manufacturing, Springer, New York, NY, available at: https://link.springer.com/book/10.1007/978-1-4419-1120-9
Goh, G.D., Sing, S.L. and Yeong, W.Y. (2021), “A review on machine learning in 3D printing: applications, potential, and challenges”, Artificial Intelligence Review, Vol. 54 No. 1, pp. 63-94, doi: 10.1007/s10462-020-09876-9.
Gordon, E.R., Shokrani, A., Flynn, J.M., Goguelin, S., Barclay, J. and Dhokia, V. (2016), “A surface modification decision tree to influence design in additive manufacturing”, Smart Innovation, Systems and Technologies, Springer, Cham, 52, pp. 423-434, available at: https://link.springer.com/chapter/10.1007/978-3-319-32098-4_36
Grünewald, J., Gehringer, F., Schmöller, M. and Wudy, K. (2021), “Influence of ring-shaped beam profiles on process stability and productivity in laser-based powder bed fusion of AISI 316L”, Metals, Vol. 11 No. 12, doi: 10.3390/met11121989.
Gullane, A., Murray, J.W., Hyde, C.J., Sankare, S., Evirgen, A. and Clare, A.T. (2021), “On the use of multiple layer thicknesses within laser powder bed fusion and the effect on mechanical properties”, Materials & Design, Vol. 212, doi: 10.1016/j.matdes.2021.110256.
Harkin, R., Quinn, J. and McFadden, S. (2018), “A review of powder bed fusion for additively manufactured Ti-6wt.%Al-4wt.%V”, Imc35, 2-6.
Hassine, N., Chatti, S. and Slama, M.B. (2021), “High layer thickness influence on melt Pool sizes and defects appearing of Ti6Al4V at high scan speeds”, HORA 2021 - 3rd International Congress on Human-Computer Interaction, Optimization and Robotic Applications, Proceedings, doi: 10.1109/HORA52670.2021.9461334.
Herzog, D., Bartsch, K. and Bossen, B. (2020), “Productivity optimization of laser powder bed fusion by hot isostatic pressing”, Additive Manufacturing, Vol. 36, doi: 10.1016/j.addma.2020.101494.
Huang, G., Wei, K., Deng, J. and Zeng, X. (2022), “High power laser powder bed fusion of 18Ni300 maraging steel: processing optimization, microstructure and mechanical properties”, Materials Science and Engineering: A, Vol. 856, doi: 10.1016/j.msea.2022.143983.
IEEE Xplore (2024), available at: https://ieeexplore.ieee.org/Xplore/home.jsp (accessed 12 September 2023).
Iris.ai (2024), available at: https://iris.ai/ (accessed 12 September 2023).
ISO/TC 261 (2021), “ISO/ASTM 52900:2021(en) additive manufacturing—general principles—fundamentals and vocabulary”, In ISO/ASTM, available at: www.iso.org/standard/74514.html
Jing, Y., Wang, P. and Yan, X. (2021), “Effect of process parameters and layer thickness on the quality and performance of Ti-6Al-4V fabricated by selective laser melting”, Coatings, Vol. 11 No. 11, doi: 10.3390/coatings11111323.
Kaletsch, A., Sondermann, M., Mirz, M., Radtke, F. and Broeckmann, C. (2023), “Influence of PBF-LB process atmosphere on the fatigue strength of hot isostatically Post-Densified duplex steel parts produced via the shell core approach”, Materials, Vol. 16 No. 11, doi: 10.3390/ma16114014.
Kan, W.H., Gao, M., Zhang, X., Liang, E., Chiu, N.S.L., Lim, C.V.S. and Huang, A. (2022), “The influence of porosity on Ti-6Al-4V parts fabricated by laser powder bed fusion in the pursuit of process efficiency”, The International Journal of Advanced Manufacturing Technology, Vol. 119 No. 7-8, pp. 5417-5438, doi: 10.1007/s00170-021-08374-8.
Khaimovich, A., Balyakin, A., Oleynik, M., Meshkov, A. and Smelov, V. (2022), “Optimization of process parameters for powder bed fusion additive manufacturing using a linear programming method: a conceptual framework”, Metals, Vol. 12 No. 11, doi: 10.3390/met12111976.
Korpela, M., Riikonen, N., Piili, H., Salminen, A. and Nyrhilä, O. (2020), “Additive manufacturing—past, present, and the future”, Technical, Economic and Societal Effects of Manufacturing 4.0, Palgrave Macmillan, Cham, pp. 17–41, available at: https://link.springer.com/chapter/10.1007/978-3-030-46103-4_2
Kose, H., Jin, M. and Peng, T. (2020), “Quality and productivity trade-off in powder-bed additive manufacturing”, Progress in Additive Manufacturing, Vol. 5 No. 2, pp. 199-210, doi: 10.1007/s40964-020-00122-w.
Kumbhar, N.N. and Mulay, A.V. (2018), “Post processing methods used to improve surface finish of products which are manufactured by additive manufacturing technologies: a review”, Journal of The Institution of Engineers (India): Series C, Vol. 99 No. 4, pp. 481-487, doi: 10.1007/s40032-016-0340-z.
Laakso, P., Riipinen, T., Laukkanen, A., Andersson, T., Jokinen, A., Revuelta, A. and Ruusuvuori, K. (2016), “Optimization and simulation of SLM process for high density H13 tool steel parts”, Physics Procedia, Vol. 83, pp. 26-35, doi: 10.1016/j.phpro.2016.08.004.
Lantzsch, T., Heussen, D., Praetzsch, N. and Häfner, C. (2022), “Evaluation of productivity scaling approaches for laser powder bed fusion of nickel-base alloy 625”, 5, doi: 10.1117/12.2611151.
Laohaprapanon, A., Jeamwatthanachai, P., Wongcumchang, M., Chantarapanich, N., Chantaweroad, S., Sitthiseripratip, K. and Wisutmethangoon, S. (2012), “Optimal scanning condition of selective laser melting processing with stainless steel 316L powder”, Advanced Materials Research, Vol. 341–342, pp. 816-820, doi: 10.4028/WWW.SCIENTIFIC.NET/AMR.341-342.816.
Leicht, A., Rashidi, M., Klement, U. and Hryha, E. (2020), “Effect of process parameters on the microstructure, tensile strength and productivity of 316L parts produced by laser powder bed fusion”, Materials Characterization, Vol. 159, doi: 10.1016/j.matchar.2019.110016.
Leicht, A., Fischer, M., Klement, U., Nyborg, L. and Hryha, E. (2021), “Increasing the productivity of laser powder bed fusion for stainless steel 316L through increased layer thickness”, Journal of Materials Engineering and Performance, Vol. 30 No. 1, pp. 575-584, doi: 10.1007/s11665-020-05334-3.
Lieberwirth, C., Harder, A. and Seitz, H. (2017), “Extrusion based additive manufacturing of metal parts”, Journal of Mechanics Engineering and Automation, Vol. 7 No. 2, doi: 10.17265/2159-5275/2017.02.004.
Liu, Y., Zhang, M., Shi, W., Ma, Y. and Yang, J. (2021), “Study on performance optimization of 316L stainless steel parts by High-Efficiency selective laser melting”, Optics & Laser Technology, Vol. 138, doi: 10.1016/j.optlastec.2020.106872.
Liu, Y., Wu, Z., Wang, Q., Zhao, L., Zhang, X., Gao, W., Xu, J., Song, Y., Song, X. and Zhang, X. (2022), “Optimization of parameters in laser powder bed fusion TA15 titanium alloy using Taguchi method”, Crystals, Vol. 12 No. 10, p. 1385, doi: 10.3390/cryst12101385.
Makoana, N.W., Yadroitsava, I., Möller, H. and Yadroitsev, I. (2018), “Characterization of 17-4ph single tracks produced at different parametric conditions towards increased productivity of lpbf systems—the effect of laser power and spot size upscaling”, Metals, Vol. 8 No. 7, doi: 10.3390/met8070475.
Marak, Z.R., Tiwari, A. and Tiwari, S. (2019), “Adoption of 3D printing technology: an innovation diffusion theory perspective”, International Journal of Innovation, Vol. 7 No. 1, pp. 87-103, doi: 10.5585/iji.v7i1.393.
Mertens, R., Clijsters, S., Kempen, K. and Kruth, J.P. (2014), “Optimization of scan strategies in selective laser melting of aluminum parts with downfacing areas”, Journal of Manufacturing Science and Engineering, Vol. 136 No. 6, doi: 10.1115/1.4028620.
Nguyen, D.S., Park, H.S. and Lee, C.M. (2020), “Optimization of selective laser melting process parameters for Ti-6Al-4V alloy manufacturing using deep learning”, Journal of Manufacturing Processes, Vol. 55, pp. 230-235, doi: 10.1016/j.jmapro.2020.04.014.
Obilanade, D., Dordlofva, C. and Törlind, P. (2021), “Surface roughness considerations in design for additive Manufacturing-A literature review”, INTERNATIONAL CONFERENCE ON ENGINEERING DESIGN, ICED21, 16-20, doi: 10.1017/pds.2021.545.
Page, M.J., McKenzie, J.E., Bossuyt, P.M., Boutron, I., Hoffmann, T.C., Mulrow, C.D., Shamseer, L., Tetzlaff, J.M., Akl, E.A., Brennan, S.E., Chou, R., Glanville, J., Grimshaw, J.M., Hróbjartsson, A., Lalu, M.M., Li, T., Loder, E.W., Mayo-Wilson, E., McDonald, S., … Moher, D. (2021), “The PRISMA 2020 statement: an updated guideline for reporting systematic reviews”, BMJ, Vol. 372, doi: 10.1136/BMJ.N71.
Pannitz, O., Großwendt, F., Lüddecke, A., Kwade, A., Röttger, A. and Sehrt, J.T. (2021), “Improved process efficiency in laser‐based powder bed fusion of nanoparticle coated maraging tool steel powder”, Materials, Vol. 14 No. 13, doi: 10.3390/ma14133465.
Pauzon, C., Forêt, P., Hryha, E., Arunprasad, T. and Nyborg, L. (2020a), “Argon-helium mixtures as Laser-Powder bed fusion atmospheres: towards increased build rate of Ti-6Al-4V”, Journal of Materials Processing Technology, Vol. 279, doi: 10.1016/j.jmatprotec.2019.116555.
Pauzon, C., Leicht, A., Klement, U., Forêt, P. and Hryha, E. (2020b), “Effect of the process gas and scan speed on the properties and productivity of thin 316L structures produced by Laser-Powder bed fusion”, Metallurgical and Materials Transactions A, Vol. 51 No. 10, pp. 5339-5350, doi: 10.1007/s11661-020-05923-w.
Pfaff, A., Jäcklein, M., Schlager, M., Harwick, W., Hoschke, K. and Balle, F. (2020), “An empirical approach for the development of process parameters for laser powder bed fusion”, Materials, Vol. 13 No. 23, pp. 1-20, doi: 10.3390/ma13235400.
Qu, M., Guo, Q., Escano, L.I., Nabaa, A., Fezzaa, K. and Chen, L. (2022), “Nanoparticle-enabled increase of energy efficiency during laser metal additive manufacturing”, Additive Manufacturing, Vol. 60, doi: 10.1016/j.addma.2022.103242.
Rayyan (2024), available at: www.rayyan.ai/ (accessed 12 September 2023).
Ruffo, M., Tuck, C. and Hague, R. (2006), “Cost estimation for rapid manufacturing-laser sintering production for low to medium volumes”, Proceedings of the Institution of Mechanical Engineers, Part B: Journal of Engineering Manufacture, Vol. 220 No. 9, pp. 1417-1427.
Ruppert, D.S., Harrysson, O.L.A., Marcellin-Little, D.J., Abumoussa, S., Dahners, L.E. and Weinhold, P.S. (2017), “Osseointegration of coarse and fine textured implants manufactured by electron beam melting and direct metal laser sintering”, 3D Printing and Additive Manufacturing, Vol. 4 No. 2, pp. 91-97.
Salem, H., Abouchadi, H. and El Bikri, K. (2020), “Design for additive manufacturing”, Journal of Theoretical and Applied Information Technology, Vol. 10 No. 19, pp. 3043-3054, doi: 10.1201/9780429466236-7.
Schnabel, T., Oettel, M. and Mueller, B. (2017), DESIGN FOR ADDITIVE MANUFACTURING Guidelines and Case Studies for Metal Applications, Fraunhofer IWU, available at: www.additiv.fraunhofer.de/content/dam/rapidprototyping/de/documents/Studien/Designfor-AM_Guidelines-and-case-studies-for-metal-applications.pdf
Scholarcy (2024), available at: www.scholarcy.com/ (accessed 12 September 2023).
Scopus (2024), available at: www.scopus.com/ (accessed 12 September 2023).
Semantic Scholar Academic Graph API (2024), available at: www.semanticscholar.org/product/api (accessed 12 September 2023).
Shi, W., Li, J., Jing, Y., Liu, Y., Lin, Y. and Han, Y. (2022), “Combination of scanning strategies and optimization experiments for laser beam powder bed fusion of Ti‐6Al‐4V titanium alloys”, Applied Sciences, Vol. 12 No. 13, doi: 10.3390/app12136653.
Shipley, H., McDonnell, D., Culleton, M., Coull, R., Lupoi, R., O’Donnell, G. and Trimble, D. (2018), “Optimisation of process parameters to address fundamental challenges during selective laser melting of Ti-6Al-4V: a review”, International Journal of Machine Tools and Manufacture, Vol. 128, pp. 1-20, doi: 10.1016/j.ijmachtools.2018.01.003.
Shoukr, D., Morcos, P., Sundermann, T., Dobrowolski, T., Yates, C., Jain, J.R., Arróyave, R., Karaman, I. and Elwany, A. (2023), “Influence of layer thickness on the printability of nickel alloy 718:a systematic process optimization framework”, Additive Manufacturing, Vol. 73, p. 103646, doi: 10.1016/j.addma.2023.103646.
Silvi.ai (2024), available at: www.silvi.ai/ (accessed 12 September 2023).
Sinico, M., Metelkova, J., Dalemans, T., Thijs, L. and Van Hooreweder, B. (2022), “High speed laser powder bed fusion of M789 tool steel with an optimized 120 µm layer thickness approach”, Procedia CIRP, Vol. 111, pp. 162-165, doi: 10.1016/j.procir.2022.08.141.
Slodczyk, M., Ilin, A., Kiedrowski, T., Schmiemann, J. and Ploshikhin, V. (2021), “Simulation aided process development with Multi-Spot strategies in laser Powder-Bed fusion”, Advanced Materials Research, Vol. 1161, pp. 75-82, doi: 10.4028/www.scientific.net/AMR.1161.75.
Sobota, V.C.M., van de Kaa, G., Luomaranta, T., Martinsuo, M. and Ortt, J.R. (2020), “Factors for metal additive manufacturing technology selection”, Journal of Manufacturing Technology Management, Vol. 32 No. 9, pp. 26-47, doi: 10.1108/JMTM-12-2019-0448.
Sohrabi, N., Jhabvala, J. and Logé, R.E. (2021), “Additive manufacturing of bulk metallic glasses—process, challenges and properties: a review”, In Metals, Vol. 11 No. 8, doi: 10.3390/met11081279.
Sun, Z., Tan, X., Tor, S.B. and Yeong, W.Y. (2016), “Selective laser melting of stainless steel 316L with low porosity and high build rates”, Materials & Design, Vol. 104, pp. 197-204, doi: 10.1016/j.matdes.2016.05.035.
Tang, X., Zhang, S., Zhang, C., Chen, J., Zhang, J. and Liu, Y. (2020), “Optimization of laser energy density and scanning strategy on the forming quality of 24CrNiMo low alloy steel manufactured by SLM”, Materials Characterization, Vol. 170, doi: 10.1016/j.matchar.2020.110718.
Ten, J.S., Seet, H.L. and Nai, S.M.L. (2020), “Scaling up build rates in laser powder bed fusion of metals using dimensionless parameters”, Minerals, Metals and Materials Series, pp. 469-479, doi: 10.1007/978-3-030-36296-6_44/COVER.
Thomas, D. and Gilbert, S. (2014), “Costs and cost effectiveness of additive manufacturing”, A Literature Review and Discussion, pp. 1-77, doi: 10.6028/NIST.SP.1176.
Udroiu, R., Braga, I.C. and Nedelcu, A. (2019), “Evaluating the quality surface performance of additive manufacturing systems: methodology and a material jetting case study”, Materials, Vol. 12 No. 6, doi: 10.3390/ma12060995.
UKRI (2020), PAS 6001:2020 Factors to Be Considered in Making and Assessing the Business Case for Additive Manufacturing and 3D Printing – Guide, BSI Standards Limited 2020, ISBN: 9780539043105, available at: www.bsigroup.com/en-GB/insights-and-media/insights/brochures/pas-6001-the-businesscase-for-additive-manufacturing-and-3d-printing/
Wang, P., Chen, D., Fan, J. and Li, G. (2023), “Study on the organization and properties of Ti–6Al–4 V fabricated by laser powder bed fusion based on the thickness of the gradient layer”, The International Journal of Advanced Manufacturing Technology, Vol. 126 No. 5-6, pp. 2249-2267, doi: 10.1007/s00170-023-11269-5.
Wang, M.-R., Huang, Y.-S., Tseng, W.-C., Lai, K.-L. and Hwang, S.-J. (2015), “Production of gas-atomized aluminum alloy powders for metal additive manufacturing processes”, Journal of the Chinese Society of Mechanical Engineers, Transactions of the Chinese Institute of Engineers, Series C/Chung-Kuo Chi Hsueh Kung Ch’eng Hsuebo Pao, Vol. 36 No. 6, pp. 557-561.
Wang, Z., Xiao, Z., Tse, Y., Huang, C. and Zhang, W. (2019), “Optimization of processing parameters and establishment of a relationship between microstructure and mechanical properties of SLM titanium alloy”, Optics & Laser Technology, Vol. 112, pp. 159-167, doi: 10.1016/j.optlastec.2018.11.014.
Wang, D., Yang, Y., Zhang, M., Lu, J., Liu, R. and Xiao, D. (2013), “Study on SLM fabrication of precision metal parts with overhanging structures”, Proceedings - 2013 IEEE International Symposium on Assembly and Manufacturing, ISAM 2013, 222-225, doi: 10.1109/ISAM.2013.6643532.
Wan, L., Xia, Z., Song, Y., Zhang, X., Liu, F., Fu, G. and Shi, S. (2020), “Parameter optimization of selective laser melting fabricated titanium alloy using skin-core and triple contour scanning strategy”, Journal of Laser Applications, Vol. 32 No. 4, p. 042001, doi: 10.2351/7.0000180.
Wang, M.R.R., Lai, K.L.L., Tseng, W.C.C., Chen, K.Y.Y., Chen, Z.H.H., Wu, L.T.T. and Huang, Y.S.S. (2015), “Production of aluminum alloy and stainless steel powder for additive manufacturing by gas atomization processes”, available at: www.scopus.com/inward/record.uri?eid=2-s2.0-85091993305&partnerID=40&md5=304995e7e07ab08546bdecbe1f6fc9bb
Web of Science (2024), available at: www.webofscience.com (accessed 12 September 2023).
Wohler, T. (2021), “Wohlers report 2021 (2021st ed.)”, available at: https://wohlersassociates.com/2021report.htm
Yonehara, M., Ikeshoji, T.-T., Nagahama, T., Mizoguchi, T., Tano, M., Yoshimi, T. and Kyogoku, H. (2020), “Parameter optimization of the high-power laser powder bed fusion process for H13 tool steel”, The International Journal of Advanced Manufacturing Technology, Vol. 110 No. 1-2, pp. 427-437, doi: 10.1007/s00170-020-05879-6/Published.
Zadpoor, A.A. (2019), “Additively manufactured porous metallic biomaterials”, Journal of Materials Chemistry B, Vol. 7 No. 26, doi: 10.1039/c9tb00420c.
Acknowledgements
Credit authorship contribution statement:Financial support statement: No funding has been received for this study.
Declaration of competing interests: The authors declare that they have no known competing financial interests or personal relationships that could have appeared to influence the work reported in this paper.
Data availability: The data used and/or analysed in the current study are contained within the manuscript or available from the corresponding author on reasonable request.