Citation
Bastos, M. (2022), "Editorial: Five challenges in detection and mitigation of disinformation on social media", Online Information Review, Vol. 46 No. 3, pp. 413-421. https://doi.org/10.1108/OIR-08-2021-563
Publisher
:Emerald Publishing Limited
Copyright © 2021, Emerald Publishing Limited
1. Introduction
The colonization of Internet communities by social media in the late noughties was accompanied by the steady transition from networked publics, centered on a user-centric and decentralized governance framework, to algorithmic-driven commercial platforms. These services built much of their social infrastructure on the back of networked publics and the community organization that shaped Internet services in the early 1990s. In the early twenty-teens, social platforms consolidated their grip on the social infrastructure by replacing desktop-based applications with mobile platforms, a transition that substituted open standards with cloud-based, centralized application interfaces controlled by social media platforms. By the early 2010s, social platforms completed the transition by pivoting from a business model centered on software and services to the leasing and trading of user data (Bastos, 2021a).
This infrastructural transformation of the networked publics replaced legacy infrastructure of public, open and collaborative spaces with private and walled-off platforms. Social media platforms became centralized gatekeepers to critical infrastructure supporting economic, democratic and social participation. Operating in an open market with limited regulation or external oversight, social platforms flourished in an environment that supported the continuous upscaling of social infrastructure with no public-facing system of governance. This change in the social infrastructure has driven anxieties about social media and the prevalence of mis- and disinformation, digital privacy, data access, surveillance, microtargeting and the growing influence of algorithms in society (Bastos, 2021b). In the following, we contribute to this debate by addressing the politics of deletion setting data privacy and access policy against research on influence operations. This problem is unpacked against the backdrop of five key challenges in detection and mitigation of disinformation on social platforms.
2. We cannot know what we do not know
Resource allocation and planning for influence operations is notoriously time consuming, but the timeframe of disinformation campaigns is typically short. Influence operations leverage the “firehose of falsehood” model (Paul and Matthews, 2016), whereby a large number of messages are broadcast rapidly, repetitively, and continuously over multiple channels without commitment to consistency or accuracy (Bertolin, 2015). Content flagged by social platform's algorithms and partnering fact-checking agencies is quickly removed so that disinformation is phased out and disappears as soon as rectifying information emerges. As such, effective mitigation strategies require tracking disinformation in real-time and considerable resources have been allocated by social platforms to this effect. Unfortunately, the general public and the research community are not privy to such internal operations and therefore do not know, indeed cannot know, what they do not know.
Our study of the Brexit referendum campaign offers a cautionary tale in this respect. We analyzed tweet decay in three million posts leading up to the vote and compared it with data from the same period, and finally to a database of Brexit-related tweets encompassing nearly four years of Twitter activity. While studies prior to 2016 found that on average less than 4% of tweets would disappear from the platform (Bagdouri and Oard, 2015; Xu et al., 2013), tweet decay in the Brexit referendum campaign was remarkably higher. Indeed, 33% of the tweets leading up to the Brexit referendum vote have been removed from Twitter. Deletion is not restricted to tweets, but to accounts as well: only about half of the most active accounts that tweeted the Brexit referendum continue to operate publicly. From the entire universe of accounts that tweeted the referendum, 20% are no longer active, and 20% of these accounts were actively blocked by Twitter. While the accounts suspended by Twitter are under 5%, they posted nearly 10% of the entire referendum data.
Perhaps more worryingly, there are more messages associated with the Leave campaign that disappeared than the entire universe of tweets affiliated with the Remain campaign (Bastos, 2021c). In fact, the list of hashtags tweeted over one thousand times with a deletion rate of 40% or higher is largely restricted to Leave terms: voteleave, votein, leaveeu, ivoted, voteout, beleave, cameron, inorout, ukip and eng. For this set of hashtags, most of the messages tweeted in the period leading up to the vote are no longer available (
But tweet decay was rarely as low as 4%, the maximum estimate found in studies prior to 2016. From dozens of hashtags archived in 2016 and rehydrated in 2019 (Twitter, 2019), we found that 15% was the deletion baseline for non-political, uncontroversial hashtags, a baseline that increases sharply as the issue at stake becomes contentious. Tweet deletion in protest hashtags tweeted worldwide was similar to non-partisan Brexit hashtags at nearly 30%. Tweet decay in openly partisan hashtags associated with the Leave campaign, however, was much higher at 42% on average. Indeed, three-quarters of the content hashtagged with #betteroffout has been removed from Twitter and more than half of the tweets hashtagged with #voteleave, the official campaign to leave the EU, is no longer available. While the volume of political content removed from Twitter is astonishingly high, there is also evidence that social platforms are removing more content in general and systematically purging accounts. In sharp contrast to the 4% baseline of tweet decay reported before 2016, we found that even non-political messages are being purged at a rate at least three times as high. Given the above, it is difficult to rely on social media as a record for public deliberation, as the public record disappears with no recourse for recovery.
3. Back engineering social platforms
Social platforms – including Facebook, arguably the worst offender – deserve some measure of sympathy for trying to juggle the conflicting priorities of privacy, transparency and safety, while policymakers demand smooth integration of cultures and nations with minimal political and cultural conflict. These competing pressures offered no incentives for social platforms to increase access to the data they collected or to increase the transparency in their policies for content removal. As such, the public accountability of social platforms is severely limited by digital privacy concerns that feed their increasing opaqueness, which in turn further skews the balance of power between the social platforms and users or the general public.
This situation has forced researchers and journalists monitoring disinformation campaigns to work with fragmentary evidence and second-guess the algorithmic decisions that resulted in the purging or downranking of content. The reverse engineering of social platforms, commonly referred to as “algorithmic auditing,” requires extensive deep digging into disinformation as it happens in real time and with limited support from social platforms. Even when individual users and journalists report potential disinformation campaigns, social platforms rarely disclose content that was flagged for removal, and therefore studying influence operations on social media becomes an exercise in reverse engineering at multiple levels, with the most prominent being the interplay between the strategies and intentions of malicious state and non-state actors and the limited amount of evidence (data) made available by social platforms. This has severely hindered the identification of influence operations in real time, which are currently carried out only retrospectively, after large influence operations have already inflicted damage.
And there is much that the academic community could contribute to the study of influence operations. In our analysis of the visual frames employed by the Internet Research Agency (IRA), a Russian company specialized in online influence operations, we identified chromatic and compositional choices in the selection of profile photos, broadly curated to embody the vox populi with relatable, familiar or attractive faces of ordinary people (Figure 1). US conservative profiles featured average young men and sensual young women with sophisticated makeup in professionally shot photos against a soft, balanced lighting typical of soft advertisements employed by the cosmetic industry. Profile images in the Russian cohort, on the other hand, featured tropes of virility and domination, with a topical emphasis on compositional undertones emphasizing power and adventure. Black Lives Matter activists, finally, were largely depicted with high angles projecting tense expressions in a single headshot. These compositional choices did not merely depict gender inequalities and gendered stereotypes supporting traditional notions of femininity and masculinity. More than merely reflecting entrenched inequalities, the compositional tropes explored by the IRA celebrated racial and gender discrimination (Bastos et al., 2021a).
The compositional choices shown in Figure 1 attest to IRA's cultural acuity and familiarity with the social identity of their targets, a cohort of users who only learned about their targeting years after social platforms had identified and removed the threat (Hern, 2017). The routine removal of influence operations out of the public eye encourages operations to infiltrate cultural subgroups to sow distrust among targeted communities. The guy next door explored by the IRA campaign is the embodiment of unassuming, dependable, yet average masculinity. He was not just ordinary, but also local, thus submitting a sense of familiarity that could effortlessly evolve to notions of reliability and trustworthiness, thereby easing the labor-intensive costs of infiltration. Similarly, the cohort of implausibly attractive young women follows an analogous tactic of infiltration and subversion that exploited the male gaze and the depiction of women as sexual objects. This analysis, however, was only possible due to Twitter's Elections Integrity initiative that offered comprehensive data about the IRA (Elections Integrity, 2018). Attributing a disinformation campaign to specific actors remains largely in the hands of social platforms, who control high-quality signal and metadata necessary for attribution.
4. Gaslighted by social platforms
Influence operations routinely daisy chain multiple harassment and disinformation campaigns that are phased out and disappear as soon as rectifying information or alternative stories starts to emerge. The low persistence and high ephemerality of social media posts are leveraged to transition from one contentious and unverified frame to the next before mechanism for checking and correcting false information are in place. As such, influence operations can easily exploit the opaqueness and inscrutability of social platforms by offloading problematic content that is removed from platforms before the relentless – but ultimately time consuming – news cycle has successfully corrected the narratives championed by highly volatile social media content.
The absence of accountability and oversight mechanisms for social platform, and a context where influence operations can easily leverage the firehose of falsehood, maximizes the vulnerability of those targeted by influence operations. Individuals find themselves unable to tell whether mass harassment and brigading are coordinated or not, and the decision-making process regarding content that has been reported or flagged for removal is restricted to social platforms' content policy team, who decides on individual cases with little to no external input. The opaqueness and the politics of deletion implemented by social platforms are beneficial to influence operations because disinformation performs well in short timeframes. Even when content is routinely removed, the high-volume posting is effective because individuals are more likely to be persuaded if a story, however confusing, appears to have been reported repetitively and by multiple sources.
To be sure, social platforms faced mounting pressure from the public and elected officials to curb mis- and disinformation, leading to the allocation of substantial resources to the removal of problematic content, including mis- and disinformation, from their platforms. Their response is largely focused on boosting their fact-checking partnerships, with Facebook alone working with over 80 partners and a range of semi- or fully automated systems to flag and remove misinformation. Indeed, the number of fact-checking organizations more than doubled since 2016, reaching 304 organizations in 84 different countries worldwide (Stencel and Luther, 2020). This governance and policy decision appear effective at face value, as fact-checking is posited as the diametrical opposite of misinformation and provides evidence to rebut the inaccuracies advanced to mislead individuals. Fact-checks would thus correct and remove problematic content by verifying and rectifying false claims based on authoritative sources, a seemingly uncontroversial task critical to a well-functioning public debate.
But there remains fundamental questions about fact-checking limitations in addressing problems that do not occur in isolation, but stem from broader social tensions, technological affordances, and partisan and sectarian fault lines (Benkler et al., 2018). Fact-checking remains the primary initiative backed by social platforms and policymakers to detect and mitigate mis- and disinformation, but fact-checking was not designed to offset, remove or contain the spread of misinformation. Fact-checking was originally devised as a tool to evaluate political claims and hold politicians to account, and yet it has gradually become the cornerstone of policies and initiatives devoted to correcting false and deceiving content on social media (Graves and Mantzarlis, 2020; Singer, 2020). It is difficult to see how fact-checking can be repurposed to scale up to the speed, velocity and magnitude of content shared on social media.
Figure 2 unpacks this limitation by showing original content that was fact-checked and removed from Twitter, only to resurface multiple times from different sister accounts. Taken from a database of the 2018 US election, it identifies tweets and webpages that disappeared after the election cycle. In the above example, the deleted content resurfaces via other accounts that repost the original webpage on other similarly hyperpartisan websites. The original tweet ID 991023408816250880 featured the headline “Outrageously Unreasonable Arizona Teachers Strike Is Illegal,” but the tweet is no longer available on Twitter, nor is the post from conspiracyoutpost.com identified with the shortened URL t.co/jQWtQmYcPW. After the post was fact-checked and removed, however, five tweets featuring the same headline and directing to various sister websites, including “Grumpy Opinions” at grumpyelder.com and “Moonbattery” at moonbattery.com, appeared on Twitter. Mis- and disinformation can thus continue to live on Twitter through a process of continuous reposting, recycling and resourcing to other sister accounts and URL domains (Bastos et al., 2021b).
5. On social media, no one knows you are a bot
The limitations of fact-checking with respect to the scale and speed of social media content is well understood by social platforms. To this end, substantial resources have been allocated to scalable solutions based on machine learning and predictive analytics (i.e. “artificial intelligence”). Data analytics and machine learning algorithms present a point of departure from statistical analyses based on probability distributions that measure significance and grounded much of the social sciences in the past century. It is unclear, however, whether machine learning as a scientific framework can bring a measure of control to this information ecosystem, not the least because machine learning is also available and can be promptly leveraged by influence operations. Indeed, the use of computer-generated profile images has become a staple in influence operations on social media and is also becoming the de facto standard in influence operations and state propaganda (Satariano, 2021).
These are long standing challenges for research in bot identification. Even state-of-the-art classifiers are imprecise when it comes to identifying social bots and estimating automated activity. The scores are prone to variance and likely to lead to false negatives (i.e. bots being classified as humans) and false positives (i.e. humans being classified as bots), particularly for accounts posting content in languages other than English (Rauchfleisch and Kaiser, 2020). In our own studies where a large botnet was identified (Bastos and Mercea, 2018), we found that even though bots rely on trivial computing routines, bot detection was not an exact science and neither human annotators nor machine-learning algorithms would perform particularly well. Moreover, bot detection tools like Botometer rely on Twitter REST API and therefore can only inspect active accounts. With Twitter efficiently detecting and removing bots, researchers cannot rely on bot classifiers to study account activity retrospectively or to analyze accounts that have been deleted, suspended or set to private.
In our study of the Brexit botnet (Bastos and Mercea, 2019), the Twitter account @nero was identified as a bot (Figure 3). This was likely a false positive, as the account was seemingly operated by the alt-right controversialist and professional troll Milo Yiannopoulos. The account was highly connected to the remainder of the botnet and disappeared in the same period. Although the account was publicly identified as belonging to a human user, there is some indication that automation may have been employed. Having tweeted over 175K messages before being removed from Twitter, this human operator would have to have consistently tweeted 60 messages every day throughout the 8-year period the account was active (Figure 4). Regardless of the level of automation employed by @nero, the account was indeed retweeted by many of the bots identified in our original study, with Figure 3 showing the hub-and-spoke formation resulting from the interaction between this user and other bot-like accounts.
Social platforms also contribute to making research into automated, bot-like networks more difficult because very little data on influence operations using bots and botnets have been made available. Despite the best efforts of researchers, there is no reliable way to understand the full scope of mis- and disinformation or their tactics because no representative data on their scale is currently available. While automated accounts are taken down to protect organic interactions, the disappearance of these accounts makes it impossible to carry out forensic analysis of bots that operated in disinformation campaigns. Equally important, the distinction between automated and supervised information warfare has remained peripheral to public deliberations. Indeed, supervised high-volume posting is a new strategy in the political arena to which very limited attention has been given.
6. If you cannot see it, did it happen at all?
The lockdown of social platform's application programming interface (APIs), especially that of Facebook and Instagram in the wake of the Cambridge Analytica data scandal and the Congressional hearings post-2016, has hindered research on influence operations and disinformation in meaningful ways. Mitigation strategies available to the public and the academic community are inadequate because independent source attribution is near impossible in the absence of digital forensics. The monitoring tools made available after the lockdown of APIs, including data access facilitated by Social Science One and CrowdTangle, which is owned by Facebook, are fundamentally imperfect because no direct access to data is possible. Similarly, while Twitter has offered archives of disinformation campaigns that the company identified and removed (Elections Integrity, 2018), such sanctioned archives offer only a partial glimpse into the extent of influence operations and may prevent researchers from examining organic contexts of manipulation (Acker and Donovan, 2019).
With no access to Facebook and Instagram data, arguably the most important platforms for propaganda and influence operations, independent source attribution and the monitoring of disinformation is near impossible. As such, our understanding of what constitutes mis- and disinformation and how widespread the problem is on social platforms is tied to, and depends on, the fragmented data that platforms such as Twitter and Facebook release to limited segments of the academic community, usually research institutions that have stroke an agreement with the companies. This rather limited sample of disinformation campaigns effectively shapes our understanding of what strategies are in place, how large these networks of disinformation are, and what strategies of mitigation can be employed to control the spread of problematic content.
The available data are in no way representative of various disinformation campaigns occurring on the platforms. It is often the case that influence operations are identified by researchers and journalists unaffiliated with the social platforms, so no one has a complete picture of the strategies taking place online at any given time. Even disinformation circulating on public platforms like Twitter can only be detected to a limited extent. This is because Twitter's Terms of Service state that content deleted by a user or blocked by the platform due to infringements on the ToS ought to disappear from the platform altogether (Twitter, 2019). Similarly, deleting a tweet automatically triggers a cascade of deletions for all retweets of that tweet. This specific affordance of social platforms facilitates the disappearance of posts, images and weblinks from the public view, with lasting effects to research on influence operations.
Lastly, even if the rapid turnover and short shelf life of social media content constitute an expected affordance of social media communication, it is hardly a desirable design of political communication and deliberation across social platforms that may be further subjected to artificial manipulation and false amplification. In other words, while the short shelf life of social media posts may be a reasonable expectation, as is the expectation that one should have control over its own personal data (Ausloos, 2012), this poses considerable challenges for deliberation and informed public debate around matters where the issue being deliberated on is constantly disappearing from public scrutiny (Bastos, 2021c).
Figures
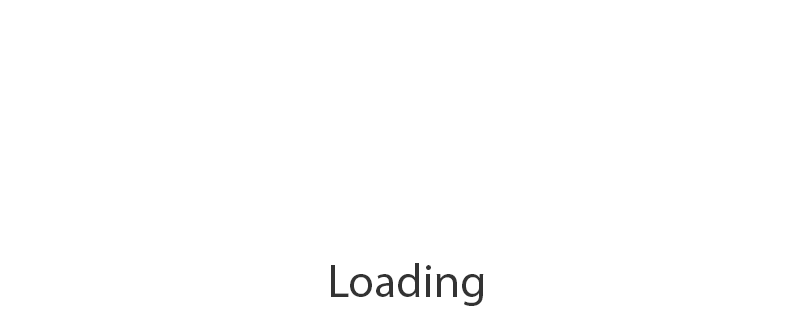
Figure 3
Tiered structure of the Brexit botnet identified in Bastos and Mercea (2019), with bots shown in red and active users in blue
References
Acker, A. and Donovan, J. (2019), “Data craft: a theory/methods package for critical internet studies”, Information, Communication and Society, Vol. 22 No. 11, pp. 1590-1609.
Ausloos, J. (2012), “The ‘right to be forgotten’– worth remembering?”, Computer Law and Security Review, Vol. 28 No. 2, pp. 143-152.
Bagdouri, M. and Oard, D.W. (2015), “On predicting deletions of microblog posts”, Proceedings of the 24th ACM International on Conference on Information and Knowledge Management: ACM.
Bastos, M.T. (2021a), “From global village to identity tribes: context collapse and the darkest timeline”, Media and Communication, Vol. 9 No. 3, pp. 50-58.
Bastos, M.T. (2021b), Spatializing Social Media: Social Networks Online and Offline, Routledge, London.
Bastos, M.T. (2021c), “This account doesn't exist: tweet decay and the politics of deletion in the Brexit debate”, American Behavioral Scientist, Vol. 65 No. 5, pp. 757-773.
Bastos, M.T. and Mercea, D. (2018), “The public accountability of social platforms: lessons from a study on bots and trolls in the Brexit campaign”, Philosophical Transactions of the Royal Society A: Mathematical, Physical and Engineering Sciences, Vol. 376 No. 2128, doi: 10.1098/rsta.2018.0003.
Bastos, M.T. and Mercea, D. (2019), “The Brexit botnet and user-generated hyperpartisan news”, Social Science Computer Review, Vol. 37 No. 1, pp. 38-54.
Bastos, M.T., Mercea, D. and Goveia, F. (2021a), “Guy next door and implausibly attractive young women: the visual frames of social media propaganda”, New Media and Society.
Bastos, M.T., Walker, S. and Simeone, M. (2021b), “The IMPED model: detecting low-quality information in social media”, American Behavioral Scientist, Vol. 65 No. 6, pp. 863-883.
Benkler, Y., Faris, R. and Roberts, H. (2018), Network Propaganda: Manipulation, Disinformation, and Radicalization in American Politics, Oxford University Press.
Bertolin, G. (2015), “Conceptualizing Russian information operations: info-war and infiltration in the context of hybrid warfare”, IO Sphere, pp. 10-11.
Elections Integrity (2018), Data Archive, 2018 ed., Twitter, Inc, October 17.
Graves, L. and Mantzarlis, A. (2020), “Amid political spin and online misinformation, fact checking adapts”, The Political Quarterly, Vol. 91 No. 3, pp. 585-591.
Hern, A. (2017), “Facebook to tell users if they interacted with Russia's ‘troll army’”, The Guardian.
Paul, C. and Matthews, M. (2016), The Russian “Firehose of Falsehood” Propaganda Model: Why It Might Work and Options to Counter It, Rand Corporation, pp. 2-7.
Rauchfleisch, A. and Kaiser, J. (2020), “The false positive problem of automatic bot detection in social science research”, PLoS One, Vol. 15 No. 10, e0241045.
Satariano, A. (2021), “Inside a pro-Huawei influence campaign”, The New York Times.
Singer, J.B. (2020), “Border patrol: the rise and role of fact-checkers and their challenge to journalists' normative boundaries”, Journalism, 1464884920933137.
Stencel, M. and Luther, J. (2020), “Fact-checking count tops 300 for the first time”, in Fact-Checking News, Duke Reporters' Lab, Durham.
Twitter (2019), “More about restricted uses of the Twitter APIs”.
Xu, J.-M., Burchfiel, B., Zhu, X. and Amy, B. (2013), “An examination of regret in bullying tweets”, Proceedings of the 2013 Conference of the North American Chapter of the Association for Computational Linguistics: Human Language Technologies.
Acknowledgements
This paper is based on a keynote address by the author to the CHIST-ERA Programme for European Coordinated Research, 19 May 2021.