Abstract
Purpose
The purpose of this paper is twofold: to identify and map contemporary research on advanced technology implementations for problem-solving purposes in the manufacturing industry, and to further understand the organizational learning possibilities of advanced technology problem-solving in the manufacturing industry.
Design/methodology/approach
This paper outlines a scoping review of contemporary research on the subject. The findings of the review are discussed in the light of theories of contradicting learning logics.
Findings
This paper shows that contemporary research on the subject is characterized by technological determinism and strong solution-focus. A discussion on the manufacturing industries’ contextual reasons for this in relation to contradicting learning logics shows that a Mode-2 problem-solving approach could facilitate further learning and expand knowledge on advanced technology problem-solving in the manufacturing industry. A research agenda with six propositions is provided.
Originality/value
The introduction of advanced technology implies complex effects on the manufacturing industry in general, while previous research shows a clear focus on technological aspects of this transformation. This paper provides value by providing novel knowledge on the relationship between advanced technology, problem-solving and organizational learning in the manufacturing industry.
Keywords
Citation
Mohlin, A. (2023), "How to facilitate manufacturing industry learning from problems: a review on advanced technology problem-solving", Journal of Workplace Learning, Vol. 35 No. 6, pp. 470-489. https://doi.org/10.1108/JWL-01-2023-0008
Publisher
:Emerald Publishing Limited
Copyright © 2023, Alice Mohlin.
License
Published by Emerald Publishing Limited. This article is published under the Creative Commons Attribution (CC BY 4.0) licence. Anyone may reproduce, distribute, translate and create derivative works of this article (for both commercial & non-commercial purposes), subject to full attribution to the original publication and authors. The full terms of this licence may be seen at http://creativecommons.org/licences/by/4.0/legalcode
Introduction
The introduction of advanced technology has brought expectations of a series of paradigm shifts in the nature of work. However, despite the big promises, many companies experience issues finalizing advanced technology projects (Westenberger et al., 2022), integrating new advanced technology into their operations (Sjödin et al., 2021), scaling up pilot projects, not seeing the predicted revolutionary change (Davenport and Ronanki, 2018; Fountaine et al., 2019) and generally not solving their problems with advanced technology as expected. In manufacturing industry in particular, these struggles are further combined with difficulties understanding the added value of the capabilities of advanced technology implementations (Burggräf et al., 2021). By being stuck in ad-hoc projects (PWC, 2021) and not seeing the expected benefits despite industrious efforts (Makarius et al., 2020), many companies in the manufacturing industry risk allocating resources to advanced technology problem-solving implementations that might never provide value.
Problems in organizations create suitable foundations for learning (Engström, 2014), and technological change most often affects current ways of working, hence triggering organizational learning in the manufacturing industry (Shahlaei and Snis, 2022). Thus, problems and problem-solving processes should be viewed as learning opportunities in relation to advanced technology to face potential implementation challenges (Davenport and Ronanki, 2018). However, when it comes to advanced technology, most companies lack an understanding of its capabilities and potential in their current approaches (Davenport and Ronanki, 2018; Kane, 2019; PWC, 2021). In addition, rapid technological changes in manufacturing industry tend to hinder forces that support organizational learning (Shahlaei and Snis, 2022), and the advanced technology currently finding its way into most operations is considered more complex than before (Davenport and Ronanki, 2018).
To support manufacturing companies in their advanced technology implementations for problem-solving purposes, more knowledge on the relationship between advanced technology, problem-solving and organizational learning is needed. As organizational change occurs in the field between existing and new knowledge (Watzlawick et al., 2011), theories of contradicting learning logics are applied in this research. The objective is twofold: to identify and map contemporary research in the field by conducting a scoping review, and to discuss the findings with theories of contradicting learning logics and problem-solving. To approach this objective, this paper aims to answer the following research questions:
“What is the state of contemporary research on advanced technology implementation for problem-solving in the manufacturing industry?”
“How can contemporary research on advanced technology implementation for problem-solving in manufacturing industry be understood from a learning perspective?”
Theory
Conceptualizing problems and problem-solving
Problems and problem-solving do not hold a single universal definition (Frensch and Funke, 1995) and have been systematically explored since the beginning of the 19th century, with roots in cognitive and gestalt psychology (D'zurilla and Goldfried, 1971; Dunbar and Fugelsang, 2005; Funke, 2013; Scheerer, 1963). Contemporary definitions stem from a mixture of disciplines (Frensch and Funke, 1995), including cognitive psychology (Thorndike, 1898), engineering (Woods et al., 1997), organizational learning (Edmondson, 2018), mathematics (Polya, 2004) and computer science (Newell and Simon, 1972). Regardless of context, the central meaning of problems and problem-solving do share some common denominators independent of conditions: there is a current state, there is a desired state and there is an actor who experiences disruption and lack of knowledge about potential courses of action to get from one to the other (Frensch and Funke, 1995). Thus, a problem is not a stand-alone task but an interaction between the problem characteristics and an actor (Frensch and Funke, 1995), resulting in a plethora of problem-solving methodologies. Based on a synthesis of problem-solving literature from different disciplines, a common, simplified process infers three stages, as illustrated in Figure 1. First, the problem is found/identified by the problem-solver, conceptually understood and its parameters defined; second potential solutions are found, identified, understood and evaluated; and finally, the most suitable solution is implemented (Basadur et al., 1982; Frensch and Funke, 1995; Mohaghegh and Furlan, 2020; Morgan, 2020; Newell and Simon, 1972; von Hippel and von Kroch, 2013).
How this process is approached is, according to Mohaghegh and Furlan (2020), dependent on a set of predetermined factors. One is the nature of the problem, in which ill-structured problems indicate complex interconnectivity of variables, often requiring unique and adjusted problem-solving approaches, while well-structured problems indicate transparent and recurring information about variables that allows for more standardized problem-solving approaches (Mohaghegh and Furlan, 2020). Other factors are time availability; a problem under time pressure may result in either jumping to unsustainable solutions too fast or even ending up solving the wrong problem, and information availability, as a lack of information about the problem variables and context leads to difficulties in finding a solution (Mohaghegh and Furlan, 2020). In addition, collaborative culture is an important factor, as it is found that individuals in collaboration outperform individuals working alone, and finally, environmental dynamism, inferring that the problem-solving approach is affected by external factors such as level of competition and level of technological development (Mohaghegh and Furlan, 2020). A change in the conditions surrounding the problem thus calls for a new problem-solving approach (Joksimovic et al., 2023).
Solving manufacturing industry problems with advanced technology
In this research, manufacturing industry is referred to as the sector of economy in which the final output is something produced from raw materials systematically using different kinds of machinery. Manufacturing industry problem-solving is thus commonly viewed as improvement-based initiatives (Mohaghegh and Größler, 2021) and is often approached with established methodologies such as Lean (Six Sigma, Kaizen, etc.) (Womack and Jones, 1997). Other methodologies include A3 Thinking, Root-Cause Analysis, 5-Why and Design Thinking (Liedtka, 2020; Mohaghegh and Furlan, 2020; Mohaghegh and Größler, 2021; Womack and Jones, 1997).
The introduction of new and advanced technology has, however, inferred a new era of problem-solving in the manufacturing industry, in which the integration of established approaches and new technological aspects is key (Joksimovic et al., 2023; Singh et al., 2021). Advanced technologies such as Artificial Intelligence, Internet of Things, Robotics and Additive Manufacturing are some examples that are considered the main pillars for building the future of industry (Zheng et al., 2021), and analytical tools related to these technologies provide both production optimization, forecasting, logistics and decision support opportunities in internal processes (Frank et al., 2019; Von Krogh, 2018). A recent study by Shahlaei and Snis (2022) shows that organizations in manufacturing industry, when faced with technological change, tend to adapt quickly as they are somewhat forced to engage in explorative possibilities. However, findings also show that technological change tends to be rapid in nature and sometimes hinders the reflection time needed to adjust and learn properly (Shahlaei and Snis, 2022). Findings by Engström (2014) further show that technological problems tend to be prioritized in organizational settings, and although there is considerable knowledge on the technologies and their meaning for innovation, there is still a knowledge gap related to many of the circumstantial aspects, such as organizational impact or effect (Ivaldi et al., 2022). Subsequently, the revolutionary promises of advanced technology implementations are at times referred to as a hype (Davenport and Ronanki, 2018; Jarrahi, 2018).
A recent review by Joksimovic et al. (2023) on the application of Artificial Intelligence (AI) in problem-solving activities mapped contemporary research on the subject and found that many studies are indeed found in the areas of engineering and industry; however, both theory development and implementation focused studies are not prioritized. It has also been found that research connected to Industry 4.0 is most often focused on the actual technologies and optimization problems (Zheng et al., 2021), and research on advanced technology in general tends to have a strong solution focus (Sturm et al., 2021). Despite these focuses in research, companies face a lot of other problems with different characteristics daily, including problems related to worker skills, inventory, supply chain disruptions, worker safety, consumer trends, maintenance and innovation.
Problem-solving and organizational learning
Similar to previous elaboration, in learning theory, problem-solving refers to the process of going from current to desired state, and the challenge of one’s existing knowledge, acting on new assumptions and learning from the results are what make problem-solving strongly connected to organizational learning (Argyris, 1976). The concept of organizational learning further refers to the study of collective learning and knowledge creation within an organization to increase adaptability, innovation and performance (Argyris and Schon, 1978). However, the review by Mohaghegh and Furlan (2020) shows that there is a consensus among scholars that not all problem-solving results in learning. As mentioned, a problem is not a problem until experienced as such by an actor (Frensch and Funke, 1995). Thus, one of the main foundations of problem-solving is cognitive processing (Mohaghegh and Furlan, 2020). According to the influential work by Newell and Simon (1972), problem-solving modes based on behavioural response are divided into two modes: searching for the most satisfying solution by trial-and-error (quick and dirty) or focusing on the problem analysis to arrive at a solution that keeps the problem from recurring (slow and steady). Different problems require different modes, and different terminology for these modes is used in different disciplines. Therefore, to simplify the use of terminology, this paper will hereon forth refer to these modes as Mode-1 and Mode-2 problem-solving and learning.
The trial-and-error mode, Mode-1, provides short-term improvements, in which the core idea is that the problem-solver approaches the problem with existing knowledge, behaviour and set of assumptions with the short-term goal of correcting an error. In learning and organizational change literature, this is referred to as single-loop learning (Argyris, 1976; Argyris and Schön, 1997), first-order change (Watzlawick et al., 2011), System-1 problem-solving (Mohaghegh and Furlan, 2020; Mohaghegh and Größler, 2021) or exploit (March, 1991). The work of Edmondson (2018) refers to this approach as learning from failures, in which mistakes are viewed as learning opportunities. Today, these experimental approaches to problem-solving have proved successful (Ferraro et al., 2015; Garud, 2020; Liedtka, 2020), and their core assumptions are often central to many of the aforementioned problem-solving methodologies used in the manufacturing industry. According to Liedtka (2020) and the ideas of Design Thinking, the success factor of this mode lies in its closeness to practice, which frees the problem-solving process from abstractness. Support for this can also be found in Schon’s (1984) ideas of reflecting in action, in which thinking is not separated from doing and thus facilitates learning in action. Although the Mode-1 approach is useful in certain situations due to its minimal requirements of resources, it may fail to contribute with sustainable solutions as attention is not put on the analysis and understanding of the problem (Mohaghegh and Größler, 2021).
The latter, slow and steady Mode-2, is about going to the root cause of the problem, engaging in analysis of its logical aspects and antecedents, and thus providing sustainable solutions based on careful analysis (Mohaghegh and Furlan, 2020). In learning theory and theory of organizational change, this is referred to as double-loop learning (Argyris, 1976; Argyris and Schön, 1997), second-order change (Watzlawick et al., 2011), System-2 problem-solving (Mohaghegh and Furlan, 2020; Mohaghegh and Größler, 2021) or explore (March, 1991). The aim is to test existing assumptions and expand knowledge about the problem instead of focusing on its solution (Mohaghegh and Furlan, 2020). Mode-2 infers learning from further investigating the problem and, hence, expanding knowledge on potential solutions as well as challenging existing behaviour (March, 1991; Mohaghegh and Größler, 2021). It is useful for establishing sustainable solutions but could, however, result in an over-abstraction (as aforementioned stated by Liedtka, 2020), in which extensive resources might be allocated to non-applicable conceptual work. By engaging only in Mode-2, organizations may create an unstable system and lose the ability to leverage and use existing capabilities in their processes (Mohaghegh and Größler, 2021). However, the surge in technological change forces manufacturing companies to transform from production companies to development companies and thus engage in more of a Mode-2 approach (Ivaldi et al., 2022; Shahlaei and Snis, 2022).
Methods
Scoping reviews are characterized by covering the scope of a specific subject in existing literature and applying a systematic approach while objectively disregarding quality (Booth et al., 2012; Munn et al., 2018). The retrieval of the literature in this scoping review was conducted as a database keyword search in accordance with procedural guidance by Booth et al. (2012). The databases searched were Scopus, Web of Science, and ProQuest Central. Scopus and Web of Science were chosen because of their comprehensiveness. ProQuest Central was chosen for its multidisciplinary focus on social sciences, technology and business, which fit with the combination of concepts of interest in this review. As several online sources claim that Scopus is one of the most extensive databases, the searches in Scopus included all fields (title, abstract, full text), while searches in Web of Science and ProQuest Central were considered complementary and only included title searches. Scopus was searched several times with new combinations of search words as new knowledge about the field emerged during the screenings.
Block searchers were used, in which synonyms of two main concepts of interest (advanced technology and problem-solving) of the review were combined in different constellations using Boolean operators “OR” and “AND”. As the results showed which terms were frequently used, new keywords were added to the searches during the process. Keywords included: “problem-solving”, “problem-definition”, “problem-understanding”, “problem*”, “issue”, “challenge”, “artificial intelligence”, “AI”, “machine learning”, “ML”, “data-driven”, “industry 4.0”, “industry”, “organization” and “business”. For the inclusion criteria, only research published in the last 5 years was included to keep the focus on contemporary research. As mentioned, the term “problem” indicates different things in different subject areas. Inclusion criteria therefore included delimiting the search to areas such as “social science”, “business”, “engineering” and “management”, and excluding areas such as “mathematics” and purely “computer science” to maintain the focus on the problem term as defined in the theory section. However, it is here worth noting that the findings of the review included several papers directly connected to the field of computer science, despite the active choice to exclude this area. This is further discussed in the results section.
The search and review were inspired by and conducted using the preferred reporting items for systematic reviews and meta-analyses (PRISMA) flow procedure (PRISMA, 2021), as depicted in Figure 2. The keyword database search from all three databases resulted in a total of n = 1,695 articles. The first step of the selection process was a title screening, in which titles with a clear connection to and/or combination of the keywords used in the search were included. The results were in this stage condensed to n = 299. Followingly, an abstract screening was conducted, in which the articles with the focus on problem-solving, manufacturing industry and advanced technology were chosen for further examination. Articles not focusing on problem-solving or advanced technology were excluded, as were articles focusing on other contexts than manufacturing industry, such as education, healthcare or agriculture. Quality measures were also taken at this stage, as the journals in which the articles were published were searched in the Norwegian List (Scientific Publishing Knowledge Base). Articles published in journals rated as 1 or 2 according to the websites’ score system were included, as this meant that they were approved by a scientific editorial board, peer-reviewed, were internationally acknowledged and had approved International Standard Serial Numbers. This stage resulted in n = 126.
The next step was a full-text screening of the n = 126 articles, which meant re-reading the abstracts more carefully, as well as further screening the article in its entirety to get a sense of its content. Of the 126 articles, 28 were removed as they were duplicates. During the full-text screening stage, it became clear that the nature and characteristics of the articles were approaching saturation. Many studies reported on in the articles were becoming similar to a majority of the ones that had already been screened and did not provide additional knowledge in relation to the research questions. In all, 20 articles were therefore not included in the final selection after the full-text screening; three articles were removed, as they lacked a definite, concrete connection to advanced technology. The final selection of articles in the review was n = 35.
The final selection of articles was inserted into an Excel sheet, demographically described by study type, research area, methods, industrial focus, and problem-solving connection. Purely demographic characteristics such as publication year, country of origin and more were not included in this analysis as they were not of interest for the study objectives. The analysis was conducted in two stages: first, the demographic information about the final selection of articles was aggregated into a table (see Table 1) to map contemporary research on the subject. Secondly, the findings of the scoping review were discussed with theories of contradicting learning logics.
Results
First, as seen in Table 1, a clear majority of articles included in the review had a quantitative methodological approach in which computational experiments or simulations of a more or less abstract and often explorative nature were conducted. Many of these studies’ objectives were to test either established or new algorithms/models on either empirically collected data (Abdirad et al., 2021; Guo and Hesthaven, 2019; Duc and Nananukul, 2020) or on fictional datasets (e.g. Dias and Ierapetritou, 2019; Garbaya et al., 2022). In terms of research design, most of these studies first outlined a conceptual phase in which the algorithm/model is either developed or conceptually discussed, followed by a testing phase, and finally a discussion of the results. The two articles of conceptual nature were Francalanza et al. (2018), which discussed manufacturing systems as wicked problems, and Hobscheidt et al. (2021), which discussed and categorized common Industry 4.0 problems from a socio-technical perspective. Three articles were literature reviews on different subjects. Burggräf et al. (2021) meta-approached machine learning techniques for facility layout problems; Islam et al. (2020) examined the literature on machine learning application to an established optimization problem (well-placement problem), and Singh et al. (2021) reviewed the trends in machine learning for logistics problems.
As seen in Table 1, there was a rather even distribution between the research areas of electronics, engineering, computer science, operations management, information systems and multidisciplinary research in the results. Although the search strategy aimed to include research from social science areas such as business- and/or organization studies, none of the articles reviewed positioned themselves within the social sciences. It is further worth noting, again, that “computer science” was consciously excluded from the literature search but still constituted a significant part of the results. This result is considered important, as it supports the notion that the field is somewhat technologically deterministic.
A similar trend was observed when categorizing the industrial focus of the final selection of articles; only two articles reviewed focused on contexts of a “softer” nature. Here, de Jager and Nel (2019) studied workflows and business process automation focusing on image digitization, and Jhaver et al. (2019) examined the application of a prediction system to employee turnover. Furthermore, as seen in Table 1, there was also a connection to the methodological categorization. For example, articles studying areas in which operational problems were of focus, such as distribution- and facility-related, production- and manufacturing-related and machinery-focused, employed quantitative, experimental/simulation-based methods. Nonetheless, the results show that contemporary research on the subject holds a clear industrial focus on the operational level and somewhat neglects strategic and/or organizational processes in a manufacturing company.
Finally, the problem-solving connection of the reviewed articles is depicted in Table 1, aiming at categorizing the meaning and usage of the terms “problem” and “problem-solving” in the area. Another connection to the methodological and industrial focus of the reviewed articles is seen here, as articles focusing on the operational areas of a manufacturing industry while adopting quantitative methods also viewed problems as a situation to be solved and attempted solutions using technological tools (Agrawal et al., 2022; Chen et al., 2021; Dias and Ierapetritou, 2019; Fang et al., 2019; Pisacane et al., 2021 and several more). A smaller sample of the articles studied problem-solving from a methodological/process-based perspective. These included Bergès et al. (2021), who studied root-cause analysis approaches in relation to machine learning and data analytics, and Xu and Dang (2020), who focused on causal analysis in problem-solving using data-driven approaches. Three articles focused on studying and/or identifying problems per se, for example, Singh et al. (2021), who discuss different logistics-related problems and their connection to machine learning. Two articles focused on practical business problems: Jhaver et al. (2019) and employee turnover, and Vithitsoontorn and Chongstitvatana (2022) and demand forecasting.
Analysis and research agenda
The first research question of this paper is: “What is the state of contemporary research on advanced technology implementation for problem-solving in the manufacturing industry?”. Connecting back to the introduction of this paper, it is clear that expectations for using advanced technology to solve company problems on both strategic and operational level are high (Frank et al., 2019; Von Krogh, 2018). The scoping review results presented in Table 1 display two clear lines of thought that are discussed as answers to the question.
First, the results show a clear focus on operational-level problems, optimization problems in particular, and different quantitative approaches towards them, while problems of softer nature are not given equal attention. This finding is in accordance with conclusions by Engström (2014), stating that technological problems tend to be prioritized in organizational settings, and with Zheng et al. (2021), commenting that research on advanced technology in the manufacturing industry is generally focused on the actual technologies and, by extension, optimization problems. This finding could have a contextual explanation, as manufacturing industry has historically applied improvement-based problem-solving approaches, as exemplified in the theory section (Mohaghegh and Größler, 2021; Womack and Jones, 1997). As stated by Mohaghegh and Furlan (2020), the nature of the problem affects the suitable mode for approaching it and distinguishes between ill- and well-structured problems. Technological problems, which are often the nature of operational and optimization problems, comprise the characteristics of a well-structured problem with its transparent variables and are therefore suitably approached with standardized problem-solving methods (Mohaghegh and Furlan, 2020), as is the case in manufacturing industry. The complex interconnectivity of ill-structured problems, which most problems involving social aspects could be considered as, requires other problem-solving techniques and attitudes (Mohaghegh and Furlan, 2020) that may perhaps generate friction with established problem-solving culture of the manufacturing industry. And so, by maintaining the focus on operational and/or optimization problems, research on the subject stays familiar with the situational-experimental mode of solving problems. In other words, it can first be suggested that contemporary research on advanced technology implementation for problem-solving in the manufacturing industry holds a technological deterministic view, as there is a significant focus on well-structured operational/optimization problems and a quantitative, positivistic approach towards solving them.
Secondly, as mentioned, aggregated problem-solving literature roughly divides this process into three parts: finding, understanding and defining the problem; finding, understanding and evaluating potential solutions; and implementing the chosen solution (Basadur et al., 1982; Frensch and Funke, 1995; Mohaghegh and Furlan, 2020; Morgan, 2020; Newell and Simon, 1972; von Hippel and von Kroch, 2013). In accordance with comments by Sturm et al. (2021), the findings in the scoping review displayed in Table 1 show a clear focus on the solution phase. This too could be an effect of above discussed established problem-solving culture in the manufacturing industry (Mohaghegh and Größler, 2021; Womack and Jones, 1997), but could also have to do with what Mohaghegh and Furlan (2020) refer to as environmental dynamism as an impacting factor of problem-solving; the level of competition surrounding the hype narrative of advanced technology (Davenport and Ronanki, 2018; Jarrahi, 2018) could stress the process of implementing advanced technology for problem-solving purposes in manufacturing industry, forcing the process to go into the solution phase. This is in further accordance with findings by Shahlaei and Snis (2022), showing that manufacturing industry is somewhat coerced into change in times of technological advancement. The findings are also in accordance with results in the review by Joksimovic et al. (2023), stating that research on AI and problem-solving in the manufacturing industry lacks a focus on the implementation stage as well as with statements by Sturm et al. (2021), saying that research on advanced technology and problem-solving in general lacks a focus on the initial, conceptual phases of the problem-solving process. In other words, it is suggested that a combination of the problem-solving culture of manufacturing industry and external competitive forces that generally surround the advanced technology transformation force the field into a clear focus on the solution phase of the problem-solving process.
The second research question of this paper read: “How can contemporary research on advanced technology implementation for problem-solving in manufacturing industry be understood from a learning perspective?”. This question is answered building on the answer to the first question and the results of the scoping review in light of contradicting learning logics as described in the theory section.
As mentioned, the new paradigm of problem-solving practices that the introduction of advanced technology infers requires an integration of both new and old, but also of machines and humans (Jarrahi, 2018; Joksimovic et al., 2023; Singh et al., 2021; Von Krogh, 2018). Seen from a problem-solving perspective, this implies a change in the nature of the actual problems and, thus, a change in suitable and successful problem-solving approaches (Joksimovic et al., 2023). The scoping review findings display a clear focus on operational and/or optimization problems, meaning that they are most likely found, identified, and their potential solutions discussed based on existing knowledge and resources. In other words, it is proposed that the focus in these specific areas ensnares the research on advanced technology implementations for problem-solving in the manufacturing industry in what is in this study referred to as Mode-1 problem-solving and learning, engaging in trial-and-error short-term improvements with the aims of correcting errors, and potentially failing at contributing with sustainable solutions (Argyris and Schön, 1997; Mohaghegh and Größler, 2021; Watzlawick et al., 2011). In this particular area of context, sustainable is somewhat synonymous with socially and practically applicable in “real life”.
Furthermore, the clear focus on the solution phase of the problem-solving process found in the scoping review results adds another dimension to this issue. By engaging in Mode-1 problem-solving and learning while focusing on the solution phase, it is suggested that both the initial phase of finding, understanding, and defining the problem, as well as the actual implementation phase are colored by the exploitative mechanisms that characterize Mode-1. In other words, affected by predetermined assumptions and behaviours (March, 1991). Staying in this loop could contribute to the choice of problems to be focused on in terms of advanced technology implementations, as current knowledge is not challenged but used as a foundation for engaging in problem-solving (Argyris, 1976). By engaging in Mode-1 problem-solving and learning, manufacturing companies may restrict themselves to using the full potential of the new and advanced technology without gaining new knowledge about exploring innovative ways of adopting it. This statement is somewhat in accordance with findings by Shahlaei and Snis (2022), who say that technological change in the manufacturing industry tends to lack time and space for reflection – a key aspect for organizational learning.
To learn from problems, organizations must allow existing knowledge to be challenged and act on new assumptions (Argyris, 1976). As a countermeasure to the identified Mode-1 conditions on the subject, the argument here is that contemporary research on the subject, and in extension, the practical adoption of such results, should embrace the central ideas of Mode-2 problem-solving and learning in advanced technology implementations for problem-solving purposes. This infers taking the time to go to the problem, as advocated by Sturm et al. (2021), reflect on it properly (Shahlaei and Snis, 2022), test existing assumptions about it, expand the knowledge about it, and hence, expand the knowledge on potential solutions as well as choosing sustainable courses of action (Argyris and Schön, 1997; March, 1991; Mohaghegh and Furlan, 2020; Watzlawick et al., 2011). Although consciously adopting Mode-2 may result in excessive abstractedness (Liedtka, 2020) and a loss of using existing capabilities (Mohaghegh and Größler, 2021), its explorative nature may on the other hand contribute to the generation of new knowledge about potential use of advanced technology implementations for problem-solving. Further support for this argument could be found in some of the impacting factors of successful problem-solving as listed by Mohaghegh and Furlan (2020); engaging in Mode-2 problem-solving could enhance the time availability for solving the problems, increase the information availability of the problems, promote the collaborative culture surrounding the problems, and thus increase the possibilities for generating practically applicable and sustainable solutions. From a more long-term perspective, the idea of further adopting Mode-2 is in some ways supported by Shahlaei and Snis (2022) and Ivaldi et al. (2022), stating that manufacturing industries are moving from being production companies into being development companies, thus naturally more explorative in their nature.
To further support the transition of contemporary research on advanced technology implementation for problem-solving purposes in manufacturing industry, and in extension, the transition for manufacturing industry companies from engaging in Mode-1 into Mode-2 in terms of problem-solving and organizational learning, a research agenda with six propositions based on the findings of the scoping review and the discussion with contradicting learning logics of the findings is presented in Table 2 and elaborated on below.
P1 is to expand the methodological repertoire in the field to include empirically anchored and practical application studies. This is grounded in the scoping review findings showing a lack of empirical anchoring of tested algorithms and models (see Table 1), as well as the identified solution focus of the field. By including more empirically anchored studies, both the initial and implementation phases of problem-solving processes could be further understood, as advocated by Sturm et al. (2021) and Joksimovic et al. (2023). Further research on empirically anchored applications may also support manufacturing industries in understanding added value of advanced technology, as commented by Burggräf et al. (2021). Furthermore, additional empirical studies in the field are suggested to enhance the theoretical understanding of organizational learning on the subject, as learning could be studied and evaluated during implementation processes per se.
P2 is to include additional research paradigms and designs to allow for a perspective expansion on the subject. The scoping review findings display a positivistic tradition in which quantitative methods are explicitly used, which is useful for the technologically deterministic state of the subject, however, may risk neglecting others. This notion is in accordance with findings by Engström (2014), who commented that technological problems tend to gain more attention in organizational settings. By including research paradigms connected to social sciences and qualitative methods (e.g. interpretivism, pragmatism and action research), further theorization in the field could be possible, as advocated by Joksimovic et al. (2023). In addition, Ivaldi et al. (2022) comment that there is a knowledge gap related to circumstantial aspects of advanced technology introduction in terms of organizational impact. This gap could, proposedly, be approached by including additional research paradigms, scientific lines of thought, and social science-related methodologies, and, in extension, by allowing for additional knowledge on the relationship between organizational learning, advanced technology implementation and problem-solving.
P3 is to include additional research focusing solely on the initial and final phases of the problem-solving process, as depicted in Figure 1. As commented by Sturm et al. (2021), initial phases of the problem-solving process are clearly neglected in general problem-solving literature, as well as in the field of problem-solving with advanced technology. This change of focus could facilitate Mode-2 problem-solving and learning (as described by (Argyris and Schön, 1997; March, 1991; Mohaghegh and Furlan, 2020; Watzlawick et al., 2011) and thus allow for more exploratory approaches towards advanced technology implementations for problem-solving purposes. Such focus could also provide companies struggling with understanding the added value of advanced technology capabilities, as commented by Burggräf et al. (2021), as well as identify further potential by expanding current approaches, as advocated by Davenport and Ronanki (2018) and Kane (2019). Davenport and Ronanki (2018) further comment that the new and advanced technology now entering is more complex than before, and thus, it is suggested that established problem-solving approaches and research focus on the solution phase may hinder for expanding theoretical knowledge in the field of organizational learning, also in accordance with the notion by Joksimovic et al. (2023), commenting that a change in conditions surrounding a problem calls for new problem-solving approaches.
P4 infers studying other problems than operational and/or optimization problems. The findings imply that contemporary research on these types of problems forces the field into Mode-1 problem-solving and learning as the problems are most often of a well-structured nature (Mohaghegh and Furlan, 2020), and thus solvable with established methods. By including other problems, knowledge on ill-structured problems and, thus, a more holistic understanding of advanced technology possibilities for problem-solving purposes can, as proposed, be achieved. In extension, companies struggling with seeing the potential of advanced technology, as commented by Burggräf et al. (2021), are suggested to achieve deeper understanding of advanced technology capabilities and thus, understand its innovation value. Studying problems of more social nature may also open up for additional studies focusing on organizational learning in relation to problem-solving with advanced technology in the manufacturing industry.
P5 implies including more social and socio-technical aspects in the research field. As stated, advanced technology is expected to have a significant impact on both operational and strategical areas (Frank et al., 2019; Von Krogh, 2018), and to implement advanced technology is to open up new types of integration (Flores et al., 2020; Joksimovic et al., 2023; Singh et al., 2021). Since a challenge for companies in terms of advanced technology transformation is understanding its use and value as well as treating their problems as learning opportunities (Davenport and Ronanki, 2018; PWC, 2021), a shift in the field to include social and socio-technical perspectives on problem-solving in the manufacturing industry involving advanced technology is imperative. Furthermore, as commented by Mohaghegh and Furlan (2020), collaborative culture is identified as an important impacting factor for problem-solving success, while Ivaldi et al. (2022) comment that there is a knowledge gap related to organizational impact of technology implementations. It is thus finally suggested that a further social and socio-technical focus in the field would provide additional knowledge on advanced technology problem-solving from a more holistic point of view.
Finally, proposition 6 is to further evolve and study the terminology related to problems and problem-solving in the context of advanced technology implementations and in relation to organizational learning. As stated, the terms “problem” and “problem-solving” in relation to technology are often connected to mathematical (and thus, optimization) problems, which could be one of the reasons why the findings of this review point to technological determinism in the field. However, many social science scholars studying advanced technology in organizations refer to companies’ implementation challenges as not properly understanding the “problem” or not using “problem-solving” as learning opportunities (Davenport and Ronanki, 2018). The proposition is thus to evolve the terminology to include a more concrete and multidisciplinary understanding of the concepts and allow for a more inclusive arena to combine the subjects of problem-solving, organizational learning and advanced technology.
Conclusion
This paper shows that contemporary research on advanced technology implementations for problem-solving purposes in manufacturing industry finds itself in a technologically deterministic, solution-focused state. To further understand the relationship between advanced technology, problem-solving and organizational learning in the manufacturing industry, theories of contradicting learning logics were used to discuss the scoping review findings. The discussion suggests that the current state of contemporary research could be explained by contextual factors of manufacturing industry problem-solving culture, which forces the field into Mode-1 problem-solving and learning conditions. To fully use advanced technology’s potential and facilitate organizational learning surrounding the technology, it is suggested that a Mode-2 problem-solving and learning approach could be adopted. To further support this transition, a research agenda with six propositions based on the analysis is presented, providing suggested guidance for further research in the field. The mapping, analysis and discussion on the relationship between problem-solving, advanced technology and organizational learning are considered the main theoretical contribution of this paper, in addition to the research agenda. The paper is also considered to further provide practical contribution in the form of insights related to ongoing challenges of organizational learning in terms of implementing advanced technology for problem-solving purposes in manufacturing industry.
Limitations
The chosen terminology for this paper and the keyword search in the scoping review are considered highly influential on the findings, as the terms “problem” and “problem-solving” are commonly conceptually connected to operational tasks in an industry context. It is thus acknowledged that articles from the social sciences or those focusing on social issues could have been a part of the findings if database searches also included additional terminology. Some examples are “digitalization”, “digital transformation”, or “advanced technology application”. In contrast, articles with similar focuses in educational science and health care that were screened during the scoping review display more human involvement, pointing to the notion that the technological deterministic approach and thus the challenge with using the term “problem” could be a manufacturing industry contextual factor.
Figures
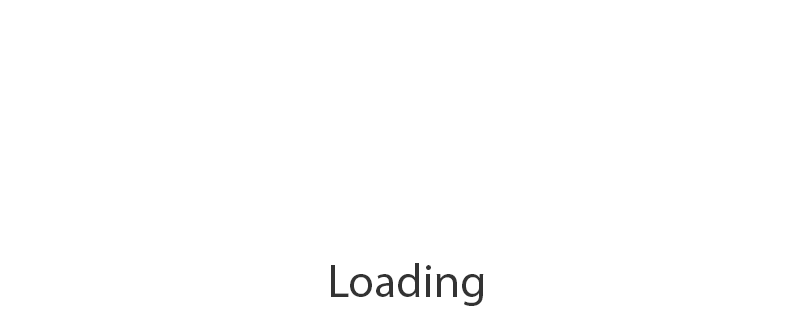
Figure 2.
Visualization of literature search and review procedure, inspired by PRISMA flow diagram (PRISMA, 2021)
Final selection article research demographics
Source: Authors’ own work
Proposed research agenda to expand knowledge on the subject
Methodology | Context | Perspective |
---|---|---|
1. Expand methodological repertoire to include empirically anchored and practical application studies 2. Additional research paradigms and designs to allow for perspective expansion on the subject |
3. Focus change to initial phase of problem-solving (i.e. problem-finding, problem-understanding, problem-definition) as well as to the solution implementation phase 4. Broaden the scope to additional problems to promote advanced technology innovation and organizational learning connected to technology |
5. Include social and socio-technical perspective in the field to promote practical use of advanced technology and increase organizational learning 6. Further develop the concepts of problems and problem-solving in the context of advanced technology transformation to facilitate further research on the subject |
Source: Authors’ own work
References
Abdirad, M., Krishnan, K. and Gupta, D. (2021), “A two-stage metaheuristic algorithm for the dynamic vehicle routing problem in industry 4.0 approach”, Journal of Management Analytics, Vol. 8 No. 1, pp. 69-83.
Agrawal, P., Alnowibet, K., Ganesh, T., Alrasheedi, A.F., Ahmad, H. and Mohamed, A.W. (2022), “An artificial intelligence approach for solving stochastic transportation problems”, Computers, Materials and Continua, Vol. 70 No. 1, pp. 817-829.
Argyris, C. (1976), “Single-loop and double-loop models in research on decision making”, Administrative Science Quarterly, Vol. 21 No. 3, pp. 363-375.
Argyris, C. and Schon, D. (1978), Organizational Learning: A Theory of Action Perspective, Addison Wesley, Reading, Mass.
Argyris, C. and Schön, D.A. (1997), “Organizational learning: a theory of action perspective”, Reis, Vols 77/78, pp. 345-348.
Aylak, B.L., Ince, M., Oral, O., Süer, G., Almasarwah, N., Singh, M. and Salah, B. (2021), “Application of machine learning methods for pallet loading problem”, Applied Sciences, Vol. 11 No. 18.
Basadur, M., Graen, G.B. and Green, S.G. (1982), “Training in creative problem solving: effects on ideation and problem finding and solving in an industrial research organization”, Organizational Behavior and Human Performance, Vol. 30 No. 1, pp. 41-70.
Benda, F., Braune, R., Doerner, K.F. and Hartl, R.F. (2019), “A machine learning approach for flow shop scheduling problems with alternative resources, sequence-dependent setup times, and blocking”, Or Spectrum, Vol. 41 No. 4, pp. 871-893.
Bergès, C., Bird, J., Shroff, M.D., Rongen, R. and Smith, C. (2021), “Data analytics and machine learning: root-cause problem-solving approach to prevent yield loss and quality issues in semiconductor industry for automotive applications”, 2021 IEEE International Symposium on the Physical and Failure Analysis of Integrated Circuits (IPFA), pp. 1-10, IEEE.
Booth, A., Sutton, A. and Papaioannou, D. (2012), Systematic Approaches to a Successful Literature Review, SAGE Publications Ltd, London.
Burggräf, P., Wagner, J. and Heinbach, B. (2021), “Bibliometric study on the use of machine learning as resolution technique for facility layout problems”, IEEE Access, Vol. 9, pp. 22569-22586.
Chen, Y., Zhou, Y. and Zhang, Y. (2021), “Machine learning-based model predictive control for collaborative production planning problem with unknown information”, Electronics, Vol. 10 No. 15, p. 1818.
Davenport, T.H. and Ronanki, R. (2018), “Artificial intelligence for the real world”, Harvard Business Review, Vol. 96 No. 1, pp. 108-116.
de Jager, C. and Nel, M. (2019), “Business process automation: a workflow incorporating optical character recognition and approximate string and pattern matching for solving practical industry problems”, Applied System Innovation, Vol. 2 No. 4, p. 33.
Dias, L.S. and Ierapetritou, M.G. (2019), “Data-driven feasibility analysis for the integration of planning and scheduling problems”, Optimization and Engineering, Vol. 20 No. 4, pp. 1029-1066.
Duc, D.N. and Nananukul, N. (2020), “A hybrid methodology based on machine learning for a supply chain optimization problem”, paper presented at the Journal of Physics: Conference Series, 2020, available at: www.researchgate.net/publication/345543941_A_Hybrid_Methodology_Based_on_Machine_Learning_for_a_Supply_Chain_Optimization_Problem (accessed 3 July 2022).
Dunbar, K. and Fugelsang, J. (2005), “Scientific thinking and reasoning”, The Cambridge Handbook of Thinking and Reasoning, Cambridge University Press, Cambridge, pp. 705-725.
D'zurilla, T.J. and Goldfried, M.R. (1971), “Problem solving and behavior modification”, Journal of Abnormal Psychology, Vol. 78 No. 1, p. 107.
Edmondson, A.C. (2018), The Fearless Organization: Creating Psychological Safety in the Workplace for Learning, Innovation, and Growth, John Wiley and Sons, Hoboken, NJ.
Engström, A. (2014), Lärande Samspel För Effektivitet: En Studie Av Arbetsgrupper i Ett Mindre Industriföretag, Linköping University Electronic Press, Linköping.
Fang, W., Guan, Z., Luo, D., He, C., Wang, H. and Wang, C. (2019), “Research on automatic flow-shop planning problem based on data driven modelling simulation and optimization”, Paper presented at the IOP Conference Series: Materials Science and Engineering 2019, available at: www.researchgate.net/publication/334044472_Research_on_Automatic_Flow-shop_Planning_Problem_Based_on_Data_Driven_Modelling_Simulation_and_Optimization (accessed 3 July 2022).
Ferraro, F., Etzion, D. and Gehman, J. (2015), “Tackling grand challenges pragmatically: robust action revisited”, Organization Studies, Vol. 36 No. 3, pp. 363-390.
Flores, E., Xu, X. and Lu, Y. (2020), “Human capital 4.0: a workforce competence typology for industry 4.0”, Journal of Manufacturing Technology Management, Vol. 31 No. 4, pp. 687-703.
Fountaine, T., McCarthy, B. and Saleh, T. (2019), “Building the AI-powered organization”, Harvard Business Review, Vol. 97 No. 4, pp. 62-73.
Francalanza, E., Borg, J. and Constantinescu, C. (2018), “Approaches for handling wicked manufacturing system design problems”, Procedia CIRP, Vol. 67, pp. 134-139.
Frank, A.G., Dalenogare, L.S. and Ayala, N.F. (2019), “Industry 4.0 technologies: implementation patterns in manufacturing companies”, International Journal of Production Economics, Vol. 210, pp. 15-26.
Frensch, P.A. and Funke, J. (1995), “Definitions, traditions, and a general framework for understanding complex problem solving”, in Frensch, P.A. and Funke, J. (Eds), Complex Problem Solving: The European Perspective, Lawrence Erlbaum Associates, Hillsdale, New Jersey, pp. 4-25.
Funke, J. (2013), “Human problem solving in 2012”, The Journal of Problem Solving, Vol. 6 No. 1, p. 3.
Garbaya, A., Kallel, I., Fakhfakh, M. and Siarry, P. (2022), “Machine learning techniques for solving constrained engineering problems”, Paper presented at the 2022 2nd International Conference on Innovative Research in Applied Science, Engineering and Technology (IRASET) Meknes, Morocco, 2022, available at: https://ieeexplore.ieee.org/document/9738011 (accessed 4 July 2022).
Garud, R. (2020), “Microfoundations of innovation as process: Usher’s cumulative synthesis model”, in Poole, M.S. and Van de Ven, A.H. (Eds), Oxford Handbook of Organization Change and Innovation, Oxford University Press, Oxford.
Guo, M. and Hesthaven, J.S. (2019), “Data-driven reduced order modeling for time-dependent problems”, Computer Methods in Applied Mechanics and Engineering, Vol. 345, pp. 75-99.
Han, D., Li, W., Li, X., Gao, L. and Li, Y. (2021), “A data-driven proactive scheduling approach for hybrid flow shop scheduling problem”, Paper presented at the International Manufacturing Science and Engineering Conference, available at: https://asmedigitalcollection.asme.org/MSEC/proceedings-abstract/MSEC2021/85079/V002T07A002/1115435 (accessed 4 July 2022).
Hellas, M.S., Chaib, R. and Verzea, I. (2019), “Artificial intelligence treating the problem of uncertainty in quantitative risk analysis (QRA)”, Journal of Engineering, Design and Technology, Vol. 18 No. 1, pp. 40-54.
Hobscheidt, D., Kühn, A. and Dumitrescu, R. (2021), “Derivation of socio-technical solution patterns for industry 4.0 problem classes”, Procedia Cirp, Vol. 100, pp. 301-306.
Huu, T.T., Duc, D.N. and Nananukul, N. (2020), “Machine learning model for a dynamic path planning problem”, Paper presented at the Journal of Physics: Conference Series, available at: www.researchgate.net/publication/345543949_Machine_Learning_Model_for_a_Dynamic_Path_Planning_Problem (accessed 4 July 2022).
Islam, J., Vasant, P.M., Negash, B.M., Laruccia, M.B., Myint, M. and Watada, J. (2020), “A holistic review on artificial intelligence techniques for well placement optimization problem”, Advances in Engineering Software, Vol. 141, p. 102767.
Ivaldi, S., Scaratti, G. and Fregnan, E. (2022), “Dwelling within the fourth industrial revolution: organizational learning for new competences, processes and work cultures”, Journal of Workplace Learning, Vol. 34 No. 1, pp. 1-26.
Jarrahi, M.H. (2018), “Artificial intelligence and the future of work: human-AI symbiosis in organizational decision making”, Business Horizons, Vol. 61 No. 4, pp. 577-586.
Jhaver, M., Gupta, Y. and Mishra, A.K. (2019), “Employee turnover prediction system”, Paper presented at the 2019 4th International Conference on Information Systems and Computer Networks (ISCON), available at: https://ieeexplore.ieee.org/document/9036180 (accessed 3 July 2022).
Joksimovic, S., Ifenthaler, D., Marrone, R., De Laat, M. and Siemens, G. (2023), “Opportunities of artificial intelligence for supporting complex problem-solving: findings from a scoping review”, Computers and Education: Artificial Intelligence, Vol. 4, p. 100138.
Kane, G. (2019), “The technology fallacy: people are the real key to digital transformation”, Research-Technology Management, Vol. 62 No. 6, pp. 44-49.
Leusin, M.E., Frazzon, E.M., Uriona Maldonado, M., Kück, M. and Freitag, M. (2018), “Solving the job-shop scheduling problem in the industry 4.0 era”, Technologies, Vol. 6 No. 4, p. 107.
Liedtka, J. (2020), “Putting technology in its place: design thinking’s social technology at work”, California Management Review, Vol. 62 No. 2, pp. 53-83.
Lin, S., Chen, Y., Li, Y. and Shen, Z.J.M. (2022), “Data‐driven newsvendor problems regularized by a profit risk constraint”, Production and Operations Management, Vol. 31 No. 4, pp. 1630-1644.
Makarius, E.E., Mukherjee, D., Fox, J.D. and Fox, A.K. (2020), “Rising with the machines: a sociotechnical framework for bringing artificial intelligence into the organization”, Journal of Business Research, Vol. 120, pp. 262-273.
March, J.G. (1991), “Exploration and exploitation in organizational learning”, Organization Science, Vol. 2 No. 1, pp. 71-87.
Matsuoka, Y., Nishi, T. and Tiemey, K. (2019), “Machine learning approach for identification of objective function in production scheduling problems”, Paper presented at the 2019 IEEE 15th international conference on automation science and engineering (CASE), available at: https://ieeexplore.ieee.org/document/8843054 (accessed 5 July 2022).
Mohaghegh, M. and Furlan, A. (2020), “Systematic problem-solving and its antecedents: a synthesis of the literature”, Management Research Review, Vol. 43 No. 9, pp. 1033-1062.
Mohaghegh, M. and Größler, A. (2021), “Exploring organizational problem-solving modes: a dynamic capabilities approach”, Management Decision, Vol. 60 No. 1, pp. 254-277.
Moretti, E., Tappia, E., Limère, V. and Melacini, M. (2021), “Exploring the application of machine learning to the assembly line feeding problem”, Operations Management Research, Vol. 14 Nos 3/4, pp. 403-419.
Morgan, D.L. (2020), “Pragmatism as a basis for grounded theory”, The Qualitative Report, Vol. 25 No. 1, p. 64.
Müller, D., Müller, M.G., Kress, D. and Pesch, E. (2022), “An algorithm selection approach for the flexible job shop scheduling problem: choosing constraint programming solvers through machine learning”, European Journal of Operational Research, Vol. 302 No. 3, pp. 874-891.
Munn, Z., Peters, M.D., Stern, C., Tufanaru, C., McArthur, A. and Aromataris, E. (2018), “Systematic review or scoping review? Guidance for authors when choosing between a systematic or scoping review approach”, BMC Medical Research Methodology, Vol. 18 No. 1, pp. 1-7.
Nahhas, A., Lang, S., Bosse, S. and Turowski, K. (2018), “Toward adaptive manufacturing: scheduling problems in the context of industry 4.0”, Paper presented at the 2018 Sixth International Conference on Enterprise Systems (ES), available at: www.researchgate.net/publication/328364857_Toward_Adaptive_Manufacturing_Scheduling_Problems_in_the_Context_of_Industry_40 (accessed 3 July 2022).
Newell, A. and Simon, H.A. (1972), Human Problem Solving, Prentice-hall Englewood Cliffs, NJ, Vol. 104.
Nie, L., Wang, X., Bai, Y. and Wu, X. (2019), “Machine learning approach based on gene expression programming for dynamic production scheduling problem”, Paper presented at the IOP conference series: materials science and engineering, available at: https://iopscience.iop.org/article/10.1088/1757-899X/612/3/032169/pdf (accessed 3 July 2022).
Pisacane, O., Potena, D., Antomarioni, S., Bevilacqua, M., Emanuele Ciarapica, F. and Diamantini, C. (2021), “Data-driven predictive maintenance policy based on multi-objective optimization approaches for the component repairing problem”, Engineering Optimization, Vol. 53 No. 10, pp. 1752-1771.
Polya, G. (2004), How to Solve It: A New Aspect of Mathematical Method, Princeton university press, Princeton.
PRISMA (2021), “PRISMA flow diagram”, available at: https://prisma-statement.org/PRISMAStatement/FlowDiagram (accessed 5 August 2022).
Pwc, H. (2021), “Operationalizing artificial intelligence: making the promise a reality”, Research Report, Harvard Business Review, available at: https://hbr.org/sponsored/2021/10/operationalizing-artificial-intelligence-making-the-promise-a-reality (accessed 3 July 2022).
Scheerer, M. (1963), “Problem-solving”, Scientific American, Vol. 208 No. 4, pp. 118-131.
Schon, D.A. (1984), The Reflective Practitioner: How Professionals Think in Action, Routledge, London.
Shahlaei, C.A. and Snis, U.L. (2022), “Conceptualizing industrial workplace learning: an information systems perspective”, Journal of Workplace Learning, Vol. 35 No. 9, pp. 1-21.
Singh, A., Wiktorsson, M. and Hauge, J.B. (2021), “Trends in machine learning To solve problems In logistics”, Procedia CIRP, Vol. 103, pp. 67-72.
Sjödin, D., Parida, V., Palmié, M. and Wincent, J. (2021), “How AI capabilities enable business model innovation: scaling AI through co-evolutionary processes and feedback loops”, Journal of Business Research, Vol. 134, pp. 574-587.
Sturm, T., Fecho, M. and Buxmann, P. (2021), “To use or not to use artificial intelligence? A framework for the ideation and evaluation of problems to be solved with artificial intelligence”, Paper presented at the Proceedings of the 54th Hawaii International Conference on System Sciences, available at: www.researchgate.net/publication/348048339_To_Use_or_Not_to_Use_Artificial_Intelligence_A_Framework_for_the_Ideation_and_Evaluation_of_Problems_to_Be_Solved_with_Artificial_Intelligence (accessed 4 May 2023).
Sun, Y., Ernst, A., Li, X. and Weiner, J. (2021), “Generalization of machine learning for problem reduction: a case study on travelling salesman problems”, Or Spectrum, Vol. 43 No. 3, pp. 607-633.
Thorndike, E.L. (1898), “Animal intelligence: an experimental study of the associative processes in animals”, The Psychological Review: Monograph Supplements, Vol. 2 No. 4.
van der Laan, N., Teunter, R.H., Romeijnders, W. and Kilic, O.A. (2022), “The data-driven newsvendor problem: achieving on-target service-levels using distributionally robust chance-constrained optimization”, International Journal of Production Economics, Vol. 249, p. 108509.
Vithitsoontorn, C. and Chongstitvatana, P. (2022), “Demand forecasting in production planning for dairy products using machine learning and statistical method”, Paper presented at the 2022 International Electrical Engineering Congress (iEECON), available at: https://ieeexplore.ieee.org/document/9741683 (accessed 5 August 2022).
von Hippel, E. and von Kroch, G. (2013), “Identifying viable ‘need-solution pairs’: problem solving without problem formulation”, Organization Science, Vol. 27 No. 1, pp. 207-221.
Von Krogh, G. (2018), “Artificial intelligence in organizations: new opportunities for phenomenon-based theorizing”, Academy of Management Discoveries, Vol. 4 No. 4, pp. 4040-4409.
Watzlawick, P., Weakland, J.H. and Fisch, R. (2011), Change: Principles of Problem Formation and Problem Resolution, WW Norton and Company, New York, NY.
Westenberger, J., Schuler, K. and Schlegel, D. (2022), “Failure of AI projects: understanding the critical factors”, Procedia Computer Science, Vol. 196, pp. 69-76.
Womack, J.P. and Jones, D.T. (1997), “Lean thinking – banish waste and create wealth in your corporation”, Journal of the Operational Research Society, Vol. 48 No. 11, pp. 1148-1148.
Woods, D.R., Hrymak, A.N., Marshall, R.R., Wood, P.E., Crowe, C.M., Hoffman, T.W. and Bouchard, C.K. (1997), “Developing problem solving skills: the McMaster problem solving program”, Journal of Engineering Education, Vol. 86 No. 2, pp. 75-91.
Xu, Z. and Dang, Y. (2020), “Automated digital cause-and-effect diagrams to assist causal analysis in problem-solving: a data-driven approach2”, International Journal of Production Research, Vol. 58 No. 17, pp. 5359-5379.
Zhang, J., Yu, M., Feng, Q., Leng, L. and Zhao, Y. (2021), “Data-driven robust optimization for solving the heterogeneous vehicle routing problem with customer demand uncertainty”, Complexity, Vol. 2021, pp. 1-19.
Zhang, C., Zhang, D. and Wu, T. (2021), “Data-driven branching and selection for lot-sizing and scheduling problems with sequence-dependent setups and setup carryover”, Computers and Operations Research, Vol. 132, p. 105289.
Zheng, T., Ardolino, M., Bacchetti, A. and Perona, M. (2021), “The applications of industry 4.0 technologies in manufacturing context: a systematic literature review”, International Journal of Production Research, Vol. 59 No. 6, pp. 1922-1954.
Acknowledgements
The author acknowledge the Knowledge Foundation, Jönköping University, and the industrial partners for financially supporting the research and education environment on Knowledge Intensive Product Realization SPARK at Jönköping University, Sweden. Project: AFAIR with agreement number 20200223.