Abstract
Purpose
The purpose of this paper is to investigate the influence of driver demographic characteristics on the driving safety involving cell phone usages.
Design/methodology/approach
A total of 1,432 crashes and 19,714 baselines were collected for the Strategic Highway Research Program 2 naturalistic driving research. The authors used a case-control approach to estimate the prevalence and the population attributable risk percentage. The mixed logistic regression model is used to evaluate the correlation between different driver demographic characteristics (age, driving experience or their combination) and the crash risk regarding cell phone engagements, as well as the correlation among the likelihood of the cell phone engagement during the driving, multiple driver demographic characteristics (gender, age and driving experience) and environment conditions.
Findings
Senior drivers face an extremely high crash risk when distracted by cell phone during driving, but they are not involved in crashes at a large scale. On the contrary, cell phone usages account for a far larger percentage of total crashes for young drivers. Similarly, experienced drivers and experienced-middle-aged drivers seem less likely to be impacted by the cell phone while driving, and cell phone engagements are attributed to a lower percentage of total crashes for them. Furthermore, experienced, senior or male drivers are less likely to engage in cell phone-related secondary tasks while driving.
Originality/value
The results provide support to guide countermeasures and vehicle design.
Keywords
Citation
Cao, H., Zhang, Z., Song, X., Wang, H., Li, M., Zhao, S. and Wang, J. (2020), "An investigation on the link between driver demographic characteristics and distracted driving by using the SHRP 2 naturalistic driving data", Journal of Intelligent and Connected Vehicles, Vol. 3 No. 1, pp. 1-16. https://doi.org/10.1108/JICV-10-2019-0012
Publisher
:Emerald Publishing Limited
Copyright © 2020, Haotian Cao, Zhenghao Zhang, Xiaolin Song, Hong Wang, Mingjun Li, Song Zhao and Jianqiang Wang.
License
Published in Journal of Intelligent and Connected Vehicles. Published by Emerald Publishing Limited. This article is published under the Creative Commons Attribution (CC BY 4.0) licence. Anyone may reproduce, distribute, translate and create derivative works of this article (for both commercial and non-commercial purposes), subject to full attribution to the original publication and authors. The full terms of this licence may be seen at http://creativecommons.org/licences/by/4.0/legalcode
1. Introduction
National Highway Traffic Safety Administration (NHTSA) reported that 3,450 people in the USA were killed in distraction-related crashes in 2016, and 444 (14 per cent) fatalities occurred in crashes with at least one of the drivers involved in cell phone usages at the time of the crash. Consequently, using cell phones while driving increases the risk of crash fatalities and injuries and continues to be a widely concerned issue related to public safety. Moreover, NHTSA reported that 13 per cent of drivers distracted by cell phones in fatal crashes were aged 15-19 years, while drivers aged 20-29 represented 35 per cent of fatalities (National Highway Traffic Safety Administration, 2018). Thus, from the point of improving driving safety, it is worthwhile to investigate the relationship between the risk posed by using cell phones while driving and driver demographic characteristics.
According to a report in the USA, 542,000 drivers of cars were observed talking on cell phones during one day, equating to 3.8 per cent of American drivers (Pickrell et al., 2016), using cell phones while driving is rather common. The Harris Group’s poll (Harris Interactive, 2011) of representative samples of the adult American population showed that despite 47 per cent of respondents believing that using cell phone while driving was “very risky”, nearly 60 per cent of the group still engaged in cell phone usage. Meanwhile, it is important to know how often drivers are likely to engage in cell phone-related tasks while driving and which kind of group of drivers was more likely to be affected by cell phone activities, such that policymakers might get benefits from the results and more effective countermeasures could be developed. What is more, many investigations have indicated that using cell phones while driving negatively affects various aspects of driving performances, such as lane-keeping (McKeever et al., 2013; Rudin-Brown et al., 2013), speed selection (Metz et al., 2015; Reimer et al., 2014; Tractinsky et al., 2013), car-following distance (Caird et al., 2014; He et al., 2014; Oviedo-Trespalacios et al., 2017), visual scanning (Caird et al., 2014; Collet et al., 2010; Oviedo-Trespalacios et al., 2017), response time in avoiding road hazards (Caird et al., 2018; Lipovac et al., 2017;Oviedo-Trespalacios et al., 2017), and even the risk of crashing (Dingus et al., 2016; Simmons et al., 2016; Simons-Morton et al., 2014). It was also found that using a cell phone while driving was likely to be influenced by driver demographic characteristics such as drivers’ age and driving experience, but not by gender. For instance, Zhao et al. (2013) demonstrated that gender was not a significant factor in mobile phone distracted driving, based on a quasi-naturalistic study of 108 participants. Guo et al. (2017) also found that driver’s gender did not significantly affect the safety of driving. Additionally, it was found that older drivers had worse driving performance compared to young drivers when engaged in dual-tasking (Asbridge et al., 2013; Chaparro et al., 2005). Guo et al. (2017) are based on a massive naturalistic driving data set to point out that the influence of secondary tasks on driving safety varied a lot because of the driver’s age and the nature of tasks. The study concluded that teenagers and young adults bare a higher risk than medium-aged drivers when undertaking one secondary task while driving. As for the driving experience, Tractinsky et al. (2013) found that younger drivers were more influenced by phone conversations than middle-aged or experienced drivers. Moreover, Klauer et al. (2014) also identified that the risk of crashing because of secondary-task engagement for novice drivers was higher than for experienced drivers. Choudhary and Velaga (2019) showed that young drivers manage to compensate for less steering reversals and show higher variations in lane positioning compared to professional drivers.
Nevertheless, we noticed that the most previous research was mainly focusing on the individual level (e.g. using odds ratio [OR]) (Dingus et al., 2016; Klauer et al., 2014). Considering OR can only describe the odds that a crash will occur given a particular exposure and compared to the odds of the crash occurring in the absence of that exposure, while the crash risk difference between different groups cannot be directly presented. What is more, the risk level of one secondary task engagement among the whole population is unclear while only have ORs reported. Other limitations of previous studies might include small sample size (Chaparro et al., 2005; Merat et al., 2005; Tractinsky et al., 2013), which the occurrence rate on a population basis was not considered, thereby missing the opportunity for a quantitative description of the driving risk for a particular population. While some of the studies only investigated in a single factor effect (e.g. age or driving experience) on driving performance involved with distractions (Guo et al., 2017; Kass et al., 2007; Klauer et al., 2014). Though there were some studies investigating the prevalence of distracting related behaviors by roadside observation. However, considering the roadside observation, a stationary observer simply records the activities and demographic characteristics (e.g. gender, age) of drivers as they pass a selected location (Ranney, 2008). Roadside observational studies did not include a range of traffic and roadway conditions that occur during the driving. As a result, observing sites were often limited, leading to an inaccurate assessment of the prevalence of distractions (Johnson et al., 2004; Sullman, 2012). On the contrary, naturalistic observation assesses the driving behavior of drivers over a period of time using instrumented vehicles. Thus, naturalistic data were collected over an extended period and represent normal, daily driving that occurs in a metropolitan environment, and the sample sizes were large enough. Consequently, the prevalence estimates would be more reasonable. Therefore, this study is based on a massive naturalistic driving database – the Strategic Highway Research Program 2 (SHRP 2) – which aims to evaluate the correlation between different driver demographic characteristics (age, driving experience or their combinations) and the crash risk regarding cell phone engagements, as well as the correlation among the likelihood of the cell phone engagement, multiple driver demographic characteristics (gender, age and driving experience) and environment conditions by using the mixed logistic regression model. We also use the population attributable risk (PAR) percentage and the prevalence (Pe) to quantitatively investigate the crash risk because of cell phone engagements during the driving.
2. Materials and methods
The SHRP 2 Naturalistic Driving Study, which is the largest naturalistic driving-behavior study to date, monitored over 3,500 participants and produced 2PB of their day-to-day naturalistic driving data from 2010 to 2013. The data were collected from six sites in the USA: Seattle, WA; Tampa, FL; Buffalo, NY; Durham, NC; State College, PA; and Bloomington, IN. The naturalistic driving data were obtained using the data acquisition system (DAS) from key-on to key-off for every trip. The onboard DAS collected four video messages (i.e. driver’s face, driver’s hand, front road and rear road), vehicle network information (i.e. speed, brake and accelerator position) and additional sensors from the DAS (including global positioning system, forward radar and accelerometer). The Technical Coordination and Quality Control were conducted by the Virginia Tech Transportation Institute at Virginia Polytechnic Institute and State University (Dingus et al., 2015). One way to provide researchers with access to SHRP 2 data is the SHRP 2 Insight website (https://insight.shrp2nds.us/), which allows researchers outside of secure facilities to browse de-identified driving data and build queries to search what they are interested in.
Participants comprised approximately half female (1,818) and half male (1,662) drivers. Their ages ranged from 16 to 98 and were categorized as young drivers (16-24 years old), middle-aged drivers (25-59 years old) and senior drivers (>60 years old) in this study. Additionally, drivers were divided into two groups based on their driving experience, namely, less-experienced drivers (≤10 years) and experienced drivers (>10 years). Instead of gathering data using a questionnaire after the accident, the SHRP 2 driving study directly records the driver’s behavior and secondary task engagements before a crash occurred. According to Dingus et al. (2015), more than 1,500 possible crash events were identified in the data set through the automatic crash notification algorithms on the DAS. If the vehicle status parameter was greater than the threshold value (e.g. breaking at more than 65 gravitational units), it was identified as a crash event. Once the event was identified, it was verified via video reviews. The definition of a crash was any contact between the vehicle and another object. Crash severities were defined as one of the following four levels as shown in Table I.
A case-cohort approach was used to evaluate the crash risk caused by secondary task engagements. The controls were short segments of non-safety critical events and comprised normal driving episodes, which were used to represent the exposure of risk factors under normal driving conditions. That is, random sampling control segments, which are composed of 6-s periods, a comparable length of time for determining the exposure to one secondary-task engagement for crashes, was used to represent normal driving conditions when the vehicle velocity was larger than 5 mph (8.05 km/h). For each driver, the number of control segments was directly proportionate to the number of miles or the number of driving hours. A secondary task was coded if it occurred during the control segment. Thus, the random sampling control segments also provided an opportunity to calculate the prevalence of one secondary task (Guo and Hankey, 2009). What is more, considering the research purpose of this study, only secondary tasks associated with cell phone usages, which includes seven specific subtasks, as shown in Table II, are analyzed. Finally, a data set comprising 21,146 epochs was collected from 3,302 vehicles with 3,481 drivers in 23,697 trips, which were recorded in the SHRP 2 database. Table III shows the numbers of crashes and baseline epochs in which each behavior was present and in which each was absent.
A mixed effect logistic regression model with a driver-specific random effect was used to examine the influence of driver demographic characteristics (age, driving experience or their combinations) on the driving safety consequence because of cell phone usages. Moreover, another similar mixed effect logistic regression model was performed to examine whether driver demographic characteristics (gender, age and driving experience) and environment condition variables (weather, light, traffic density and time of the day) were correlated with the likelihood of the cell phone engagement during the driving. If the interaction effect is significant at 0.05 level, then the estimated marginal mean (EMM) of the interaction effect respecting to the crash risk or the likelihood of the cell phone engagement would be obtained by using the professional statistical software IBM SPSS Statistics©. We also use the PAR percentage to describe the percentage of one group’s total risk which is in excess of the risk among persons not exposed to the suspect factor (Cole and Macmahon, 1971). In our case, it provides an assessment of the percentage of crashes occurring in the population at-large that are directly attributable to the specific behavior measured. Please follow Appendix to get detailed descriptions of statistical methods used in this study, which includes the regression modeling and the calculation of the PAR percentage.
3. Result analysis
3.1 Analysis of crash risk
According to mixed regression model results, differences of age (P < 0.05), driving experience (P < 0.05), as well as the combination of the age and driving experience (P < 0.05) are statistically significant for the crash risk when regarding the overall cell phone use in driving, but not for gender. What is more, Tables IV and V, as well as depicted in Figures 1, 2 and 3, further show the PAR percentage (with corresponding 95 per cent confidence intervals) and prevalence of each cell phone-related secondary task by the age group, driving experience group or their combinations.
As depicted in Figure 4(a), EMMs of the crash risk associated with the overall cell phone use is 12.80 per cent for the young, 6.60 per cent for the middle-aged and 14.30 per cent for the senior, as illustrated in Figure 2(a). The results indicate that, compared with middle-aged drivers, young drivers would confront higher crash risks when they are involved in any cell phone-related engagements while driving, and their difference of the EMM of crash risk is 6.20 per cent (P < 0.001). What is more, senior drivers might face higher crash risks than middle-aged drivers when engaging in cell phones, as their corresponding difference of the EMM of crash risk is 7.70 per cent, which is marginal (P = 0.06), while no significant difference is reported between the young and the senior. In addition, according to Table AI, ORs were extremely high for most specific activities related to the cell phone for senior drivers, such as cell phone texting, holding and dialing handheld. Nevertheless, point estimates of the PAR percentage showed in Table IV, as well as in Figure 1, indicate that the overall cell phone usage only accounts for a smaller percentage of total crashes for senior drivers (PAR per cent 1.86) or middle-aged drivers (PAR per cent 1.39) when compared with that of young drivers (PAR per cent 7.73).
As shown in Figure 4(a), EMMs of the crash risk regarding the overall cell phone use for less-experienced and experienced drivers are 12.00 and 7.20 per cent, respectively. Compared with experienced drivers, less-experienced drivers would confront higher crash risks when they were distracted by cell phones, the difference of the EMM of crash risk is 4.80 per cent (P <* 0.01). Furthermore, as shown in Table IV and Figure 2, it is interesting to observe that the point estimate of PAR percentage regarding the overall cell phone use for experienced drivers (PAR per cent 0.93) is much lower than that of less-experienced drivers (PAR per cent 7.07), as well as for each subtask. That indicates cell phone use contributes to a much lower percentage of total crashes for experienced drivers when compared with less-experienced drivers.
To investigate whether the combined effect of the age and driving experience regarding the safety impact would be significantly associated with the cell phone, drivers who are middle-aged, as well as have over 10 years’ driving experience are further categorized as one group, while rest of drivers are treated as the other one. And we found that the interaction effect of the combination of the age and driving experience is statistically significant (P < 0.05) with the overall cell phone use, cell phone visual-manual tasks, as well as subtasks including the cell phone holding and cell phone texting when regarding the crash risk. As depicted in Figure 4(a); EMMs of the crash risk for those two groups are 12.10 and 6.20 per cent. More specifically, experienced-middle-aged drivers engaging in any cell phone-related tasks would confront lower crash risks than other drivers; the difference of the EMM regarding the crash risk is 5.90 per cent (P <* 0.001). In addition, as shown in Table IV and Figure 3, the point estimate of PAR percentage regarding the overall cell phone use for experienced-middle-aged drivers is much lower than that of other drivers (PAR per cent: 0.87 vs 6.15), which indicates the percentage of all crashes that is attributable to cell phone usages for experienced-middle-aged drivers is much lower than to other drivers.
What is more, there are some other interesting findings regarding cell phone use, which are listed as follows:
The interaction effect between cell phone visual-manual demanded tasks and age groups is statistically significant for safety impacts (P < 0.05). As illustrated in Figure 4(b), EMMs of the crash risk regarding cell phone visual-manual tasks for three age groups are 16.70, 8.40 and 27.30 per cent. Young and senior drivers confront higher crash risks than middle-aged drivers when engaging in cell phone visual-manual related tasks. Their differences in the EMM of crash risk for young and middle-aged drivers, senior and middle-aged drivers are 8.30 per cent (P <0.01) and 18.90 per cent (P<0.05), respectively, while the difference between the young and the senior does not differ much. Further, experienced-middle-aged drivers engaged in cell phone visual-manual demand tasks would also face lower crash risks than other drivers; the difference of the EMM regarding the crash risk is 9.90 per cent (16.70 vs 6.80 per cent, P <0.001). And point estimates of PAR percentages regarding this task for experienced-middle-aged drivers are also higher than those of other drivers (PAR per cent: 0.59 vs 4.25).
The interaction effect regarding the crash risk between the cell phone holding and age is statistically significant (P < 0.05). More specifically, young and senior drivers are more adversely impacted by holding a cell phone than middle-aged drivers, as shown in Figure 4(c); EMMs of the crash risk for three age groups are 21.10, 10.20 and 36.40 per cent. Differences of the EMM between the young and the middle-aged is 11.00 per cent (P<0.01), and that between the senior and the middle-aged is 26.20 per cent (P < 0.05); however, no significant difference between the young and the senior is reported. In addition, the PAR percentage of cell phone holding is the largest among all cell phone-related engagements for each group. The point estimate of the PAR percentage varies among 2.19-6.74, especially the cell phone holding contributes to a relatively large percentage of total crashes for some groups (e.g. 6.74 of young drivers and 6.08 of less-experienced drivers). Thus, holding a cell phone needs to be paid more attention to their daily driving.
As depicted in Figure 4(c), middle-aged-experienced drivers are easier to be impacted by cell phone holding and cell phone texting, differences of the EMM regarding the crash likelihood are 8.90 per cent (20.10 vs 11.20 per cent, P < 0.05) and 10.30 per cent (16.70 vs 6.40 per cent, P < 0.01). Compared with experienced-middle-aged drivers, point estimates of PAR percentages regarding these two types of distractions account for a larger percentage of total crashes for non-experienced-middle-aged drivers (PAR per cent of cell phone holding: 2.63 vs 4.99; cell phone texting: 0.22 vs 3.20). Besides, PAR percentages of cell phone talking/listening handheld for both groups of drivers are NA. That indicates this distraction task only accounts for very few percentages of total crashes for all drivers.
As shown in Table IV, PAR percentages with respect to the cell phone dialing hand-held stay low as all groups of drivers scarcely dial hand-held while driving, which means it only contributes to a rather low percentage of total crashes for all drivers.
According to Table V, cell phone talking/listening hand-held has a relatively high prevalence which varies within 1.75 −4.38 per cent for all groups of drivers except the senior; however, as shown in Table IV, corresponding PAR percentages for all drivers except the senior are NA. Again, that indicates cell phone talking/listening hand-held also contributes to very few percentages of total crashes for all drivers except the senior.
3.2 Analysis of prevalence of engagements
As shown in Table VI, the prevalence of being engaged in cell phone engagements during driving is 8.31 per cent. The cell phone talking/listening hand-held (3.20 per cent) is the most frequently happened task, followed by the cell phone holding (2.18 per cent) and the cell phone texting (1.95 per cent). Remaining prevalence of distractions associated with the cell phone, which includes the cell phone browsing (0.83 per cent), cell phone locating/reaching/answering (0.62 per cent) and cell phone dialing hand-held (0.12 per cent), were observed less than 1.0 per cent. Mixed logistic regression models were performed to examine whether driver demographic characteristics (gender, age and driving experience), as well as environmental conditions (light, traffic density, weather and time of the day), were statistically significant for various cell phone engagements while driving. As a result, most environment variables did not appear to be related to cell phone engagements, except for the weather condition (P < 0.05). Thus, only demographic variables and weather conditions were considered in the regression model.
According to analysis results, gender did not appear to be related to most types of cell phone engagements while driving, except for the overall cell phone use (P < 0.05) and cell phone talking/listening hand-held (P <* 0.001). As shown in Figure 5(a) and (b), being female was related to an approximately 18 per cent increase in the probability of observed using a cell phone when compared with male drivers; the difference of the EMM regarding overall cell phone use is 1.20 per cent (8.80 vs 7.60 per cent, P <0.05). The same pattern can be observed for talking/listening hand-held, with the increased odds of engaging in this task is about 33 per cent for female drivers; the difference of the EMM regarding cell phone talking/listening hand-held is 1.60 per cent compared to male drivers (6.90 per cent for females vs 5.30 per cent for males, P <0.001).
Age factor has a significant contribution to the likelihood of cell phone engagements for all cell phone-related secondary tasks except the cell phone dialing hand-held, cell phone locating/reaching/answering and cell phone browsing. In those cases, compared with young and middle-aged drivers, senior drivers were less likely to engage in any cell phone-related tasks (P <* 0.001), more specifically, young drivers were associated with a 7.6 times increase in the odds of using a cell phone, and middle-aged drivers were related to a 6.4 times increase. As shown in Figure 5(a), EMMs of the likelihood of cell phone engagements for three age groups are 16.20 per cent, 14.20 per cent, and 2.20 per cent. The difference of the EMM between young drivers and senior drivers is 14.00 per cent (P <0.001), while that between young drivers and middle-aged drivers is 12.00 per cent (P < 0.001).
Young and middle-aged drivers also tend to engage in cell phone visual-manual demanded tasks more frequently than senior drivers, with the increased odds of engaging in this type of distraction being about 1.28 times for young drivers and almost 76 per cent for middle-aged drivers. As shown in Figure 5(c), EMMs of the likelihood of cell phone engagements for three age groups is 7.90 per cent, 6.20 per cent, and 3.60 per cent, and the difference of the EMM between young drivers and senior drivers is 4.30 per cent (P <0.001), while that between the middle-aged and the senior is 2.60 per cent (P < 0.001). Moreover:
A similar pattern was also found in talking/listening handheld, with the increased odds of engaging in this behavior is about 81 per cent for young drivers and nearly 125 per cent for middle-age drivers. As shown in Figure 5(b), EMMs regarding this behavior for three age groups are 6.80 per cent, 8.30 per cent, and 3.90 per cent. The difference of the EMM between the young and the senior is 2.90 per cent (P <0.01), while that between the middle-aged and the senior is 4.40 per cent (P <0.001).
The likelihood of being observed holding a cell phone while driving was dramatically higher for young drivers (more than 71 per cent) and middle-age drivers (more than 64 per cent) when compared to senior drivers. As shown in Figure 5(d), EMMs regarding this activity for three age groups are 6.30 per cent, 6.10 per cent, and 3.80 per cent. The difference of the EMM between the young and the senior is 2.50 per cent (P<0.01), while that between the middle-aged and the senior is 2.30 per cent (P<0.001).
Senior drivers are associated with a larger than 53 per cent decrease in the likelihood of being observed texting. As shown in Figure 5(e), corresponding EMMs regarding this behavior for three age groups are 6.30 per cent, 5.50 per cent, and 3.70 per cent, with the difference of the EMM between the young and the senior is 2.70 per cent (P<0.01), while that between the middle-aged and the senior is 1.80 per cent (P<0.001).
Driving experience also emerged as a significant predictor for the likelihood of overall cell phone use, cell phone visual-manual demanded tasks, as well as subtasks such as cell phone holding, cell phone texting and cell phone talking/listening handheld. Experienced drivers were associated with a larger than 68 per cent decrease in the odds of being observed engaged in all cell phone-related tasks when compared with less-experienced drivers, as shown in Figure 5(a); the difference of the EMM is 4.00 per cent (6.20 vs 11.20 per cent, P<0.001). Moreover, the likelihood of being observed in cell phone visual-manual tasks was also much lower for experienced drivers, as shown in Figure 5(c); the difference of the EMM is 2.10 per cent (4.70 vs 6.80 per cent, P < 0.05) when compared with less-experienced drivers. Similar patterns were also found for cell phone holding, cell phone texting and cell phone talking/listening handheld; in those cases, likelihoods of cell phone engagements were lower for experienced drivers, with the decreased odds of being 71.3, 58.1 and 47.0 per cent. More specifically:
Experienced drivers were associated with an approximately 30 per cent decrease in the odds of being observed talking/listening handheld. As shown in Figure 5(b), EMMs of the likelihood regarding this task for the experienced and the less-experienced are 7.20 and 5.00 per cent, and the difference of the EMM is 2.20 per cent (P<0.01).
Engagements of being observed holding a cell phone while driving were lower for experienced drivers, with the decreased odds of being about 71 per cent. As shown in Figure 5(d), the EMM regarding this activity for experienced and less-experienced drivers is 4.10 per cent and 6.70 per cent, respectively, and the difference of the EMM is 2.60 per cent (P<0.01).
The likelihood of being observed texting while driving was dramatically higher for less-experienced drivers (about 58 per cent) when compared to experienced drivers. As shown in Figure 5(e), the EMM regarding this activity for the experienced and the less-experienced are 6.20 and 4.00 per cent, respectively. What is more, the difference of the EMM is about 2.20 per cent (P<0.05).
Finally, as shown in Figure 5(a) and (b), the likelihood of engaging in all cell phone-related tasks, as well as cell phone talking/listening hand-held, was lower when adverse weather conditions (e.g. fog, rain, snow) were recorded, with the incensement being between 25 and 36 per cent, and corresponding differences of the EMM regarding these two types of engagements are 1.70 per cent (9.10 vs 7.40 per cent, P <* 0.05), and 1.80 per cent (7.00 vs 5.20 per cent, P < 0.05).
4. Discussion and conclusion
Secondary task related to cell phone usages during driving is a major cause of traffic accidents. On the basis of the SHRP 2 NDS data, this research quantitatively evaluated the cell phone use on driving safety impacts both at the individual and large-population level. One interesting finding in this study is that talking/listening to a cell phone handheld seems not to significantly increase the crash risk, which is consistent with some other naturalistic driving studies (Klauer et al., 2014). The reason might be talking/listening to a cell phone handheld is generally considered as a cognitive task that may not require too much attention from the driver.
Analysis results in this study indicate that risks of the cell phone engagement may substantially differ because of driver demographic characteristics such as age, driving experience or their combinations. The impact of cell phone engagement regarding the crash risk is significantly higher for young drivers than middle-aged drivers, which is in accordance with the results of Guo et al. (2017). Moreover, senior drivers increased crash odds when engaged in cell phone dialing hand-held, texting, holding or browsing. One of the potential reasons could be senior drivers perceived a higher level of hazard for most mobile phone-related tasks when compared to the young and the middle-aged drivers (Ferreira et al., 2013). Generally, they need more time to text by hand, thus, they made longer internal glances which degraded the control of the vehicle to a greater extent for senior drivers (Owens et al., 2011). However, nearly all PAR percentages of cell phone-related secondary tasks were the lowest for senior drivers. Just as Papantoniou et al. (2015) pointed out, cell phone use did not account for a large number of crashes for older drivers, it would increase the crash risk for them. That means, compared with young drivers, using a cell phone was much more dangerous but was performed less frequent by the senior. Additionally, most PAR percentages of cell phone-related tasks for young drivers were the highest, which indicates that using cell phone might contribute to more crashes for young drivers; one explanation could be they might be likely to underestimate road risks (Taubman-Ben-Ari and Lotan, 2011).
The degree of crash risk respecting to cell phone engagements varies with the driving experience. More specifically, less-experienced drivers confront a larger crash risk compared to experienced drivers when distracted by cell phone. This finding is also consistent with the conclusions from Klauer et al. (2014). In addition, most PAR percentages of specific cell phone-related subtask were obviously lower for experienced drivers than that of less-experienced drivers, which indicates cell phone usage accounted for fewer crashes for experienced drivers. Potential reasons could be mature risk-management skills may take a longer time to develop than driving skills (Stavrinos et al., 2013), therefore, drivers with less than 10 years of driving experience might be easily over-confident in their driving skills while multitasking and over-estimating their risk-management capabilities. In addition, a previous study indicates that young novice drivers had higher crash risk while engaged in texting compared with experienced drivers (Klauer et al., 2014).
The prevalence of the overall cell phone use in this study is considerably higher than that reported in Albans (Hertfordshire), England by Sullman et al. (2015), as well as in Spain by Prat et al. (2015); however, it is lower than that reported in Alabama, USA by Huisingh et al. (2015). These differences may come from multiple reasons such as the method of data collection (roadside observation and naturalistic in-vehicle observation), cultural factors or differences in legislation and the level of enforcement (e.g. handheld mobile phone use was illegal in St. Albans and Spain, whereas it is legitimate in Alabama).
In addition, our results also suggest that the prevalence of certain distracting activities related to cell phones varies with demographic factors such as gender, age and driving experience. More specifically, female drivers are more likely to engage in cell phone while driving than male drivers. Indeed, in the periodic studies conducted in the USA, the prevalence of cell phone use of women was higher (Glassbrenner, 2005; Pickrell et al., 2016). While a majority of studies conducted outside the USA show that male drivers were more likely to use cell phone while driving than female drivers (Sullman et al., 2015; Vollrath et al., 2016; Wundersitz, 2014). Similar patterns are also observed for specific cell phone-related tasks. For instance, talking/listening to the cell phone handheld was more frequently seen for female drivers, whereas the opposite was true for conversing on a handheld mobile phone, as reported by Sullman et al. (2015). Gender did not appear to be related to the frequency of cell phone visual-manual task engagements (i.e. texting, dialing, reaching). O'Brien et al. (2010) showed that there was no obvious difference in texting prevalence observed in male and female drivers. Sullman et al. (2015) also found that gender was not a significant predictor for texting; similarly, Huisingh et al. (2015) concluded that there were no differences in the proportion of male and female drivers observed for texting/dialing a phone.
Age differences found in this study were broadly verified by previous questionnaire-based research (Nurullah et al., 2013; Young and Lenné, 2010), as well as a roadside observational study (Huisingh et al., 2015; Prat et al., 2015; Sullman et al., 2015). Age was a significant predictor of engagements in cell phone. Drivers older than 60 years were less likely to be engaged in driving distractions related to cell phone compared with younger drivers (<59 years), which is in agreement with several previous observational studies (Huisingh et al., 2015; Prat et al., 2015; Sabzevari et al., 2016; Sullman et al., 2015). However, the prevalence of cell phone-related secondary tasks between young and middle-aged drivers did not differ much in this study. Those roadside observational research studies in England or in Alabama indicated that being middle-aged was associated with lower odds of being distracted by cell phone (Sullman et al., 2015). The reason for the difference might be young people seem to use modern technology more frequently, which also transfers into the driving environment, while middle-aged drivers may have more driving journeys for work-related purposes.
In addition, the prevalence of most tasks related to cell phone is the lowest for senior or experienced drivers. The reason might be related to the drivers’ self-regulation. Self-regulation is the dynamic process in which drivers manage competing demands, while simultaneously avoiding collisions (Oviedo-Trespalacios, 2018). Self-regulation in drivers occurs at three different levels including operational, tactical and strategic. Tactical self-regulation includes deciding when or where to engage in secondary tasks related to mobile phones. According to Young and Regan (2013), tactical control involves adjusting or controlling the time of secondary task engagement such as stopping talking or delaying responses to ringing phones. Hancox et al. (2013) concluded that roadway demands and phone functionality can affect the willingness of drivers to engage with mobile phones. Oviedo-Trespalacios (2018) suggested that the length of time of having a driving license would significantly influence the likelihood of engaging in secondary tasks related to mobile phones, with more experienced drivers associated with less likelihood of distracted driving. Therefore, drivers are more willing to answer calls than to initiate calls, and senior/experienced drivers are less likely to engage in a cell phone call because they are more sensitive to varying road and context conditions, while young novice drivers show no such sensitivity (Andrews and Westerman, 2012; Tractinsky et al., 2013).
In conclusion, this research demonstrates that demographic characteristics are potentially related to crash risk when engaging in cell phone-related tasks while driving. Young drivers and senior drivers are found to be more susceptible to be distracted by cell phones, and cell phone usages account for a much larger percentage of total crashes for young drivers. Experienced drivers confront lower risks when engaging in cell phone-related tasks when compared with the less-experienced. Similarly, a smaller percentage of total crashes is attributed to cell phone usages for experienced drivers. Moreover, experienced-middle-aged drivers engaging in all cell phone-related tasks would confront lower crash risks than other drivers. Also, cell phone usages contribute to a lower percentage of total crashes for experienced-middle-aged drivers.
In view of the above, road safety policymakers could better understand the negative effects of cell phone use while driving from this study, with the aim of formulating appropriate strategies in the field of road safety. Thus, more comprehensive training systems should be established to educate potential drivers, especially for young or novice ones, so that they are fully aware of the importance of driving safety. Nevertheless, the prevalence of cell phone use is still large (Waddell and Wiener, 2014; Oviedo-Trespalacios et al., 2017) and current legislation and enforcement alone do not prevent drivers using handheld cell phones (Ehsani et al., 2016; Nevin et al., 2016; Oviedo-Trespalacios, 2018). Parnell et al. (2017) argue that legislation on distraction is based on driver behavior but overlooks the role of the wider road transport system, e.g. car manufacturers and mobile phone designers. Therefore, car manufacturers and mobile phone designers can develop new ideas to reduce the rates of cell phone usage when driving such as “textalyzer” detection devices (Richtel, 2016), thereby preventing crashes and injuries. Furthermore, the applications of the advanced driver assistance systems, such as autonomous emergency braking, collision avoidance system and lane-departure warning system, are also encouraged to avoid crashes by cell phone usage while driving.
Figures
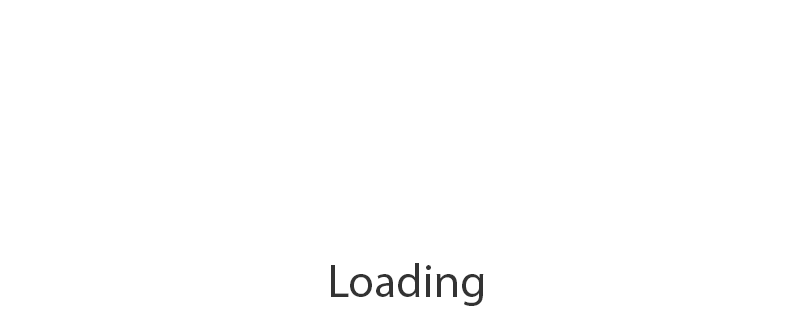
Figure 5
EMM of the likelihood of the cell phone engagement (F.: female, M.: male, Y.: young, Mid.: middle-aged, S.: senior, Less-: less-experienced, Exp.: experienced, Wea.: weather. Wea.* represents adverse weather conditions were recorded. EMM: Estimated Marginal Mean. Significance level: ***P < 0.001, **P <* 0.01, *P < 0.05)
The levels of crash severities
Level | Severities |
---|---|
1 | Airbag/injury/rollover, high delta-V crashes (virtually all would be police reported) |
2 | Police-reportable crashes (including police-reported crashes, as well as others of similar severity which were not reported) |
3 | Crashes involving physical contact with another object |
4 | Tire strike; low-risk crashes |
Source: Dingus et al. (2015)
Each specific cell phone task and its definition
Cell phone-related task | Definition in SHRP 2 |
---|---|
Overall cell phone use | All secondary tasks related to cell engagements during driving |
Cell phone visual-manual task | Including dialing hand-held, locating/reaching/answering, texting |
Dialing hand-held | The subject driver is pushing a number buttons on a cell phone or touch screen to dial/browse/check something else on their phone; the subject driver is holding a phone but not manipulating it |
Holding | The subject driver is holding a phone but not manipulating it |
Locating/reaching/answering | The subject driver is glancing at find phone, reaching toward his/her cell phone and flipping phone open or pressing a button to answer a call |
Texting | The subject driver is pressing buttons on cell phone or touch screen to create and/or send a text message |
Browsing | The subject driver is pressing buttons on cell phone or touch screen to browse the internet or phone applications |
Talking/listening, hand-held | The subject driver is talking on a handheld phone or has a phone up to ear as if listening to a phone conversation or waiting for the person they are calling to pick up the phone |
The numbers of crashes and baseline epochs where each cell phone-related task was present and absent
Secondary task | Present | Absent | ||
---|---|---|---|---|
Baseline | Crash | Baseline | Crash | |
Overall cell phone use | 1,639 | 186 | 18,075 | 1,246 |
Cell visual-manual | 517 | 87 | 19,197 | 1,345 |
Browsing | 164 | 16 | 19,550 | 1,416 |
Dialing handheld | 24 | 8 | 19,690 | 1,424 |
Holding | 430 | 95 | 19,284 | 1,337 |
Locating/reaching | 123 | 21 | 19,591 | 1,411 |
Talking/listening, hand-held | 631 | 42 | 19,083 | 1,390 |
Texting | 385 | 65 | 19,329 | 1,367 |
The PAR percentage (with 95% CI) of cell phone use by driver demographics
Secondary task | Young | Middle-aged | Senior |
---|---|---|---|
PAR% (CI) | PAR% (CI) | PAR% (CI) | |
Overall cell phonecell phone use | 7.73 (4.35,11.59) | 1.39 (−1.53,5.35) | 1.86 (0.40,4.54) |
Cell phoneCell phone visual-manual tasks | 5.22 (2.98,8.03) | 1.56 (−0.06,4.22) | 1.38 (0.36,3.92) |
Holding | 6.74 (4.40,9.66) | 2.34 (0.58,5.13) | 2.01 (0.70,5.05) |
Texting | 4.49 ( 2.48,7.09) | 0.35 (0.01,2.50) | 0.68 (0.07,2.93) |
Locating/Reaching/Answering | 1.36 (0.36,3.02) | 0.55 (−0.13,2.41) | NA |
Dialing Handheld | 0.20 (−0.04,1.32) | 0.56 (0.01,2.41) | 0.87 (0.07,7.92) |
Browsing | 0.29 (−0.45,1.44) | NA | 0.53 (0.06,6.25) |
Talking/Listening, hand-held | NA | NA | NA |
Secondary task | Less-experienced | Experienced |
PAR% (CI) | PAR% (CI) | |
Overall cell phone use | 7.07 (3.89,10.65) | 0.93 (−0.72,3.19) |
Cell phone visual-manual tasks | 5.21 (3.11,7.82) | 0.90 (−0.01,2.45) |
Holding | 6.08 (3.95,8.72) | 2.19 (0.99,4.05) |
Texting | 4.05 (2.22,6.40) | 0.38 (−0.25,1.67) |
Locating/reaching/answering | 1.32 (0.39,2.84) | 0.14 (−0.17,1.16) |
Dialing handheld | 0.56 (0.11,1.86) | 0.31 (0,1.39) |
Browsing | 0.38 (−0.39,1.71) | NA |
Talking/listening, hand-held | NA | NA |
Secondary task | Non-middle-aged- experienced | Middle-aged-experienced |
PAR% (CI) | PAR% (CI) | |
Overall cell phone use | 6.15 (3.94,8.66) | 0.87 (−2.12,5.08) |
Cell phone visual-manual tasks | 4.25 (2.75,6.11) | 0.59 (−0.79,3.33) |
Holding | 4.99 (3.45,6.91) | 2.63 (0.71,5.90) |
Texting | 3.20 (1.91,4.86) | 0.22 (−0.79,2.63) |
Locating/reaching/answering | 0.91 (0.28,1.94) | 0.53 (−0.17,2.78) |
Dialing handheld | 0.63 (0.21,1.70) | NA |
Browsing | 0.42 (−0.13,1.36) | NA |
Talking/listening, hand-held | NA | NA |
CI: Confidence interval; PAR%: population attributable risk percentage; NA indicates that the odds ratios of specific cell task for this individual subgroup are less than 1.0 or prevalence of specific cell task for this individual subgroup is null
Prevalence of the cell phone usage by different driver demographics
Secondary task | Young | Middle-aged | Senior |
---|---|---|---|
Pe(%) | Pe(%) | Pe(%) | |
Overall cell phone use | 12.98 | 8.84 | 1.25 |
Cell phone visual-manual tasks | 4.58 | 2.37 | 0.30 |
Talking/listening, hand-held | 4.12 | 4.07 | 0.76 |
Holding | 3.65 | 2.12 | 0.27 |
Texting | 3.50 | 1.74 | 0.15 |
Browsing | 1.45 | 0.77 | 0.08 |
Locating/reaching/answering | 1.11 | 0.50 | 0.13 |
Dialing handheld | 0.10 | 0.22 | 0.02 |
Secondary task | Less-experienced | Experienced |
Pe(%) | Pe(%) | |
Overall cell phone use | 13.03 | 4.78 |
Cell phone visual-manual tasks | 4.44 | 1.25 |
Talking/listening, hand-held | 4.38 | 2.32 |
Holding | 3.69 | 1.06 |
Texting | 2.23 | 0.84 |
Browsing | 1.43 | 0.38 |
Locating/reaching/answering | 1.02 | 0.31 |
Dialing handheld | 0.11 | 0.13 |
Secondary task | Non-middle-aged- experienced | Middle-aged-experienced |
Pe(%) | Pe(%) | |
Overall cell phone use | 8.51 | 7.86 |
Cell phone visual-manual tasks | 2.85 | 2.09 |
Talking/listening, hand-held | 2.98 | 3.72 |
Holding | 2.37 | 1.75 |
Texting | 2.17 | 1.45 |
Browsing | 0.91 | 0.66 |
Locating/reaching/answering | 0.69 | 0.47 |
Dialing handheld | 0.73 | 0.24 |
Mixed effect of logistic regression model results regarding the likelihood of cell phone engagements
Overall cell phone | Cell phone VM tasks | Dialing handheld | Holding | |||||
---|---|---|---|---|---|---|---|---|
Wald | OR | Wald | OR | Wald | OR | Wald | OR | |
Intercept | −24.822*** | 0.014 | −21.048*** | 0.029 | −16.449 | 0.028 | −20.174*** | 0.029 |
Gender (F.) | 2.160* | 1.176 | 0.364 | 1.030 | 0.282 | 1.035 | −1.091 | 0.909 |
Age (Young) | 11.119*** | 8.645 | 4.062*** | 2.284 | 0.237 | 1.072 | 2.650** | 1.719 |
Age (Mid.) | 13.703*** | 7.411 | 4.308*** | 1.761 | 0.557 | 1.089 | 3.731*** | 1.649 |
Age (Senior) | 0a | Ref. | 0a | Ref. | 0a | Ref. | 0a | Ref. |
Less-exp. | 3.691*** | 1.684 | 2.380* | 1.486 | −0.130 | 0.968 | 3.245*** | 1.713 |
Wea.* | 2.096* | 1.250 | 0.836 | 1.125 | 0.174 | 1.036 | 1.026 | 1.166 |
L/R/A | Texting | Browsing | T/L, hand-held | |||||
Wald | OR | Wald | OR | Wald | OR | Wald | OR | |
Intercept | −17.356*** | 0.030 | −20.013 | 0.029 | 17.991*** | 34.81 | 22.139*** | 0.025 |
Gender (F.) | −0.271 | 0.970 | 0.171 | 1.015 | 0.109 | 1.012 | 3.652*** | 1.332 |
Age (Young) | 1.413 | 1.481 | 2.672** | 1.781 | 1.135 | 1.332 | 3.330*** | 1.813 |
Age (Mid.) | 0.927 | 1.147 | 3.060** | 1.527 | 1.520 | 1.249 | 6.873*** | 2.246 |
Age (Senior) | 0a | Ref. | 0a | Ref. | 0a | Ref. | 0a | Ref |
Less-exp. | 0.966 | 1.010 | 2.574** | 1.581 | 1.122 | 1.273 | 2.780** | 1.470 |
Wea.* | 0.761 | 1.058 | 0.462 | 1.073 | 0.763 | 1.054 | 2.157* | 1.358 |
VM: visual-manual; T/L: talking/listening; F.: female; Mid.: middle-aged; Less-exp.: less-experienced; Wea.* represents adverse weather conditions were recorded. L/R/A: locating/reaching/answering. Significance level: ***P <* 0.001; **P < 0.01; *P <* 0.05
Predictors and targets by level in regression models
Predictor/Targets | Values |
---|---|
Gender | 0 = female, 1 = male |
Age | 1 = young, 2= middle-aged, 3 = senior |
Driving experience | 0 = less-experienced, 1 = experienced |
Combination of age and experience | 0 = experienced middle-aged, 1 = others (non-experienced-middle-aged) |
Secondary task (specific) | 0 = not engaged any secondary task, 1 = engaged one specific secondary task |
Weather | 0 = no adverse weather recorded, 1 = adverse weather recorded |
Light | 0 = daylight, 1 = Night |
Traffic density | 1 = low, 2 = medium, 3 = high |
Time of the day | 1 = morning rush hour, 2 = evening rush hour, 3 = others |
Safety consequence (target) | 0 = baseline, 1 = crash |
Cell phone engagement (target) | 0 = not engaged, 1 = engaged |
ORs (with 95% CI) of the Cell phone use by driver demographics
Secondary task | Young | Middle-aged | Senior |
---|---|---|---|
OR (CI) | OR (CI) | OR (CI) | |
Overall cell phone use | 1.64 (1.35,2.01) | 1.16 (0.83,1.64) | 2.51 (1.32,4.80) |
Cell phone visual-manual tasks | 2.20 (1.67,2.90) | 1.67 (0.98,2.86) | 5.63 (2.19,14.47) |
Dialing handheld | 2.98 (0.62,14.36) | 3.55 (1.03,12.25) | 44.75 (4.64,431.35) |
Holding | 2.98 (2.26,3.93) | 2.13 (1.28,3.55) | 8.63 (3.59,20.70) |
Locating/reach/answering | 2.24 (1.32,3.81) | 2.11 (0.75,5.94) | NA |
Texting | 2.34 (1.73,3.18) | 1.20 (0.58,2.47) | 5.59 (1.48,21.15) |
Browsing | 1.41 (0.80,2.48) | 0.34 (0.04,2.42) | 3.71 (0.41,33.25) |
Talking/listening, hand-held | 0.87 (0.58,1.33) | 0.96 (0.56,1.62) | 0.74 (0.18,3.07) |
Secondary task | Less-experienced | Experienced |
OR (CI) | OR (CI) | |
Overall cell phone use | 1.58 (1.31,1.92) | 1.20 (0.85,1.69) |
Cell phone visual-manual tasks | 2.24 (1.72,2.91) | 1.72(0.99,3.00) |
Dialing handheld | 6.09 (2.04,18.22) | 3.43 (0.99,11.87) |
Holding | 2.75 (2.11,3.59) | 3.11 (1.94,4.98) |
Locating/reaching/answering | 2.31 (1.38,3.87) | 1.47 (0.45,4.78) |
Texting | 2.23 (1.66,2.99) | 1.46 (0.71,3.02) |
Browsing | 1.27 (0.73,2.21) | 0.79 (0.19,3.29) |
Talking/listening, hand-held | 0.91 (0.63,1.32) | 0.72 (0.39,1.32) |
Secondary task | Non-middle-aged-experienced | Middle-aged-experienced |
OR(CI) | OR(CI) | |
Overall cell phone use | 1.77 (1.48,2.11) | 1.11 (0.74,1.68) |
Cell phone visual-manual tasks | 2.55 (1.99,3.28) | 1.29 (0.62,2.65) |
Dialing handheld | 9.80 (3.86,24.87) | NA |
Holding | 3.22 (2.51,4.14) | 2.54 (1.41,4.58) |
Locating/reaching/answering | 2.33 (1.40,3.87) | 2.13 (0.65,7.06) |
Texting | 2.52 (1.90,3.35) | 1.15 (0.47,2.86) |
Browsing | 1.47 (0.86,2.51) | 0.51 (0.07,3.70) |
Talking/listening, hand-held | 0.98 (0.68,1.40) | 0.80 (0.41,1.58) |
CI: 95% confidence interval; OR: odds ratio; Italic text indicates statistically significant OR at the 0.05 level; NA indicates that no crash with cell task was observed in the data set or no statistical significance reported between the cell task and the crash
Appendix. Details on statistical methods used in this study
As multiple crashes and baselines can be derived from one driver, then another mixed effect random logistic regression model including the interaction term between one secondary task and one exposure of interest was adopted to incorporate the driver-specific correlation. That means analyses were conducted for both crashes and controls overall for each demographic (gender, age group or driving experience group) when considering one specific distraction activity (listed in Table II) while driving, which aims to examine whether the driver demographic characteristic would significantly affect the driving safety when drivers engaged in cell phone-related secondary tasks during the driving. The risk associated with cell phone engagements is evaluated through a comparison with alert, attentive and sober driving episodes. Assuming that,
And supposing the observed safety consequence Y is expected to be followed by a Bernoulli distribution,
where pij is the probability of event j being a crash for driver i. Then, the probability pij is associated with a set of covariates by a logit link function, such that,
For example, as cell use for distraction in SHRP 2 event data set is determined by three variables, namely, “Secondary Task 1”, “Secondary Task 2”, and “Secondary Task 3” (we use “secondary task 1/2/3” to denote those variables). Then the independent variable of secondary task engagement Xst is defined as:
Moreover, Xdc represents a nominal variable of one specific driver demographic characteristic (age group, driving experience group or the combination group of the age and driving experience), [Xst * Xdc] represents the interaction effect between the exposure of interest and one specific secondary task engagement listed in Table III, βi i = 0,1,2,3 are the regression parameters and αi is a driver-specific random term. If the interaction effect in the regression model is significant at 0.05 level, then the EMM of the interaction effect respecting to the crash risk would be obtained by using the professional statistical software IBM SPSS Statistics©.
Similar procedures are performed when investigating the correlation among the likelihood of the cell phone-related engagement and demographic characteristics (gender, age and driving experience) and environment conditions (weather, light, traffic density and time of the day). Please follow Table AI to get more detailed information on predictors and targets by level.
Population attributable risk percentage
One possible calculation method for the PAR percentage among the population is based on the “population relative risk” that is determined as the relative risks for the two groups comprising the population, and the proportion of the population exposed that is referred to as “prevalence” in this study. The relative risk is commonly referred to as if it were the usual measure of risk obtained in a case-control study such as OR. OR is a measure of association between an outcome (e.g. a crash involvement) and an exposure (e.g. one secondary task engagement), which represents the odds that an outcome will occur, given a particular exposure compared to the odds of the outcome occurring in the absence of that exposure. To obtain the PAR percentage, first, a 2 × 2 contingency table would be constructed to obtain the OR for a specific secondary task in a specific driver group (gender, age or driving experience group) were exposed and unexposed groups combined must form the entire population. Algebraically, this equation can be written as shown in (A5),
where,
A = the number of crashes where one specific secondary task was present (while without any other type of secondary task);
B = the number of baseline epochs where one specific secondary task was present (while without any other type of secondary task);
C = the number of crashes where no such secondary task was present (but can have other secondary tasks); and
D = the number of baseline epochs where no such secondary task was present (but can have other secondary tasks).
Table AII shows the OR (with corresponding 95 per cent confidence intervals) of each cell phone-related secondary task by age groups, driving experience groups or their combinations. It is important to notice that the resulting odds ratio by (A5) only reflects the crash risk of engaging in one specific secondary task compared with not engaging in it (which could possibly engage in other secondary tasks).
Moreover, if the OR value of the secondary task for one group of drivers is over 1.0, then the PAR percentage is evaluated by,
Where Pe denotes the prevalence of one specific cell phone use, and OR denotes the relative risk estimate for a crash. When assessing the PAR percentage for a secondary task related to the cell phone use, the baseline prevalence Pe was calculated by counting the number of baseline epochs where a specific task associated with the cell phone use was present and counting the total number of baseline epochs (“m of baseline epochs with a cell phone use present”+ “n of baseline epochs where no such type of cell phone use was present”), namely,
For example, baseline epochs of the cell phone texting for young drivers are 251, while the baseline epochs, where no such type of inattention was present, are 6,927, then the baseline prevalence of the cell phone texting for female drivers is:
According to Table A2, if the OR of cell phone texting, in this case, is 2.34, then the resulting PAR percentage is,
That indicates the cell phone texting contributed to 4.49 per cent of all crashes for young drivers.
References
Andrews, E.C. and Westerman, S.J. (2012), “Age differences in simulated driving performance: compensatory processes”, Accident Analysis & Prevention, Vol. 45 No. 1, pp. 660-668.
Asbridge, M., Brubacher, J.R. and Chan, H. (2013), “Cell phone use and traffic crash risk: a culpability analysis”, International Journal of Epidemiology, Vol. 42 No. 1, pp. 259-267.
Caird, J.K., Johnston, K.A., Willness, C.R., Asbridge, M. and Steel, P. (2014), “A meta-analysis of the effects of texting on driving”, Accident Analysis & Prevention, Vol. 71, pp. 311-318.
Caird, J.K., Simmons, S.M., Wiley, K., Johnston, K.A. and Horrey, W.J. (2018), “Does talking on a cell phone, with a passenger, or dialing affect driving performance? An updated systematic review and meta-analysis of experimental studies”, Human Factors: The Journal of the Human Factors and Ergonomics Society, Vol. 60 No. 1, pp. 101-133.
Chaparro, A., Wood, J.M. and Carberry, T.P. (2005), “Effects of age and auditory and visual dual tasks on closed-road driving performance”, Optometry and Vision Science, Vol. 82 No. 8, pp. 747-754.
Cole, P. and Macmahon, B. (1971), “Attributable risk percent in case-control studies”, Journal of Epidemiology & Community Health, Vol. 25 No. 4, pp. 242-244.
Choudhary, P. and Velaga, N.R. (2019), “Effects of phone use on driving performance: a comparative analysis of young and professional drivers”, Safety Science, Vol. 111, pp. 179-187.
Collet, C., Guillot, A. and Petit, C. (2010), “Phoning while driving i: a review of epidemiological, psychological, behavioural and physiological studies”, Ergonomics, Vol. 53 No. 5, pp. 589-601.
Dingus, T.A., Guo, F., Lee, S., Antin, J.F., Perez, M., Buchanan-King, M. and Hankey, J. (2016), “Driver crash risk factors and prevalence evaluation using naturalistic driving data”, Proceedings of the National Academy of Sciences, Vol. 113 No. 10, pp. 2636-2641.
Dingus, T.A., Hankey, J.M., Antin, J.F., Lee, S.E., Eichelberger, L., Stulce, K.E., McGraw, D., Perez, M. and Stowe, L. (2015), “Naturalistic driving study: technical coordination and quality control (shrp 2 report s2-s06-rw-1)”, Pneuma, Vol. 36, pp. 501-503.
Ehsani, J.P., Ionides, E., Klauer, S.G., Perlus, J.G. and Gee, B.T. (2016), “Effectiveness of cell phone restrictions for young drivers”, Transportation Research Record: Journal of the Transportation Research Board, Vol. 2602 No. 1, pp. 35-42.
Ferreira, A.L., Piccinini, G.F.B., Rôla, S. and Simões, A. (2013), “Gender and age-related differences in the perception of in-vehicle mobile phone usage among Portuguese drivers”, IET Intelligent Transport Systems, Vol. 7 No. 2, pp. 223-229.
Glassbrenner, D. (2005), Driver Cell Phone Use in 2004–Overall Results Report. No. DOT HS 809 847.
Guo, F. and Hankey, J.M. (2009), Modeling 100-Car Safety Events: A Case-Based Approach for Analyzing Naturalistic Driving Data, VT Tech. VT Tech Transportation Institute, Blacksburg.
Guo, F., Klauer, S.G., Fang, Y., Hankey, J.M. and Dingus, T.A. (2017), “The effects of age on crash risk associated with driver distraction”, International Journal of Epidemiology, Vol. 46 No. 1, pp. 258-265.
Hancox, G., Richardson, J. and Morris, A. (2013), “Drivers' willingness to engage with their mobile phone: the influence of phone function and road demand”, IET Intelligent Transport Systems, Vol. 7 No. 2, pp. 215-222.
Harris Interactive (2011), Most U.S. Drivers Engage in ‘Distracting’ Behaviors: Poll, November 30.
He, J., Chaparro, A., Nguyen, B., Burge, R.J., Crandall, J., Chaparro, B. and Cao, S. (2014), “Texting while driving: is speech-based text entry less risky than handheld text entry”, Accident Analysis & Prevention, Vol. 72, pp. 287-295.
Huisingh, C., Griffin, R. and Mcgwin, G. (2015), “The prevalence of distraction among passenger vehicle drivers: a roadside observational approach”, Traffic Injury Prevention, Vol. 16 No. 2, pp. 140-146.
Johnson, M.B., Voas, R.B., Lacey, J.H., Mcknight, A.S. and Lange, J.E. (2004), “Living dangerously: driver distraction at high speed”, Traffic Injury Prevention, Vol. 5 No. 1, pp. 1-7.
Kass, S.J., Cole, K.S. and Stanny, C.J. (2007), “Effects of distraction and experience on situation awareness and simulated driving”, Transportation Research Part F: Traffic Psychology and Behaviour, Vol. 10 No. 4, pp. 321-329.
Klauer, S.G., Guo, F., Simons-Morton, B.G., Ouimet, M.C., Lee, S.E. and Dingus, T.A. (2014), “Distracted driving and risk of road crashes among novice and experienced drivers”, New England Journal of Medicine, Vol. 370 No. 1, pp. 54-59.
Lipovac, K., Đerić, M., Tešić, M., Andrić, Z. and Marić, B. (2017), “Mobile phone use while driving-literary review”, Transportation Research Part F: Traffic Psychology and Behaviour, Vol. 47, pp. 132-142.
Mckeever, J.D., Schultheis, M.T., Padmanaban, V. and Blasco, A. (2013), “Driver performance while texting: even a little is too much”, Traffic Injury Prevention, Vol. 14 No. 2, pp. 132-137.
Merat, N., Anttila, V. and Luoma, J. (2005), “Comparing the driving performance of average and older drivers: the effect of surrogate in-vehicle information systems”, Transportation Research Part F Traffic Psychology & Behaviour, Vol. 8 No. 2, pp. 147-166.
Metz, B., Landau, A. and Hargutt, V. (2015), “Frequency and impact of hands-free telephoning while driving – results from naturalistic driving data”, Transportation Research Part F: Traffic Psychology and Behaviour, Vol. 29, pp. 1-13.
National Highway Traffic Safety Administration (2018), Traffic safety facts: Distracted driving 2016, Report No. DOTHS-812-517, Washington, DC.
Nevin, P.E., Blanar, L., Kirk, A.P., Freedheim, A., Kaufman, R., Hitchcock, L., Maeser, J.D. and Ebel, B.E. (2016), “I wasn't texting; ‘I was just reading an email …’: a qualitative study of distracted driving enforcement in Washington state”, Injury Prevention, Vol. 23 No. 3, pp. 165-170.
Nurullah, A.S., Thomas, J. and Vakilian, F. (2013), “The prevalence of cell phone use while driving in a Canadian province”, Transportation Research Part F: Traffic Psychology and Behaviour, Vol. 19, pp. 52-62.
O'Brien, N.P., Goodwin, A.H. and Foss, R.D. (2010), “Talking and texting among teenage drivers: a glass half empty or half full?”, Traffic Injury Prevention, Vol. 11 No. 6, pp. 549-554.
Owens, J.M., Mclaughlin, S.B. and Sudweeks, J. (2011), “Driver performance while text messaging using handheld and in-vehicle systems”, Accident Analysis & Prevention, Vol. 43 No. 3, pp. 939-947.
Papantoniou, P., Antoniou, C., Yannis, G., Papadimitriou, E., Pavlou, D. and Golias, J. (2015), “P10 how cell phone use affects reaction time of older drivers”, Journal of Transport & Health, Vol. 2 No. 2, pp. S68-S69.
Parnell, K.J., Stanton, N.A. and Plant, K.L. (2017), “What’s the law got to do with it? Legislation regarding in-vehicle technology use and its impact on driver distraction”, Accident Analysis & Prevention, Vol. 100, pp. 1-14.
Pickrell, T.M., Li, R. and Kc, S. (2016), “Driver electronic device use in 2015”, Traffic Safety Facts: Research, National Highway Traffic Safety Administration. Washington, DC, Research No. DOT HS 812 326.
Prat, F., Planes, M., Gras, M.E. and Sullman, M.J.M. (2015), “An observational study of driving distractions on urban roads in Spain”, Accident Analysis & Prevention, Vol. 74, pp. 8-16.
Ranney, T. (2008), Driver Distraction: A Review of the Current State-of-Knowledge, NHTSA, Washington, DC, Research No. DOT HS 810 704.
Reimer, B., Mehler, B. and Donmez, B. (2014), “A study of young adults examining phone dialing while driving using a touchscreen vs a button style flip-phone”, Transportation Research Part F: Traffic Psychology and Behaviour, Vol. 23, pp. 57-68.
Richtel, M. (2016), Texting and Driving? Watch out for the Textalyzer, The New York, NY Times, New York, NY, Science.
Rudin-Brown, Y., Patten, L. and Ceci, M. (2013), “Driver distraction in an unusual environment; effect of text-messaging in tunnels”, Rev.bras.ginecol.obstet, Vol. 29 No. 4, pp. 331-334.
Sabzevari, J.T., Nabipour, A.R., Khanjani, N., Tajkooh, A.M. and Sullman, M.J.M. (2016), “An observational study of secondary task engagement while driving on urban streets in Iranian safe communities”, Accident Analysis & Prevention, Vol. 96, pp. 56-63.
Simmons, S.M., Hicks, A. and Caird, J.K. (2016), “Safety-critical event risk associated with cell phone tasks as measured in naturalistic driving studies: a systematic review and meta-analysis”, Accident Analysis & Prevention, Vol. 87, pp. 161-169.
Simons-Morton, B.G., Guo, F., Klauer, S.G., Ehsani, J.P. and Pradhan, A.K. (2014), “Keep your eyes on the road: young driver crash risk increases according to duration of distraction”, Journal of Adolescent Health, Vol. 54 No. 5, pp. S61-S67.
Stavrinos, D., Jones, J.L., Garner, A.A., Griffin, R., Franklin, C.A., Ball, D., Welburn, S.C., Ball, K.K., Sisiopiku, V.P. and Fine, P.R. (2013), “Impact of distracted driving on safety and traffic flow”, Accident Analysis & Prevention, Vol. 61, pp. 63-70.
Sullman, M.J.M. (2012), “An observational study of driver distraction in England”, Transportation Research Part F: Traffic Psychology and Behaviour, Vol. 15 No. 3, pp. 272-278.
Sullman, M.J.M., Prat, F. and Tasci, D.K. (2015), “A roadside study of observable driver distractions”, Traffic Injury Prevention, Vol. 16 No. 6, pp. 552-557.
Taubman-Ben-Ari, O. and Lotan, T. (2011), “The contribution of a novel intervention to enhance safe driving among young drivers in Israel”, Accident Analysis & Prevention, Vol. 43 No. 1, pp. 352-359.
Tractinsky, N., Ram, E.S. and Shinar, D. (2013), “To call or not to call—that is the question (while driving)”, Accident; Analysis and Prevention, Vol. 56, pp. 59-70.
Vollrath, M., Huemer, A.K., Teller, C., Likhacheva, A. and Fricke, J. (2016), “Do German drivers use their smartphones safely? Not really!”, Accident Analysis & Prevention, Vol. 96, pp. 29-38.
Waddell, L.P. and Wiener, K.K.K. (2014), “What’s driving illegal mobile phone use? Psychosocial influences on drivers’ intentions to use hand-held mobile phones”, Transportation Research Part F: Traffic Psychology and Behaviour, Vol. 22, pp. 1-11.
Wundersitz, L.N. (2014), “Phone use while driving: results from an observational survey”, Traffic Injury Prevention, Vol. 15 No. 6, pp. 537-541.
Young, K.L. and Lenné, M.G. (2010), “Driver engagement in distracting activities and the strategies used to minimise risk”, Safety Science, Vol. 48 No. 3, pp. 326-332.
Young, K.L. and Regan, M.A. (2013), “Defining the relationship between behavioural adaptation and driver distraction”, Behavioural Adaptation and Road Safety Theory, Evidence and Action, CRC Press, Taylor and Francis Group, Boca Raton, pp. 227-243.
Zhao, N., Reimer, B., Mehler, B., D’Ambrosio, L.A. and Coughlin, J.F. (2013), “Self-reported and observed risky driving behaviors among frequent and infrequent cell phone users”, Accident Analysis & Prevention, Vol. 61, pp. 71-77.
Further reading
Caird, J.K., Willness, C.R., Steel, P. and Scialfa, C. (2008), “A meta-analysis of the effects of cell phones on driver performance”, Accident Analysis & Prevention, Vol. 40 No. 4, pp. 1282-1293.
Dingus, T.A., Klauer, S., Vicki, R.L., Petersen, A. and Ronald, R.K. (2006), The 100-Car Naturalistic Driving Study: phase ii – Results of the 100-Car Field Experiment, Behavior.
Drews, F.A., Yazdani, H., Godfrey, C.N., Cooper, J.M. and Strayer, D.L. (2009), “Text messaging during simulated driving”, Human Factors: The Journal of the Human Factors and Ergonomics Society, Vol. 51 No. 5, pp. 762-770.
Horrey, W.J. and Wickens, C.D. (2006), “Examining the impact of cell phone conversations on driving using meta-analytic techniques”, Human Factors: The Journal of the Human Factors and Ergonomics Society, Vol. 48 No. 1, pp. 196-205.
Kidd, D.G., Tison, J., Chaudhary, N.K., Mccartt, A.T. and Casanova-Powell, T.D. (2016), “The influence of roadway situation, other contextual factors, and driver characteristics on the prevalence of driver secondary behaviors”, Transportation Research Part F: Traffic Psychology and Behaviour, Vol. 41, pp. 1-9.
Klauer, S.G., Dingus, T.A., Neale, V.L., Sudweeks, J.D. and Ramsey, D.J. (2006), The Impact of Driver Inattention on near-Crash/Crash Risk: An Analysis Using the 100-Car Naturalistic Driving Study Data, p. 31.
National Safety Council (2013), Crashes Involving Cell Phones: Challenges of Collecting and Reporting Reliable Crash Data, National Safety Council, Itasca, IL.
National Safety Council (2017), Undercounted is Underinvested: How Incomplete Crash Reports Impact Efforts to save Lives, National Safety Council, Itasca, IL.
NHTSA (2012), “State Laws”, available at: www.distraction.gov/content/getthe-facts/state-laws.html (accessed 18 July 2012).
Oscar, O.T. (2018), “Getting away with texting: behavioural adaptation of drivers engaging in visual-manual tasks while driving”, Transportation Research Part A: Policy and Practice, Vol. 116, pp. 112-121.
Oscar, O.T., Mark, K., Mazharul, H.M., Simon, W. and Boris, P. (2017), “Risk factors of mobile phone use while driving in Queensland: prevalence, attitudes, crash risk perception, and task-management strategies”, Plos One, Vol. 12 No. 9, p. e0183361.
Oscar, O.T., Mazharul, H.M., Mark, K. and Simon, W. (2018), “Should i text or call here? A situation-based analysis of drivers’ perceived likelihood of engaging in mobile phone multitasking”, Risk Analysis.
Reimer, B., Mehler, B., Wang, Y. and Coughlin, J.F. (2010), “The impact of systematic variation of cognitive demand on drivers' visual attention across multiple age groups”, Human Factors & Ergonomics Society Meeting, SAGE Publications.
SHRP2 Insight Website (2019), available at: https://insight.shrp2nds.us/ (accessed 15 March 2019).
Stavrinos, D., Garner, A.A., Franklin, C.A., Johnson, H.D., Welburn, S.C., Griffin, R., Underhill, A.T. and Fine, P.R. (2015), “Distracted driving in teens with and without attention-deficit/hyperactivity disorder”, Journal of Pediatric Nursing, Vol. 30 No. 5, pp. e183-e191.
Strayer, D.L., Crouch, D.J. and Drews, F.A. (2006), “A comparison of the cell phone driver and the drunk driver”, Social Science Electronic Publishing, Vol. 48 No. 2, pp. 381-391.
Sun, D. and Jia, A. (2016), “Impacts of cell phone use on driving safety and drivers' perception of risk”, Journal of Modern Transportation, Vol. 24 No. 2, pp. 61-68, 02.
Violanti, J.M. (1997), “Cellular phones and traffic accidents”, Public Health, Vol. 111 No. 6, pp. 423-428.
Yager, C.E. (2013), “Driver safety impacts of voice-to-Text mobile applications”, Human Factors & Ergonomics Society Meeting, SAGE Publications.
Yagil, D. (1998), “Gender and age differences in attitudes toward traffic laws and traffic violations”, Transportation Research Part F: Traffic Psychology and Behaviour, Vol. 1 No. 2, pp. 123-135.
Young, K.L., Rudin-Brown, C.M. and Lenné, M.G. (2010), “Look who’s talking! a roadside survey of drivers’ cell phone use”, Traffic Injury Prevention, Vol. 11 No. 6, pp. 555-560.
Acknowledgements
This work is supported in part by the Joint Laboratory for Internet of Vehicles, Ministry of Education-China Mobile Communications Corporation under Grant ICV-KF2018-01 and in part by the National Natural Science Foundation of China under Grant 51975194 and 51905161.We would like to thank the Transportation Research Board of the National Academies of Science (USA) for providing the SHRP2 Insight dataset. Findings and conclusions of this paper are those of authors and do not necessarily represent views of the SHRP 2, the Transportation Research Board, or the National Academies (USA).