Abstract
Purpose
This paper presents a co-authorship study of authors who published in Digital Humanities journals and examines the apparent influence of gender, or more specifically, the quantitatively detectable influence of gender in the networks they form.
Design/methodology/approach
This study applied co-authorship network analysis. Data has been collected from three canonical Digital Humanities journals over 52 years (1966–2017) and analysed.
Findings
The results are presented as visualised networks and suggest that female scholars in Digital Humanities play more central roles and act as the main bridges of collaborative networks even though overall female authors are fewer in number than male authors in the network.
Originality/value
This is the first co-authorship network study in Digital Humanities to examine the role that gender appears to play in these co-authorship networks using statistical analysis and visualisation.
Keywords
Citation
Gao, J., Nyhan, J., Duke-Williams, O. and Mahony, S. (2022), "Gender influences in Digital Humanities co-authorship networks", Journal of Documentation, Vol. 78 No. 7, pp. 327-350. https://doi.org/10.1108/JD-11-2021-0221
Publisher
:Emerald Publishing Limited
Copyright © 2022, Jin Gao, Julianne Nyhan, Oliver Duke-Williams and Simon Mahony
License
Published by Emerald Publishing Limited. This article is published under the Creative Commons Attribution (CC BY 4.0) licence. Anyone may reproduce, distribute, translate and create derivative works of this article (for both commercial and non-commercial purposes), subject to full attribution to the original publication and authors. The full terms of this licence may be seen at http://creativecommons.org/licences/by/4.0/legalcode
1. Introduction
Researchers are known to benefit from diverse interpersonal and institutional connections and networks, which have distinctive advantages for creating and sharing knowledge. For example, it is known that co-authored publications often prove to be more highly cited than single-author publications, and it is similarly known that there is a significant positive correlation between the number of authors and the number of citations (Gazni and Didegah, 2011). Collaborations with more senior or highly cited colleagues are also known to have a positive influence on the recruitment and progression of individual researchers and on institutional rankings (Gorska et al., 2020, p. 273). Less well understood is the role that gender might play in the formation and maintenance of such networks. What, then, can be concluded about the role of gender in such networks from a quantitative analysis of co-authorship networks? And what kinds of data sets and data-curation methods may advance this research?
This paper explores these questions through the microcosm of Digital Humanities (DH), an emerging field that is closely intertwined with Information Science (Bawden and Robinson, 2012, p. 7; Joo et al., 2021; Su and Zhang, 2021). Digital Humanities offers an important case study on which to conduct this research because of its “small-world” composition (Wang and Inaba, 2009; Grandjean, 2016; Tang et al., 2017), its demographic formation (as a predominately white male-oriented community, Weingart and Eichmann-Kalwara, 2017; Gao et al., 2018), and its geographical distribution of languages (forming an Anglophone-oriented network as shown in studies by De la Cruz et al., 2015; Weingart and Eichmann-Kalwara, 2017; Gao et al., 2018). Thus, the findings presented in this paper about the nature of Digital Humanities can fruitfully be brought into conversation with quantitative studies of fields with both similar and distinct compositions, opening the possibility that, in due course, information science researchers will be able to answer the broad questions set out above from the perspectives of a number of disciplines and from a global perspective.
Co-authorship links are formed when two or more scholars author a publication together. These links can be modelled as a co-authorship network. The first statistical investigation of co-authorship in DH was undertaken by (Nyhan and Duke-Williams, 2014a) based on a smaller subset of the publication metadata analysed in this study [1]. To the best of our knowledge, this paper presents the first co-authorship network study to combine co-authorship patterns with gender in the study of DH communities. Co-authorship networks have been used to investigate the structure of scientific collaborations in many fields (Newman, 2001, 2004; Smeaton et al., 2002) but, until this paper, not of the DH. This may be because few DH journals are indexed in the repositories (e.g. Web of Science and Scopus) that commonly provide publication data export for research analysis. The building of bibliometric datasets from scratch is time- and labour-intensive because of the collecting, cleaning, and coding that it entails. Yet, the practical difficulties of deriving or making datasets aside, DH communities certainly manifest many of the characteristics studied in co-authorship network analyses. Not only are DH communities interdisciplinary, the field itself is increasingly fast-growing and outputs many co-authored publications (Savić et al., 2019).
Although age and academic position usually have a more significant impact on academic production and communication, other variables, like gender, also play an important role (Noordenbos, 1992; Aksnes et al., 2011). By enriching the Digital Humanities' co-authorship network with gender information, this study takes a first step towards quantifying gender and its longitudinal trend from 1966 to 2017 in ADHO (the Alliance of Digital Humanities Organizations) and ACH (the Association for Computers and the Humanities) official DH journals. It accordingly asks of those journals: what is the gender distribution of authors of published papers? What patterns can be detected between academic productivity and gender (when productivity is conceptualized as the number of papers published)? How do gender differences affect DH interpersonal connections? How might this open new perspectives on definitions of productivity that currently define this merely as number of publications output?
This paper furthermore compiles and releases a dataset of ADHO and ACH DH journal publication metadata, together with detailed methodological reflections on the nature of its compilation to guide its use in further studies. From this dataset, this paper statistically analyses and visualizes gender-disaggregated DH co-authorship, co-author clusters, and publication-linked gender differences. In the narrative analysis of the network that follows, this paper reflects on the new knowledge we can derive about how and why authors in DH choose to co-publish.
The classification of individuals into a mutually exclusive gender-binary has been common in many cultures. While the assignment of gender according to a mutually exclusive male/female gender-binary is increasingly problematized, most empirical studies of gender in DH, including this study, continue to operationalize this framework. Using a binary gender system in quantitative studies can introduce errors when findings cannot represent identities other than the traditional male/female. Lindqvist et al., reviewed previous quantitative studies and found that “when gender distributions among participants are described in the methods section, few researchers report how this information was collected” (Lindqvist et al., 2020). Moreover, ethical problems are raised when people cannot self-identify their gender identity in published research. With better resources, and under different circumstances, this study would ideally have sought to operationalize non-binary gender frameworks. However, given the difficulties we encountered when seeking to identify and collect quantitative data on gender-diverse DH scholars, this has not been possible. Therefore, the current study uses the binary model, extended with “other/unknown” where appropriate, while acknowledging this limitation and the presence and parity of scholars in DH who are gender diverse.
We proceed by setting out this paper's wider Research Context; there follows a Methodology section illustrating this study's bibliometric data collection, author gender identification; data cleaning; network construction and analysis, which can also be adapted for other quantitative analyses of community influences; a Results and Discussion section interprets our findings and suggests interpretations of the observed gender differences explored in this paper; finally, a Conclusion reflecting on the current methodology and analysis and providing new directions for further research.
2. Research context
Feminist DH is a rich and thriving sub-field of DH. It asks questions of the past, present, and future of DH that bring (an often intersectional) feminism into conversation with the digital artefacts that the digital humanities creates, and the people, processes, platforms, ideas, histories, and more, that give rise to them, e.g. (Wernimont and Losh, 2018). Ongoing questions include how technology can advance (Wernimont, 2013), or indeed, impede the attainment of equality and co-liberation (Risam, 2015; Nyhan and Terras, 2017; Wernimont, 2018); how gender, and other modes of difference, can impact technological encounter and creation (Nowviskie, 2015; Posner, 2015) and how feminism might remake DH (#transformDH, Bailey et al., 2016), and play a role in making the wider technological landscape more representative and inclusive than it is now. Connected to this, in a range of recent publications, Feminist DH is being brought into conversation with many cognate areas of inquiry, including critical heritage studies (Smyth et al., 2020), postcolonial DH (Risam, 2018), and data feminism (D'Ignazio and Klein, 2020), emphasizing that technology and gender are far from minority concerns. Indeed, they are deeply implicated with questions of social justice and the desire for technology to be developed and embedded in society and academia in ways that are more equal and progressive than has heretofore been achieved, e.g. (Mullaney et al., 2021).
A growing number of statistical studies of DH papers have been undertaken. For example, data collected from the ADHO annual conferences have been published in a series of blogs and other publications on conference analysis that covered topics, scholars, geographic distributions, and peer-review bias of the conference submissions and programs from 2012 to 2016 (Weingart and Eichmann-Kalwara, 2017). Yet, relatively few gender-disaggregated studies of publication metrics (including conference participation) have been published. In 2014, Weingart analyzed author names, affiliations, and keywords from ADHO conference programs, and manually assigned gender information for each author as “male, female, unknown/other” (Weingart, 2014). He pointed to the unbalanced gender ratio of attendees at ADHO conferences (around 30% female scholars each year from DH2004 to DH2016). Weingart combined his gender dataset with topical keywords, and found that certain subjects are gender weighted at DH conferences:
Women are twice as likely to use the “Gender Studies” tag as male authors, whereas men are twice as likely to use the “Asian Studies” tag as female authors. Subjects related to pedagogy, creative/performing arts, art history, cultural studies, GLAM (galleries, libraries, archives, museums), DH institutional support, and project design/organization/management are more likely to be presented by women. Men, on the other hand, are more likely to write about standards and interoperability, the history of DH, programming, scholarly editing, stylistics, linguistics, network analysis, and natural language processing/text analysis. It seems DH topics have inherited the usual gender skews associated with the disciplines in which those topics originate. (Weingart, 2016a)
A regional perspective was given by Tello, who analysed the Spanish-speaking DH conference HDH2015, Humanidades Digitales Hispánicqs (Tello, 2015). Of 229 attendees, around 55% were female scholars. How indicative this is of other regional conferences cannot be said currently. It should also be noted that the distribution of topics and authors at conferences is not necessarily stable, and it is likely that patterns of conference attendance and demography vary from language to language, country to country, and year to year. To the best of our knowledge, empirical studies of gender representation in DH journals have not been previously undertaken.
Looking beyond DH, many studies have found that female scholars tend to have fewer publications than male scholars (Prpić, 2002; Symonds et al., 2006; van Arensbergen et al., 2012; Mueller et al., 2017). Differences in publication rates between male and female scholars is significant in many fields (e.g. natural sciences, technology, medicine). Based on a review of several studies, Larivière and others analysed the output of 15 universities in Québec across all fields and showed that female scholars there tended to publish around 70–80% of the output of their male colleagues (Larivière et al., 2011). In the natural sciences, gender accounts for 22% of the differences in the publication rate in favor of men, when all other variables are constant; it is 15% in engineering and technology and 8% in the field of medicine (Rørstad and Aksnes, 2015, p. 327).
To explain these results, some have invoked the metaphor of the “leaky pipeline”, where the numerically-balanced gender representation that characterises lower levels of seniority is replaced at more senior levels by an imbalance in favor of (white) males (National Science Foundation, 2018). Although seeming to contradict the above, young female researchers have been shown to outperform young male researchers in terms of the number of publications they output (van Arensbergen et al., 2012). It is suggested accordingly that female scholars seem to publish fewer publications overall because fewer female scholars climb the academic ladder (Long, 1992; Kyvik and Teigen, 1996; Lawrence, 2006; Abramo et al., 2009). Rørstad and Aksnes, meanwhile, have argued that women and men choose differently. Arguably overdetermining the agency of individual female scholars and underdetermining the impact of structural sexism and gender-stereotyped job allocation, they discussed some previous scholarship and argued that female scholars allocate more of their time to teaching and administrative tasks, while their male counterparts focus more on research and projects (Rørstad and Aksnes, 2015, p. 318).
Gender difference has been shown to have a significant impact in studies of scholarly communication and networking more broadly (Sugimoto et al., 2015a; Day et al., 2020). There, gender has a significant effect on network formation (Brass, 1985; Ibarra, 1992), with most female groups having either a significantly higher centrality (centrality as an indicator that identifies the most important nodes in a network graph) (Brass, 1985) or significantly lower centrality (Tharenou, 1999) than male groups. Kretschmer and Aguillo detected low participation of female scholars in collaborative activities (2005). More recent studies show that female scholars are more likely to be in and benefit from central positions in the co-authorship network. It has been argued that this is because of their stronger and confident characteristics that helped them to break gender publication barriers (Badar et al., 2013).
3. Methodology
Despite the paucity of gender disaggregated studies of DH author networks, we can draw on robust studies of other fields to inform the current research assumption and design.
As a method, co-authorship analysis features prominently in studies of academic disciplines (Cronin, 2005; Fagan et al., 2018; Köseoglu et al., 2018), and DH (e.g. Nyhan and Duke-Williams, 2014a; Tang et al., 2017). Co-authorship network studies can provide an in-depth synthesis of the collaboration network; for example, by uncovering its structure, patterns of connection, and mechanism of formation (Kumar, 2015, p. 55). Lundberg asserted that “analysing co-authored publications has become the standard way of measuring research collaborations” (Lundberg et al., 2006, p. 575). This positions co-authorship as a proxy for research collaboration, especially in academic publications (Ponomariov and Boardman, 2016, p. 1,939). This proxy can be detected as, “These collaborations leave digital footprints in the form of bibliography, which can be effectively tracked and evaluated.” (Kumar, 2015, p. 57). In DH, few studies have applied social network analysis to visualize the co-authorship structure, apart from De la Cruz et al., with a limited dataset (i.e. 178 Digital Humanities Quarterly articles) (De la Cruz et al., 2015). Indeed, co-authorship has been “operationalized” as a proxy of research collaboration for decades by academic evaluators and policy makers, not only because the bibliometric data is structured and available but also because it reflects an aspect of research collaboration (Melin and Persson, 1996), although some disagree (Kumar, 2018).
An edge of a given co-authorship network is formed by two scholars who co-authored an article. The scholars are nodes in the network while the co-authorship relation is the edge. The more scholars that co-author publications, the more nodes and edges, and the larger the network will be. Such a network provides an output-centric view of an academic community and reveals its many structural characteristics; for example, the level of connectivity and field development (macro aspect), central or peripheral scholars in that network (micro aspect). Different approaches are used in the study of co-authorship patterns, such as counting bilateral and multilateral co-authored papers, unique affiliations and regions (Adams and Gurney, 2018), and the percentage of multi-authored papers (Hudson, 1996). Most co-authorship studies in DH, e.g. (Spiro, 2009; Nyhan and Duke-Williams, 2014a), applied this approach by counting the percentage of multi-authored papers. This study applies co-authorship network analysis to examine bibliometric data and explores the social structure of the DH community.
Compared to author co-citation analysis (ACA), co-authorship network focuses more on investigating personal connections and social interactions, while ACA aims to study the knowledge structure and intellectual formation of a given field. A co-authorship network studies the personal relationships formed by both co-authors (i.e. bilateral link) indicating their two-way interpersonal communication and social connection; a citation, however, is often a one-way relationship (i.e. unilateral link) from the article to the cited publication from which they carry the influence (or knowledge flow) from the source publication to the citing article. In this way, the co-authorship network not only explores relatively newer author data and the knowledge front, but also focuses on scholarly communication and collaboration.
Although it does not directly link to a scholar's research productivity, appropriately counting the citations of co-authors is an important task. One of the main purposes of constructing a network of co-authorship is to study the scholarly community itself, instead of evaluating individuals. From this perspective, relationships and social structures are often more essential than measuring individual authorship and citations. Consequently, many co-authorship studies apply full counting to weigh the nodes. Even though the fractional counting approach is preferred by some bibliometric studies, e.g. (Zhao and Strotmann, 2008, 2011), the full counting approach pulls co-authorship clusters closer together on the network so the important nodes and bridges are easier to detect (Perianes-Rodriguez et al., 2016, p. 1,186). Also, the full counting method is easier to explain with integer weights, and it is especially useful when presenting results like networks as visual graphs.
Co-authorship based indicators are noted in many bibliometric studies to be but one index of collaboration (Ponds, 2009; Gazni et al., 2012). Uncritical use of the co-authorship data could lead to misinterpretations of scholarly collaboration activities and thus provide incorrect data for decision-making (Lundberg et al., 2006). Co-authorship data is also likely to be subject to more generally observed citation-gaming practices, e.g. (Baccini et al., 2019). In addition, different disciplines use different author naming conventions. This can impact on career trajectory, as in the case of “alphabetical discrimination” (Einav and Yariv, 2006). This paper, however, does not seek to differentiate the distinction among authors that are listed in different orders on the publications. Nevertheless, such measures should be used cautiously, as just one source of evidence on exploring the scholarly communication and collaboration.
3.1 Data collection
This paper selects all the authors that have published articles in three well-known Digital Humanities journals: Computers and the Humanities (CHum) 1966–2004, Digital Scholarship in the Humanities (DSH) formerly known as Literary and Linguistic Computing (LLC) 1986–2017, and Digital Humanities Quarterly (DHQ) 2007–2017. The publication period covers 52 years ranging from 1966 to 2017.
These three journals have been studied as representative data sources in empirical studies that analyzed bibliometric elements to discover the developments of DH terminologies, DH Invisible College, and DH collaboration patterns (Wang and Inaba, 2009, para. 3; Bowman et al., 2013; Nyhan and Duke-Williams, 2014a, p. 387), and, furthermore, we have also selected these journals because of our familiarity with them. The main data collection took place during the full month of January 2018. All the peer-reviewed articles published in the three journals were collected. Following previous practices, e.g. (Larivière et al., 2013; Nyhan and Duke-Williams, 2014a), content like editorials, reviews, erratum, and notes were excluded because they are generally not peer-reviewed, or considered original contributions to knowledge. Every author's full name, along with other metadata (e.g. article title, publication year, volume and issue number, cited references) were collected (see Figure 1).
In total, 2,527 articles were collected (1,035 from CHum, 1,195 from LLC/DSH, and 297 from DHQ). The period between 1985 and 2017 had around 80.7% of the collected articles; two 10-year periods in particular had the most articles: 1986 to 1995 (26.6%) and 2008 to 2017 (29.0%). The year 2017 had the highest number of publications, which was 135 (5.3%).
3.2 Data cleaning and author identification
Once publication data has been collected it must be cleaned before constructing the co-authorship networks. It is common to have multiple authors with the same last names (i.e. homonymy), or multiple names belonging to the same author (i.e. synonymy). Authors sharing a common surname is not something rare in an academic publication dataset, whether in the English language (Goodman et al., 2015) or other language contexts (Yu et al., 2011; Freeman and Huang, 2015).
For author name data cleaning, apart from manually detecting errors and duplications, we applied the Author Name Disambiguation method (Strotmann et al., 2009; Zhao and Strotmann, 2011, p. 120) to identify the same author with different name forms. A python script was applied to help with auto-detection. The basic logic of this method is that we recognize two names to be the same author if those two names are mutually compatible (e.g. “Kretzschmar, w.a” and “Kretzschmar jr., W.A”); they have common co-authors (e.g. “Kretzschmar, w.a” and “Nerbonne J.” had co-authored a paper, while “Kretzschmar jr., W.A” and “Nerbonne J.” also co-authored a paper); and they have not co-authored any paper in the dataset (e.g. no paper is co-authored by “Kretzschmar, w.a” and “Kretzschmar jr., W.A”).
Because many authors have a complete surname, and first or/and middle names given in initial form on publications (especially in older content of the three journals such as LLC publications before 2000), to avoid further author identification errors, we also manually checked authors full names to reduce the name disambiguation error.
Assigning gender is part of the further data collection procedure. In the current dataset, there are 3,382 authors and the analysis would be more accurate if authors provided their own gender information. A few researchers do use author-provided data for their linguistic analysis, e.g. (Goswami et al., 2009, p. 214). However, in the context of this research, it was not feasible for us to contact authors to ask for their data due to lack of resources. Furthermore, some authors are deceased or otherwise uncontactable.
To identify gender in such a large cohort of authors, some studies propose automatic approaches based on an author's use of language. For example, Rangel et al., analyzed the stylistic features in authors' English and Spanish written texts from social media and the frequency of their use of different grammatical categories (e.g. pronouns and verbs) to obtain their gender and age (Rangel and Rosso, 2013, p. 177; Rangel et al., 2018). These automatic gender identification approaches have attracted much academic attention for their potential in online forensic, security, and marketing studies to help identify fake profiles or senders of harassing messages (Fatima et al., 2017). Nevertheless, they are not suitable for identifying authors' gender in the current dataset for the lack of sufficient text (e.g. only article titles and abstracts are available). This is also certainly not applicable to articles that have multiple authors from different gender groups.
Some studies in DH have relied on “gender guessing” to assign gender through a combination of hand-coding and automated inference (Weingart et al., 2016, pp. 2,000–2,016). This current study takes such an effort a step further by applying a well-tested name-gender assignment method (Larivière et al., 2013; Sugimoto et al., 2015b). Three gender categories were created for assignment: “female”, “male”, and “unknown”. It is noted that some people are gender diverse, but the sources for that information are very limited, so this study follows the previous gender category convention (Rørstad and Aksnes, 2015, p. 321). Firstly, a given name list with gender information was developed based on the universal and country-specific name lists in Larivière and Sugimoto's studies. The country-specific names were applicable to authors from English, French, Korean, Lithuanian, Persian, Portuguese, Serbian, Ukrainian, Thai and Japanese -speaking countries, as well as authors in India. Chinese names, in addition, were mostly assigned gender by searching the internet and checking affiliation and personal pages as the lead author is a native Mandarin speaker. Besides this, author names from other regions employed the universal name list for gender assignment. If it was a unisex name, and there was no additional author information that could be found from the internet, the author was assigned to the “unknown” category.
3.3 Data analysis
From a descriptive statistics point of view, there are 2,527 articles in total, of which 960 are multi-authored articles, accounting for 38.0% of the total. Figures 2 and 3 present the proportion and percentage of co-authored articles over the 52-year period. There is a steady growth on both graphs, and multi-authored articles have accounted for the majority, especially after 2006, e.g. LLC/DSH in 2013 (69.5%), 2015 (66.1%), 2017 (70.2%). Although the level of co-authored articles does not fully represent the pattern of collaboration, it is one of the important indicators and can help us gain more understanding of DH academic collaboration.
However, perhaps the increase of co-authorship is not something unique to DH, e.g. (Nyhan and Duke-Williams, 2014b). One might then ask, what is the co-authorship pattern in DH, and what determinants can encourage such co-authorship? To explore this, our study aims to go beyond descriptive statistical analysis and employs network method to visualize the links within the DH communities.
This study counts the total number of publications to weigh the author node (section 3.3.1) and the number of co-authored publications for the edge (section 3.3.2). VOSviewer 1.6.7 and Gephi 0.9.2, have also been used for network construction. VOSviewer is able to handle large-scale datasets, and employs algorithms to optimize the label display and overlapping issue (van Eck and Waltman, 2010, p. 530). Gephi, a tool well-validated through many network studies, was used in this study for centrality measure.
3.3.1 Node
There are in total 4,623 authors who have published in CHum, LLC/DSH, and DHQ (1966–2017), and 3,382 unique authors have been identified. The mean number of authors per article is 1.83, and each author, on average, has a published article weighting of 0.75 (2,527 articles in total). The node has been weighted by counting the total number of articles that each author has published in the dataset (i.e. full publication count). The total number of publications does not allow an author's academic productivity to be assessed (Rørstad and Aksnes, 2015, p. 318), especially from a limited number of journals. It still, however, reflects the contributions of the author within the data range of this study.
Table 1 shows the top 38 most prolific authors (up to 8 publications) in this dataset, and the complete table can be found in Appendix A. The author names have been used as node labels, and the size of the node has been weighted by its number of articles.
3.3.2 Edge
Calculating the edges of the co-authorship is straight forward. Each edge represents a co-authorship link, and if any two authors co-author an article, then the value of their co-authorship edge increases by 1. The undirected edges of each pair of co-authors were calculated and the matrix structure constructed. The complete network dataset can be found in Appendix A.
3.3.3 Network visualisation
Among the 3,382 identified authors, only 661 (19.5%) were connected to the main network of co-authors presenting a “small world” with a small but strong set of them collaborating actively. John Burrows, who is the most cited scholar in the dataset, published 10 articles and co-authored one paper (with Hugh Craig in Chum: Burrows and Craig, 1994), but he is not connected to the main co-authorship network as he has no link to any node on the main network. Overall, 19.5% is comparatively lower than other disciplines when compared at similar scale, e.g. Medicine – 92% (Newman, 2001), Management and Organization – 45% (Acedo et al., 2006), and Sociology – 34.5% (Moody, 2004). Figure 4 below shows the co-authorship network with all the authors in the dataset.
According to Figure 4, the main network at the centre accounts for 19.5% of the nodes, and many smaller networks and individual authors are detached from the main network and scattered around. Most authors who published more than one article are connected to the main network, although some are disconnected, e.g. John Burrows, Gordon Dixon, Robert J. Valenza, Roberto Busa, reflecting the fact that they did not publish any multi-authored papers in this current dataset. However, it is axiomatic that the longer the time span over which we view a network, the more likely it is that network members active periods will not intersect. Figure 5 below shows the main co-authorship network.
3.3.4 Centrality
As shown in Figure 5, it is clear that some nodes are important bridges of the network, such as Susan Hockey, Julianne Nyhan, Melissa Terras, Edward Vanhoutte, and John Nerbonne. To better investigate the roles that individual scholars play in research collaboration as captured in co-citation metrics, it is beneficial to apply centrality measures. Studies have shown the promising aspects of centrality indices for analysing and interpreting co-authorship networks (De Stefano et al., 2011; Abbasi et al., 2012). This study has employed Gephi 0.9.2 to calculate the betweenness centrality for the network and results are displayed in Table 3 of the next section.
3.3.5 Longitudinal analysis
As reviewed, the proportion of authors who are connected to the main co-authorship network is very limited. DH, in particular, often forms “small world” models populated with a small number of authors (Grandjean, 2016). The spread of the co-authorship network depends heavily on the scale of the dataset, i.e. the number of publications. In other words, the more publications one collects, the more authors are likely to be connected to the main co-authorship network. Because of the low proportion of authors on the main network, when studying the network longitudinally, datasets can no longer be sliced into separate periods, but must be studied as a whole accumulated structure. Table 2 shows the percentage of authors who were connected during each separate period and how the number of connected nodes is very low if not combined.
As the very low numbers of connected authors within each separate period cannot provide useful information to interrogate the pattern of scholarly collaboration, this study has combined the periods and constructed an accumulated co-authorship network. By using different colors to code the average publication year of each author, Figure 6 below shows the accumulated longitudinal information of each co-author.
The color-coding of Figure 6 indicates the different positions that authors belong to at different times, and also reflects their collaboration trend over time. On the right-hand side, we can see that people who published articles before 1980 tend to be linked together. Nevertheless, we can also find some authors who are highly ranked in all time periods, e.g. Susan Hockey, indicating that her “career plateau” extends across time (Chang Boon Lee, 2003, p. 538). Some authors have been on the rise more recently (in orange and red), while others have faded out or away (in blue).
Among all 3,382 authors, men number 2,253 (66.6%) and women 976 (28.9%), while 153 are classified as unknown. This distribution has been gradually changing during the past 52 years. Figure 7 below shows the annual percentages of unique female and male authors, and we can see a clear rising trend in the percentage of female scholars, especially in recent years, although they are still the minority.
The gender distribution of the co-authorship network is similar to that of the total number of authors in the dataset. There are 187 female authors (28.3%) and 409 male authors (61.9%). Figure 8 below shows the co-authorship network color-coded with gender information.
Figure 8 shows that female scholars may account for less than 30% of authors overall, yet many of their nodes are functioning as important bridges that link different clusters. Studies have shown that female scholars are thought to know more about their networks and to be more active in terms of forming their collaboration networks (Brass, 1985).
4. Results and discussion
Our study shows a clear rising trend in the proportion of female researchers publishing in the specified journals, especially in recent years, though they remain in a minority. Figure 7 supports the results of most previous DH gender studies by showing an apparent imbalance between the proportion of female and male scholars, e.g. (Weingart, 2012, 2013, 2014, 2015, 2016b; Risam, 2015).
Our results also present unanticipated findings about the different collaboration patterns between male and female scholars in DH. Although the gender distribution of the co-authorship network is similar to the total number of authors in the dataset (187 female – 28.3% in the co-authorship subset, compared to 28.9% in the wider full dataset, and 409 male – 61.9% (66.6% in the full dataset), see above Figure 8), this raises interesting questions about the gender differences apparent in the DH co-authorship network.
Although female scholars account for less than 30%, many of their nodes are functioning as important bridges, linking different clusters that would otherwise be disconnected from the main network. For example, the node of Susan Hockey links a cluster on the bottom right of the network, which would otherwise be disconnected without its link to her. Many nodes of female scholars play this important bridging role, e.g. Susan Brown, Melissa Terras, Julianne Nyhan, Maxine Brown, Claire Warwick, Julia Flanders. Male scholars, on the other hand, are more visibly grouped at the end of each cluster, despite the fact that they are taking up the majority places and thus are more visible in general.
In order to quantify the bridging roles of female scholars, this study has calculated the betweenness centrality of each node (i.e. the average number of pairs that have the shortest paths passing through the node) to examine the network's structural prominence. It is usually understood that the higher the centrality, the more important the node (Koschützki et al., 2005), and in a co-authorship network, the greater the impact of a scholar in forming the network (Yan and Ding, 2009, p. 30). Table 3 below presents the gender of the top 20 authors ranked by betweenness centrality. Many are female scholars (see Appendix A for the complete table).
As shown in Table 3, the value of betweenness centrality indicates the average number of pairs that have the shortest paths through the node. Authors have to be connected to at least one person in order to be included. If the value is 0, it means no pair has the shortest path through this node.
According to the results, female scholars are significantly more important than their male counterparts in forming the co-authorship network, despite them being a numeric minority. There are nine female scholars and nine male scholars in the top 20, and the highest two authors are both female (i.e. Susan Hockey and Susan Brown). Although there are equal numbers of female and male authors in the top 20 table, one needs to keep in mind that female scholars account for less than 30% of the total number of authors, which means that if they were represented in the same proportion, there would be many more female scholars in the top ranked list.
In addition, among all 661 authors who are connected to the main network, the average betweenness centrality for female scholars is 10,647.0, while it is only 7,542.5 for male scholars. The female average is 41.2% higher than that of the male average, demonstrating that the positions of female scholars in the DH network are significantly more influential and critical than those of male scholars. Moreover, moving to a broader scale, among all the 3,382 authors in the current dataset, female scholars on average have 2.6 co-authors while male scholars have 2.5. This shows that, on average, women in DH have relatively more collaborators than men.
In general, the results suggest that female scholars may have encouraged more communications, built more collaborations, and contributed more to the formation of the DH co-authorship network than males during the years studied. Not only are they the main forces to maintain DH scholarly connections, they are the icebreakers that bridge isolated groups. This finding reveals an interesting aspect of the gender difference in the DH community formation. Although we know that female scholars are a minority of the DH community, until now, no studies have detected the significant contribution they make to building connections and encouraging collaboration from a statistical perspective. On the other hand, this finding is not too surprising when brought into conversation with the external literature.
Previous empirical studies have shown that, on average, women in academia have more collaborators than men (Bozeman and Gaughan, 2011, p. 1,393). Female scholars are understood to know more about their networks and to be more knowledgeable in terms of forming their collaboration networks (Brass, 1985). They tend to expand and further develop their individual connections beyond their own areas and specialties, and this can encourage a greater level of interdisciplinary collaboration (Leahey, 2006; Rhoten and Pfirman, 2007). As DH is thought to be more interdisciplinary than many Humanities disciplines, women in DH may have more opportunities to develop such connections and form more collaborative and diverse networks.
Many studies argue that women are better communicators than men. While not an uncontroversial claim, and whether they “are” better communicators or have, for example, been socialised in this way, this might be one of the reasons why female scholars in DH have a different pattern of collaborative behaviour than male scholars. In general, women present as more “expressive” and “tentative” in conversation, while men tend to present as more “assertive” and “power-hungry” (Basow and Rubenfeld, 2003, p. 183). Women are reported to see communication as a way to improve social connections and build relationships while men are reported to use language to express dominance and achieve goals (Leaper, 1991). Thus, many have argued that women tend to be more social in communications while men value their independence (Gilligan, 1993; Chodorow, 1999; Eagly, 2013). Kuhn and Villeval found that significantly more women than men chose teamwork over individual tasks during their experiments, and that women were more attracted to collaboration (Kuhn and Villeval, 2015, p. 115).
Moreover, in the academic environment, female scholars encounter many more difficulties than their male counterparts and this may enhance their appreciation of positive collaborative networks. Numerous studies of female scholars have shown that they have less support, face greater professional isolation and slower rates of promotion, and are more likely to leave the academia before gaining tenure than their male counterparts (Wasburn, 2007; Baker, 2010; Brüggmann and Groneberg, 2017; Oleschuk, 2020). In order to overcome these challenges, some female scholars develop exceptional communication skills and make good use of their extensive connections. Collaborations can greatly help female scholars to build networks and to navigate the academic environment, and co-authorship has influenced their productivity more notably than male scholars (Abramo et al., 2013).
In addition, because female scholars are often a minority of a scholarly community, they are thought to be more dependent on networks than their more numerous male colleagues (Badar et al., 2013). Studies have shown that female scholars not only benefit more from direct and indirect connections to a network, but they also benefit significantly from connecting disconnected others (Burt, 2005, pp. 30–39). Mehra et al., have pointed out that by connecting isolated or disconnected nodes, one can take on the role of “gatekeeper”, ultimately accessing more diverse sources and information that can be used to produce more beneficial outcomes (Mehra et al., 2001).
However, many motivations may be posited for an individual pursuit of research collaborations (Ponomariov and Boardman, 2016) and the above analysis offers only a few possible explanations. Other interpretations are possible. For example, some argue that policies to reduce the family-related barriers that impeded female scholars may finally be paying off (Bozeman and Gaughan, 2011, p. 1,399). Also, some research argued that male scholars are more likely to place an emphasis on building a solid reputation through independent publications at the beginning of their career, in order to achieve better collaborations later (Abramo et al., 2013, p. 811). Some also discount the validity of using gender as an analytical unit in this way, arguing that collaboration is less influenced by gender than it is by effective leadership and team commitments (Ingram and Parker, 2002).
5. Conclusion
The co-authorship analysis that is the basis of this paper allows researchers' networks, social connections, and collaboration patterns (Newman, 2004) to be studied at a higher level of resolution than previously attained in bibliometric studies of DH. This study visualized the co-authorship network of data drawn from three well-known DH journals; it analysed the network's co-author clusters; and it studied the prominence of gender over time on the network using the betweenness centrality method. Our results show that despite being in a minority, female scholars are significantly more important than their male counterparts in forming co-authorship networks as represented by the dataset to hand. Women in DH account for 30% of the total authors published by these journals, but they appear to have encouraged more communications, built more collaborations, and contributed more to the formation of the DH co-authorship network than males during the years studied. They appear to be the main forces driving the establishment and maintenance of DH scholarly connections; they also are the facilitators who bridge isolated groups.
Our findings are the first to reveal this collaborative aspect of gender difference in DH co-author community formation. This paper also provides new data, as well as a new analysis of the gender distribution and difference in DH, and it suggests new questions to be examined in future studies. For example, how do female scholars in DH form their collaboration and co-authorship connections? How do we encourage such collaboration in DH? Is it a coincidence that along with the growth in the proportion of female scholars, the proportion of co-authored papers is also increasing?
From a methodological perspective, this paper contributes to the development of author name and gender identification methods that can be used for future studies. To deal with homonymy and synonymy issues in the author dataset, this study has not only implemented the Author Name Disambiguation method (Strotmann et al., 2009; Zhao and Strotmann, 2011, p. 120) but also provided guidelines and examples to help with auto-detection. For assigning author gender, this study has practically compared different methods, demonstrated clear “name-gender assignment” steps, added contributions to identify East Asian names in the methodology, and focalized multi-lingual author name databases. By doing so, this study has taken existing efforts a step further and provided a systematic author name-gender identification methodological framework for future relevant studies. The next stage in the development of name-gender identification methodologies and tools should entail moving away from gender binaries so as to better accommodate diverse identifications with and representations of gender.
These findings do not need to be read in a positivist way only. They might prompt reflection on communities and individuals who are less-well represented by the co-authorship network explored here. They might prompt questions about how digital humanities, and academic disciplines more broadly, can better recognise the distinctive contributions of the different groups that coalesce into the wider community. Scholars in DH and related fields might also take this study as a reference when planning future collaborations and co-author publications. Universities, centres, journals as well as organisations could take gender differences into account when encouraging authors to collaborate and publish. Future network studies could also replicate this study in other fields and topics, as a useful comparison between different disciplines.
Figures
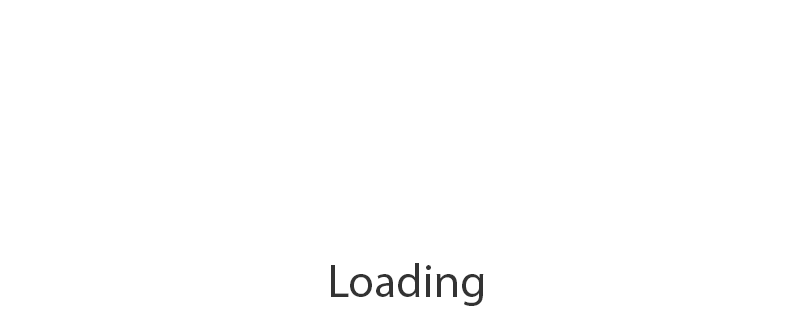
Figure 3
The percentage of multiple-authored articles each year in the collected dataset from the journals CHum, LLC/DSH, and DHQ (1966–2017). There is a steady growth on both graphs, and multi-authored articles have accounted for the majority, especially after 2006, e.g. LLC/DSH in 2013 (69.5%), 2015 (66.1%), 2017 (70.2%)
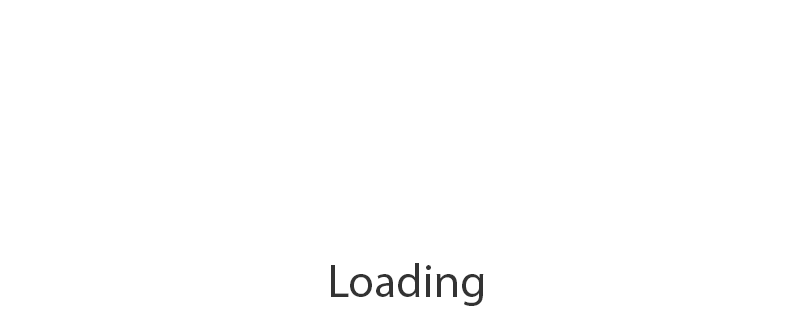
Figure 8
The co-authorship network with gender information, data from journals CHum, DSH/LLC and DHQ (1966–2017), graph created by VOSviewer. Although female scholars account for less than 30% of the total, many of their nodes are functioning as important bridges, linking different clusters that would otherwise be disconnected from the main network, and they have much higher betweenness centrality values (e.g. the nodes of Susan Hockey, Susan Brown, Melissa Terras, Julianne Nyhan, Maxine Brown, Claire Warwick, Julia Flanders)
The top 38 authors by the number of publications in journals CHum, LLC/DSH, and DHQ, 1966–2017
Author name | No. Articles | Author name | No. Articles | ||
---|---|---|---|---|---|
1 | Melissa Terras | 27 | 20 | Lisa Lena Opas-Hänninen | 10 |
2 | Susan Hockey | 22 | 21 | Stan Ruecker | 9 |
3 | MWA Smith | 20 | 22 | Julia Flanders | 9 |
4 | Mark Olsen | 17 | 23 | Whitney Bolton | 9 |
5 | John Nerbonne | 16 | 24 | Barron Brainerd | 9 |
6 | David Holmes | 16 | 25 | Raymond Siemens | 8 |
7 | Thomas N. Corns | 16 | 26 | Geoffrey Rockwell | 8 |
8 | Edward Vanhoutte | 15 | 27 | Michael Sperberg-McQueen | 8 |
9 | Willard McCarty | 14 | 28 | Tony McEnery | 8 |
10 | Peter Robinson | 14 | 29 | Gregory Crane | 8 |
11 | Julianne Nyhan | 13 | 30 | R L Widmann | 8 |
12 | Estelle Irizarry | 13 | 31 | Arianna Ciula | 8 |
13 | Susan Brown | 12 | 32 | William Kretzschmar | 8 |
14 | Fiona Tweedie | 12 | 33 | Susan Schreibman | 8 |
15 | Claire Warwick | 11 | 34 | Nancy Ide | 8 |
16 | John Bradley | 11 | 35 | G Lessard | 8 |
17 | Paul Fortier | 11 | 36 | Ellen Johnson | 8 |
18 | Lou Burnard | 11 | 37 | Matthew Spencer | 8 |
19 | Christopher Howe | 10 | 38 | WJ Jones | 8 |
The percentages of the connected authors during different periods of the co-authorship networks. Data extracted from journals CHum, LLC/DSH, and DHQ, 1966–2017
Time period | No. authors | No. connected authors | Percentage of connected authors |
---|---|---|---|
1966–1970 | 72 | 3 | 4.2% |
1971–1985 | 383 | 6 | 1.6% |
1986–1990 | 381 | 6 | 1.6% |
1991–2005 | 1,076 | 52 | 4.8% |
2006–2017 | 1,440 | 96 | 6.7% |
Whole period (1955–2017) | 3,382 | 661 | 19.6% |
The top 20 authors ranked by the highest betweenness centrality with gender information (F – female, M – male, U – unknown)
Author name | Publications | Co-authors | Gender | Betweenness centrality (average no. of pairs) | |
---|---|---|---|---|---|
1 | Susan Hockey | 22 | 16 | F | 81501.3 |
2 | Susan Brown | 12 | 25 | F | 68457.9 |
3 | SG Hall | 5 | 36 | U | 67345.3 |
4 | Raymond Siemens | 8 | 18 | M | 57718.0 |
5 | Melissa Terras | 27 | 53 | F | 49180.3 |
6 | Stan Ruecker | 9 | 31 | M | 48101.3 |
7 | Julianne Nyhan | 13 | 13 | F | 36533.6 |
8 | Geoffrey Rockwell | 8 | 23 | M | 34623.2 |
9 | Maxine Brown | 5 | 9 | F | 31533.1 |
10 | Paul Spence | 3 | 7 | M | 30916.8 |
11 | Claire Warwick | 11 | 23 | F | 30776.8 |
12 | John Nerbonne | 16 | 20 | M | 27324.3 |
13 | MWA Smith | 20 | 12 | U | 25812.2 |
14 | Michael Sperberg-McQueen | 8 | 8 | M | 24844.9 |
15 | Julia Flanders | 9 | 15 | F | 24471.7 |
16 | Hamish Cunningham | 5 | 19 | M | 24254.5 |
17 | Mark Olsen | 17 | 21 | M | 22831.9 |
18 | Tony McEnery | 8 | 21 | M | 22726.3 |
19 | Whitney Bolton | 9 | 9 | F | 22691.3 |
20 | Fiona Tweedie | 12 | 14 | F | 17960.0 |
Note
Examining journal publications in Computers and the Humanities (1966–2004) and Literary and Linguistic Computing (1986–2011), they found that, although single-authored papers predominated during this period, the number of authors who co-published was increasing.
Appendix is available online for this article.
References
Abbasi, A., Hossain, L. and Leydesdorff, L. (2012), “Betweenness centrality as a driver of preferential attachment in the evolution of research collaboration networks”, Journal of Informetrics, Vol. 6, pp. 403-412, doi: 10.1016/j.joi.2012.01.002.
Abramo, G., D'Angelo, C.A. and Caprasecca, A. (2009), “Gender differences in research productivity: a bibliometric analysis of the Italian academic system”, Scientometrics, Vol. 79, pp. 517-539, doi: 10.1007/s11192-007-2046-8.
Abramo, G., D'Angelo, C.A. and Murgia, G. (2013), “Gender differences in research collaboration”, Journal of Informetrics, Vol. 7, pp. 811-822, doi: 10.1016/j.joi.2013.07.002.
Acedo, F.J., Barroso, C. and Galan, J.L. (2006), “The resource-based theory: dissemination and main trends”, Strategic Management, Vol. 27, pp. 621-636, doi: 10.1002/smj.532.
Adams, J. and Gurney, K.A. (2018), “Bilateral and multilateral coauthorship and citation impact: patterns in UK and US International collaboration”, Frontiers in Research Metrics and Analytics, Vol. 3, p. 12, doi: 10.3389/frma.2018.00012.
Aksnes, D.W., Rorstad, K., Piro, F. and Sivertsen, G. (2011), “Are female researchers less cited? A large-scale study of Norwegian scientists”, Journal of the American Society for Information Science and Technology, Vol. 62, pp. 628-636, doi: 10.1002/asi.21486.
Baccini, A., De Nicolao, G. and Petrovich, E. (2019), “Citation gaming induced by bibliometric evaluation: a country-level comparative analysis”, PLoS ONE, Vol. 14, e0221212, doi: 10.1371/journal.pone.0221212.
Badar, K., Hite, J.M. and Badir, Y.F. (2013), “Examining the relationship of co-authorship network centrality and gender on academic research performance: the case of chemistry researchers in Pakistan”, Scientometrics, Vol. 94, pp. 755-775, doi: 10.1007/s11192-012-0764-z.
Bailey, M., Cong-Huyen, A., Lothian, A. and Phillips, A. (2016), “Reflections on a movement: #transformDH, growing up”, in Gold and Lauren (Eds), Debates in the Digital Humanities: 2016, University of Minnesota Press, Minneapolis London, doi: 10.5749/j.ctt1cn6thb.
Baker, M. (2010), “Career confidence and gendered expectations of academic promotion”, Journal of Sociology, Vol. 46, pp. 317-334, doi: 10.1177/1440783310371402.
Basow, S.A. and Rubenfeld, K. (2003), “‘Troubles talk’: effects of gender and gender-typing”, Sex Roles, Vol. 48, pp. 183-187, doi: 10.1023/A:1022411623948.
Bawden, D. and Robinson, L. (2012), An Introduction to Information Science, Facet, London, doi: 10.1002/asi.22885.
Bowman, T.D., Demarest, B., Weingart, S.B., Simpson, G.L., Larivière, V., Thelwall, M. and Sugimoto, C.R. (2013), Mapping DH through Heterogeneous Communicative Practices, Indiana University Bloomington, available at: URL http://did.ils.indiana.edu/dh/pdf/DH2013.MappingDH.pdf (accessed 10 March 2022).
Bozeman, B. and Gaughan, M. (2011), “How do men and women differ in research collaborations? An analysis of the collaborative motives and strategies of academic researchers”, Research Policy, Vol. 40, pp. 1393-1402, doi: 10.1016/j.respol.2011.07.002.
Brass, D.J. (1985), “Men's and women's networks: a study of interaction patterns and influence in an organization”, Academy of Management Journal, Vol. 28, pp. 327-343, doi: 10.5465/256204.
Brüggmann, D. and Groneberg, D.A. (2017), “An index to characterize female career promotion in academic medicine”, Journal of Occupational Medicine and Toxicology, Vol. 12, p. 18, doi: 10.1186/s12995-017-0164-7.
Burrows, J.F. and Craig, D.H. (1994), “Lyrical drama and the ‘turbid mountebanks’: styles of dialogue in romantic and renaissance tragedy”, Computers and the Humanities, Vol. 28, pp. 63-86, doi: 10.1007/BF01830688.
Burt, R.S. (2005), Brokerage and Closure: An Introduction to Social Capital, Clarendon Lectures in Management Studies Series, Oxford University Press, Oxford; New York.
Chang Boon Lee, P. (2003), “Going beyond career plateau: using professional plateau to account for work outcomes”, Journal of Management Development, Vol. 22, pp. 538-551, doi: 10.1108/02621710310478503.
Chodorow, N. (1999), The Reproduction of Mothering: Psychoanalysis and the Sociology of Gender: With a New Preface, University of California Press, Berkeley.
Cronin, B. (2005), The Hand of Science: Academic Writing and its Rewards, Scarecrow Press, Lanham, MD.
Day, A.E., Corbett, P. and Boyle, J. (2020), “Is there a gender gap in chemical sciences scholarly communication?”, Chemical Science, Vol. 11, pp. 2277-2301, doi: 10.1039/C9SC04090K.
De la Cruz, D.M., Kaupp, J., Kemman, M. and Lewis, K. (2015), “Mapping cultures in the big tent : multidisciplinary networks in the digital humanities quarterly”, in IVMOOC 2015. Presented at the IVMOOC 2015 - Visualizing the Digital Humanities Project.
De Stefano, D., Giordano, G. and Vitale, M.P. (2011), “Issues in the analysis of co-authorship networks”, Quality and Quantity, Vol. 45, pp. 1091-1107, doi: 10.1007/s11135-011-9493-2.
D'Ignazio, C. and Klein, L.F. (2020), Data Feminism, Strong Ideas Series, The MIT Press, Cambridge, Massachusetts.
Eagly, A.H. (2013), Sex Differences in Social Behavior: A Social-Role Interpretation, 1st ed., Psychology Press, New York, doi: 10.4324/9780203781906.
Einav, L. and Yariv, L. (2006), “What's in a surname? The effects of surname initials on academic success”, Journal of Economic Perspectives, Vol. 20, pp. 175-188, doi: 10.1257/089533006776526085.
Fagan, J.M., Eddens, K., Dolly, J., Vanderford, N., Weiss, H. and Levens, J. (2018), “Assessing research collaboration through co-authorship network analysis”, available at: https://www.ncbi.nlm.nih.gov/pmc/articles/PMC6703830 (accessed at 12 March 2022).
Fatima, M., Hasan, K., Anwar, S. and Nawab, R.M.A. (2017), “Multilingual author profiling on Facebook”, Information Processing and Management, Vol. 53, pp. 886-904, doi: 10.1016/j.ipm.2017.03.005.
Freeman, R.B. and Huang, W. (2015), “Collaborating with people like me: ethnic coauthorship within the United States”, Journal of Labor Economics, Vol. 33, pp. S289-S318, doi: 10.1086/678973.
Gao, J., Nyhan, J., Duke-Williams, O. and Mahony, S. (2018), “Visualising the digital humanities community: a comparison study between citation network and social network”, Paper Presented at the Digital Humanities 2018, Mexico City, Mexico.
Gazni, A. and Didegah, F. (2011), “Investigating different types of research collaboration and citation impact: a case study of Harvard University's publications”, Scientometrics, Vol. 87, pp. 251-265, doi: 10.1007/s11192-011-0343-8.
Gazni, A., Sugimoto, C.R. and Didegah, F. (2012), “Mapping world scientific collaboration: authors, institutions, and countries”, Journal of the American Society for Information Science and Technology, Vol. 63, pp. 323-335, doi: 10.1002/asi.21688.
Gilligan, C. (1993), In a Different Voice: Psychological Theory and Women's Development, Harvard University Press, Cambridge, Mass.
Goodman, A.C., Goodman, J., Goodman, L. and Goodman, S. (2015), “A few goodmen: surname-sharing economist coauthors”, Economic Inquiry, Vol. 53, pp. 1392-1395, doi: 10.1111/ecin.12167.
Gorska, A., Korzynski, P., Mazurek, G. and Pucciarelli, F. (2020), “The role of social media in scholarly collaboration: an enabler of International research team's activation?”, Journal of Global Information Technology Management, Vol. 23, pp. 273-291, doi: 10.1080/1097198X.2020.1817684.
Goswami, S., Sarkar, S. and Rustagi, M. (2009), “Stylometric analysis of bloggers' age and gender”, Presented at the the Third International ICWSM Conference (2009).
Grandjean, M. (2016), “A social network analysis of Twitter: mapping the digital humanities community”, Cogent Arts and Humanities, Vol. 3, p. 1171458, doi: 10.1080/23311983.2016.1171458.
Hudson, J. (1996), “Trends in multi-authored papers in economics”, Journal of Economic Perspectives, Vol. 10, pp. 153-158, doi: 10.1257/jep.10.3.153.
Ibarra, H. (1992), “Homophily and differential returns: sex differences in network structure and access in an advertising firm”, Administrative Science Quarterly, Vol. 37, pp. 422-447.
Ingram, S. and Parker, A. (2002), “Gender and modes of collaboration in an engineering classroom: a profile of two women on student teams”, Journal of Business and Technical Communication, Vol. 16, pp. 33-68, doi: 10.1177/1050651902016001002.
Joo, S., Hootman, J. and Katsurai, M. (2021), “Exploring the digital humanities research agenda: a text mining approach”, Journal of Documentation. doi: 10.1108/JD-03-2021-0066.
Köseoglu, M.A., Okumus, F., Putra, E.D., Yildiz, M. and Dogan, I.C. (2018), “Authorship trends, collaboration patterns, and co-authorship networks in lodging studies (1990-2016)”, Journal of Hospitality Marketing and Management, Vol. 27, pp. 561-582, doi: 10.1080/19368623.2018.1399192.
Koschützki, D., Lehmann, K.A., Peeters, L., Richter, S., Tenfelde-Podehl, D. and Zlotowski, O. (2005), “Centrality indices”, in Brandes, U. and Erlebach, T. (Eds), Network Analysis. Lecture Notes in Computer Science, Springer, Berlin, Heidelberg, Vol. 3418, pp. 16-61, doi: 10.1007/978-3-540-31955-9_3.
Kretschmer, H. and Aguillo, I.F. (2005), “New indicators for gender studies in Web networks”, Information Processing and Management, Vol. 41, pp. 1481-1494, doi: 10.1016/j.ipm.2005.03.009.
Kuhn, P. and Villeval, M.C. (2015), “Are women more attracted to Co-operation than men?”, The Economic Journal, Vol. 125, pp. 115-140, doi: 10.1111/ecoj.12122.
Kumar, S. (2015), “Co-authorship networks: a review of the literature”, Aslib Journal of Information Management, Vol. 67, pp. 55-73, doi: 10.1108/AJIM-09-2014-0116.
Kumar, S. (2018), “Ethical concerns in the rise of Co-authorship and its role as a proxy of research collaborations”, Publications, Vol. 6, p. 37, doi: 10.3390/publications6030037.
Kyvik, S. and Teigen, M. (1996), “Child care, research collaboration, and gender differences in scientific productivity”, Science, Technology, and Human Values, Vol. 21, pp. 54-71, doi: 10.1177/016224399602100103.
Larivière, V., Vignola-Gagné, E., Villeneuve, C., Gélinas, P. and Gingras, Y. (2011), “Sex differences in research funding, productivity and impact: an analysis of Québec university professors”, Scientometrics, Vol. 87, pp. 483-498, doi: 10.1007/s11192-011-0369-y.
Larivière, V., Ni, C., Gingras, Y., Cronin, B. and Sugimoto, C.R. (2013), “Bibliometrics: global gender disparities in science”, Nature News, Vol. 504, p. 211, doi: 10.1038/504211a.
Lawrence, P.A. (2006), “Men, women, and ghosts in science”, PLoS Biology, Vol. 4, e19, doi: 10.1371/journal.pbio.0040019.
Leahey, E. (2006), “Gender differences in productivity: research specialization as a missing link”, Gender and Society, Vol. 20, pp. 754-780, doi: 10.1177/0891243206293030.
Leaper, C. (1991), “Influence and involvement in children's discourse: age, gender, and partner effects”, Child Development, Vol. 62, p. 797, doi: 10.2307/1131178.
Lindqvist, A., Sendén, M.G. and Renström, E.A. (2020), “What is gender, anyway: a review of the options for operationalising gender”, Psychology and Sexuality, Vol. 12 No. 4, pp. 332-344, doi: 10.1080/19419899.2020.1729844.
Long, J.S. (1992), “Measures of sex differences in scientific productivity”, Social Forces, Vol. 71, pp. 159-178, doi: 10.1093/sf/71.1.159.
Lundberg, J., Tomson, G., Lundkvist, I., Skar, J. and Brommels, M. (2006), “Collaboration uncovered: exploring the adequacy of measuring university-industry collaboration through co-authorship and funding”, Scientometrics, Vol. 69, pp. 575-589, doi: 10.1007/s11192-006-0170-5.
Mehra, A., Kilduff, M. and Brass, D.J. (2001), “The social networks of high and low self-monitors: implications for workplace performance”, Administrative Science Quarterly, Vol. 46, p. 121, doi: 10.2307/2667127.
Melin, G. and Persson, O. (1996), “Studying research collaboration using co-authorships”, Scientometrics, Vol. 36, pp. 363-377, doi: 10.1007/BF02129600.
Moody, J. (2004), “The structure of a social science collaboration network: disciplinary cohesion from 1963 to 1999”, American Sociological Review, Vol. 69, pp. 213-238, doi: 10.1177/000312240406900204.
Mueller, C., Wright, R. and Girod, S. (2017), “The publication gender gap in US academic surgery”, BMC Surgery, Vol. 17, p. 16, doi: 10.1186/s12893-017-0211-4.
Mullaney, T.S., Peters, B., Hicks, M. and Philip, K. (Eds) (2021), Your Computer Is on Fire, The MIT Press, Cambridge, Massachusetts; London England.
National Science Foundation (2018), “2016 ‘doctorate recipients from U.S. Universities’”, available at: https://www.nsf.gov/statistics/2018/nsf18304/static/report/nsf18304-report.pdf (accessed 10 March 2022).
Newman, M.E.J. (2001), “The structure of scientific collaboration networks”, Proceedings of the National Academy of Sciences, Vol. 98, pp. 404-409, doi: 10.1073/pnas.98.2.404.
Newman, M.E.J. (2004), “Coauthorship networks and patterns of scientific collaboration”, PNAS, Vol. 101, pp. 5200-5205, doi: 10.1073/pnas.0307545100.
Noordenbos, G. (1992), “Explanations for differences in publication rates between male and female academics and between productive and less productive women”, Bulletin of Sociological Methodology/Bulletin de Méthodologie Sociologique, Vol. 35, pp. 22-45, doi: 10.1177/075910639203500102.
Nowviskie, B. (2015), “Resistance in the materials”, in Gold, M.K. and Klein, L.F. (Eds), Debates in the Digital Humanities 2016, University of Minnesota Press, 2016.
Nyhan, J. and Duke-Williams, O. (2014a), “Joint and multi-authored publication patterns in the digital humanities”, Literary and Linguistic Computing, Vol. 29, pp. 387-399, doi: 10.1093/llc/fqu018.
Nyhan, J. and Duke-Williams, O. (2014b), “Is digital humanities a collaborative discipline? Joint-authorship patterns clash with defining narrative”, available at: http://blogs.lse.ac.uk/impactofsocialsciences/2014/09/10/joint-authorship-digital-humanities-collaboration (accessed 20 March 2022).
Nyhan, J. and Terras, M. (2017), “Uncovering ‘hidden’ contributions to the history of Digital Humanities: the Index Thomisticus' female keypunch operators”, Presented at the Digital Humanities 2017, Montreal, Canada.
Oleschuk, M. (2020), “Gender equity considerations for tenure and promotion during COVID-19”, Canadian Review of Sociology/Revue canadienne de sociologie, Vol. 57, pp. 502-515, doi: 10.1111/cars.12295.
Perianes-Rodriguez, A., Waltman, L. and van Eck, N.J. (2016), “Constructing bibliometric networks: a comparison between full and fractional counting”, Journal of Informetrics, Vol. 10, pp. 1178-1195, doi: 10.1016/j.joi.2016.10.006.
Ponds, R. (2009), “The limits to internationalization of scientific research collaboration”, Journal of Technology Transfer, Vol. 34, pp. 76-94, doi: 10.1007/s10961-008-9083-1.
Ponomariov, B. and Boardman, C. (2016), “What is co-authorship?”, Scientometrics, Vol. 109, pp. 1939-1963, doi: 10.1007/s11192-016-2127-7.
Posner, M. (2015), “Selfies & feminism | selfies, snapchat, & cyberbullies”, available at: http://miriamposner.com/dh150w15/selfies-feminism/ (accessed 23 January 2019).
Prpić, K. (2002), “Gender and productivity differentials in science”, Scientometrics, Vol. 55, pp. 27-58, doi: 10.1023/A:1016046819457.
Rangel, F. and Rosso, P. (2013), “Use of language and author profiling: identification of gender and age”, Natural Language Processing and Cognitive Science, Vol. 2013, pp. 177-186.
Rangel, F., Rosso, P., Montes-y-Gómez, M., Potthast, M. and Stein, B. (2018), “Overview of the 6th author profiling task at pan 2018: multimodal gender identification in Twitter”, Working Notes Papers of the CLEF (2018), available at: file:///C:/Users/Lenovo/Downloads/129924.pdf (accessed 10 March 2022).
Rhoten, D. and Pfirman, S. (2007), “Women in interdisciplinary science: exploring preferences and consequences”, Research Policy, Vol. 36, pp. 56-75, doi: 10.1016/j.respol.2006.08.001.
Risam, R. (2015), “Introduction: gender, globalization and the digital”, Ada: A Journal of Gender, New Media, and Technology, No. 8, doi: 10.7264/N3RN364R.
Risam, R. (2018), New Digital Worlds: Postcolonial Digital Humanities in Theory, Praxis, and Pedagogy, Northwestern University Press, Evanston, Illinois.
Rørstad, K. and Aksnes, D.W. (2015), “Publication rate expressed by age, gender and academic position – a large-scale analysis of Norwegian academic staff”, Journal of Informetrics, Vol. 9, pp. 317-333, doi: 10.1016/j.joi.2015.02.003.
Savić, M., Ivanović, M. and Jain, L.C. (2019), “Co-authorship networks: an introduction”, in Complex Networks in Software, Knowledge, and Social Systems. Intelligent Systems Reference Library, Springer, Cham, Vol. 148, pp. 179-192, doi: 10.1007/978-3-319-91196-0_5.
Smeaton, A.F., Keogh, G., Gurrin, C., McDonald, K. and Sødring, T. (2002), “Analysis of papers from twenty-five years of SIGIR conferences: what have we been doing for the last quarter of a century?”, ACM SIGIR Forum, Vol. 36, p. 39, doi: 10.1145/792550.792556.
Smyth, H., Nyhan, J. and Flinn, A. (2020), “Opening the ‘black box’ of digital cultural heritage processes: feminist digital humanities and critical heritage studies”, in Schuster and Dunn (Eds), International Handbook of Research Methods in Digital Humanities, Routledge.
Spiro, L. (2009), “Collaborative authorship in the humanities”, Digital Scholarship in the Humanities, available at: https://digitalscholarship.wordpress.com/2009/04/21/collaborative-authorship-in-the-humanities (accessed 10 March 2022).
Strotmann, A., Zhao, D. and Bubela, T. (2009), “Author name disambiguation for collaboration network analysis and visualization”, Proceedings of the American Society for Information Science and Technology, Vol. 46, pp. 1-20, doi: 10.1002/meet.2009.1450460218.
Su, F. and Zhang, Y. (2021), “Research output, intellectual structures and contributors of digital humanities research: a longitudinal analysis 2005-2020”, Journal of Documentation, Vol. 78 No. 3, pp. 673-695, doi: 10.1108/JD-11-2020-0199.
Sugimoto, C.R., Ni, C. and Larivière, V. (2015a), “On the relationship between gender disparities in scholarly communication and country-level development indicators”, Science and Public Policy. Vol. scv007, doi: 10.1093/scipol/scv007.
Sugimoto, C.R., Ni, C., West, J.D. and Larivière, V. (2015b), “The academic advantage: gender disparities in patenting”, PLOS ONE, Vol. 10, e0128000, doi: 10.1371/journal.pone.0128000.
Symonds, M.R.E., Gemmell, N.J., Braisher, T.L., Gorringe, K.L. and Elgar, M.A. (2006), “Gender differences in publication output: towards an unbiased metric of research performance”, PLoS ONE, Vol. 1, e127, doi: 10.1371/journal.pone.0000127.
Tang, M.-C., Cheng, Y.J. and Chen, K.H. (2017), “A longitudinal study of intellectual cohesion in digital humanities using bibliometric analyses”, Scientometrics, Vol. 113, pp. 985-1008, doi: 10.1007/s11192-017-2496-6.
Tello, J.C. (2015), Visualizing Information about HDH2015 & EADH Day, CLIGS, available at: https://cligs.hypotheses.org/261 (accessed 10 March 2022).
Tharenou, P. (1999), “Gender differences in advancing to the top”, International Journal of Management Reviews, Vol. 1, pp. 111-132, doi: 10.1111/1468-2370.00008.
van Arensbergen, P., van der Weijden, I. and van den Besselaar, P. (2012), “Gender differences in scientific productivity: a persisting phenomenon?”, Scientometrics, Vol. 93, pp. 857-868, doi: 10.1007/s11192-012-0712-y.
van Eck, N.J. and Waltman, L. (2010), “Software survey: VOSviewer, a computer program for bibliometric mapping”, Scientometrics, Vol. 84, pp. 523-538, doi: 10.1007/s11192-009-0146-3.
Wang, X. and Inaba, M. (2009), “Analyzing structures and evolution of digital humanities based on correspondence analysis and co-word analysis”, Art Research, Vol. 9, pp. 123-134.
Wasburn, M.H. (2007), “Mentoring women faculty: an instrumental case study of strategic collaboration”, Mentoring and Tutoring: Partnership in Learning, Vol. 15, pp. 57-72, doi: 10.1080/13611260601037389.
Weingart, S.B. (2012), “Analyzing submissions to digital humanities 2013”, available at: http://scottbot.net/digital-humanities-2013-submission-analysis/ (accessed 10 March 2022).
Weingart, S.B. (2013), “Acceptances to digital humanities 2013 (part 1)”, available at: http://scottbot.net/analyzing-acceptances-to-digital-humanities-2013-part-1 (accessed 10 March 2022).
Weingart, S.B. (2014), “Submissions to digital humanities 2015 (pt. 3)”, available at: http://scottbot.net/submissions-to-digital-humanities-2014-pt-3 (accessed 10 March 2022).
Weingart, S.B. (2015), “Acceptances to digital humanities 2015 (part 4)”, available at: http://scottbot.net/acceptances-to-digital-humanities-2015-part-4 (accessed 10 March 2022).
Weingart, S.B. (2016a), “Representation at digital humanities conferences (2000-2015)”, available at: https://scottbot.net/representation-at-digital-humanities-conferences-2000-2015 (accessed 10 March 2022).
Weingart, S.B. (2016b), “Submissions to DH2016 (pt. 1)”, available at: http://www.scottbot.net/HIAL/index.html@p=41533.html (accessed 10 March 2022).
Weingart, S.B. and Eichmann-Kalwara, N. (2017), “What's under the big tent?: A study of ADHO conference abstracts”, Digital Studies/Le Champ Numérique, Vol. 7, doi: 10.16995/dscn.284.
Weingart, S.B., Eichmann-Kalwara, N. and Jorgensen, J. (2016), “Gender and centrality DH 2000-2016”, available at: http://scottbot.net/wp-content/uploads/2011/11/weingart-Gender-Centrality.pdf (accessed 10 March 2022).
Wernimont, J. (2013), “Whence feminism? Assessing feminist interventions in digital literary archives”, DHQ: Digital Humanities Quarterly, Vol. 7 No. 1, available at: http://www.digitalhumanities.org/dhq/vol/7/1/000156/000156.html (accessed 10 March 2022).
Wernimont, J. (2018), “Women in DH”, available at: https://docs.google.com/spreadsheets/d/1pPscJX7I7Vvuc4YIBbd38nbWgIjp-0FiI5yZ1sxG6Vk/edit#gid=0 (accessed 10 March 2022).
Wernimont, J. and Losh, E. (2018), “Wear and care feminisms at a long maker table”, in Sayers (Ed.), The Routledge Companion to Media Studies and Digital Humanities, Routledge, New York, NY, pp. 97-107, available at: https://scholarworks.wm.edu/asbookchapters/5.
Yan, E. and Ding, Y. (2009), “Applying centrality measures to impact analysis: a coauthorship network analysis”, Journal of the American Society for Information Science and Technology, Vol. 60, pp. 2107-2118, doi: 10.1002/asi.21128.
Yu, Q., Shao, H. and Duan, Z. (2011), “Research groups of oncology co-authorship network in China”, Scientometrics, Vol. 89, pp. 553-567, doi: 10.1007/s11192-011-0465-z.
Zhao, D. and Strotmann, A. (2008), “Comparing all-author and first-author co-citation analyses of information science”, Journal of Informetrics, Vol. 2, pp. 229-239, doi: 10.1016/j.joi.2008.05.004.
Zhao, D. and Strotmann, A. (2011), “Intellectual structure of stem cell research: a comprehensive author co-citation analysis of a highly collaborative and multidisciplinary field”, Scientometrics, Vol. 87, pp. 115-131, doi: 10.1007/s11192-010-0317-2.
Further reading
Gao, J., Duke-Williams, O., Mahony, S., Bold, M.R. and Nyhan, J. (2017), “The intellectual structure of digital humanities: an author Co-citation analysis”, Paper Presented at the Digital Humanities 2017, Montreal, Canada.
Kemman, M. (2018), “Boundary practices in digital humanities”, Paper Presented at the Digital Humanities Conference Benelux 2018, Amsterdam, The Netherlands.
Nyhan, J. and Flinn, A. (2016), Computation and the Humanities: towards an Oral History of Digital Humanities, Springer Berlin Heidelberg, New York, NY.
Acknowledgements
This work was supported by the Centre for Critical Heritage Studies, University College London and The UCL Overseas Research Scholarships (UCL-ORS)