Abstract
Purpose
In the last few years, the size of Linked Open Data (LOD) describing artworks, in general or domain-specific Knowledge Graphs (KGs), is gradually increasing. This provides (art-)historians and Cultural Heritage professionals with a wealth of information to explore. Specifically, structured data about iconographical and iconological (icon) aspects, i.e. information about the subjects, concepts and meanings of artworks, are extremely valuable for the state-of-the-art of computational tools, e.g. content recognition through computer vision. Nevertheless, a data quality evaluation for art domains, fundamental for data reuse, is still missing. The purpose of this study is filling this gap with an overview of art-historical data quality in current KGs with a focus on the icon aspects.
Design/methodology/approach
This study’s analyses are based on established KG evaluation methodologies, adapted to the domain by addressing requirements from art historians’ theories. The authors first select several KGs according to Semantic Web principles. Then, the authors evaluate (1) their structures’ suitability to describe icon information through quantitative and qualitative assessment and (2) their content, qualitatively assessed in terms of correctness and completeness.
Findings
This study’s results reveal several issues on the current expression of icon information in KGs. The content evaluation shows that these domain-specific statements are generally correct but often not complete. The incompleteness is confirmed by the structure evaluation, which highlights the unsuitability of the KG schemas to describe icon information with the required granularity.
Originality/value
The main contribution of this work is an overview of the actual landscape of the icon information expressed in LOD. Therefore, it is valuable to cultural institutions by providing them a first domain-specific data quality evaluation. Since this study’s results suggest that the selected domain information is underrepresented in Semantic Web datasets, the authors highlight the need for the creation and fostering of such information to provide a more thorough art-historical dimension to LOD.
Keywords
Citation
Baroncini, S., Sartini, B., Van Erp, M., Tomasi, F. and Gangemi, A. (2023), "Is dc:subject enough? A landscape on iconography and iconology statements of knowledge graphs in the semantic web", Journal of Documentation, Vol. 79 No. 7, pp. 115-136. https://doi.org/10.1108/JD-09-2022-0207
Publisher
:Emerald Publishing Limited
Copyright © 2023, Sofia Baroncini, Bruno Sartini, Marieke Van Erp, Francesca Tomasi and Aldo Gangemi
License
Published by Emerald Publishing Limited. This article is published under the Creative Commons Attribution (CC BY 4.0) licence. Anyone may reproduce, distribute, translate and create derivative works of this article (for both commercial and non-commercial purposes), subject to full attribution to the original publication and authors. The full terms of this licence may be seen at http://creativecommons.org/licences/by/4.0/legalcode
1. Introduction
Recent years have witnessed a growing interest in linked open data describing Cultural Heritage (Davis and Heravi, 2021). Despite many cultural institutions releasing their data only in a simple tabular form, several knowledge graphs (KGs) are addressing the description of artworks in a more structured, logical form [1]. Some of them, e.g. Wikidata (Vrandečić and Krötzsch, 2014), have a general scope and are created in a collaborative way, while others (e.g. ArCo (Carriero et al., 2019), Zeri and Lode (Daquino et al., 2017)), are generated by the conversion of authoritative data from cultural institutions.
In this diversified setting, it is important to assess the coverage, accuracy and reliability of the available data to allow their reuse for domain-specific purposes. While many studies addressed the problem of KG evaluation methods, to the authors’ knowledge, a survey on art history information stored in KGs, comprehensive of an assessment of the data quality, is still missing. Therefore, this work aims to evaluate the coverage of the content represented in visual works over existing KGs, with a focus on iconographical and iconological aspects (i.e. artistic subjects and their symbolic and cultural meanings). The phrase “iconographical and iconological” will be referred to as icon from now on. We survey KG evaluation methodologies and adapt some of their metrics to the considered domain of knowledge. Furthermore, theories concerning the icon domain are reviewed to assess the extent to which KGs cover information about visual items’ subject and content description.
Semantic web technologies offer an opportunity to formally express semantically complex information. For this reason, they are a suitable means to express fields of study as complex as iconography and iconology at the required granularity.
Artwork contents should be analysed both isolated, i.e. by identifying relevant features and associating them to features of other artworks (e.g. the study of patterns recurring in different subjects (Wittkower, 1987; Warburg, 1999)). Therefore, the knowledge emerging from an analytic approach is mostly missed when an artwork’s content is described just by a general subject term.
The traditional sources of knowledge are natural language descriptions of artworks as found in texts, but texts need knowledge extraction methods to enable further analysis and interlinking, limiting the computational reuse of that knowledge (Sartini and Gangemi, 2021).
Another problem is the lack of advanced ontologies [2] that provide a detailed semantic form to artwork description data. Only recently, a few ontologies have been designed to express icon features (Carboni and de Luca, 2019) and cultural symbolism (Sartini et al., 2021), opening the possibility to extract and represent KGs as required.
In addition, since iconographical–iconological analysis can potentially involve very different types of cultural objects, often stored by different institutions, the major benefits of storing information about this domain in KGs include at least:
The opportunity to answer domain-specific questions through quantitative analysis (e.g. which attributes and meanings were related to the mythological character of Mercury across the centuries?);
Accessing and querying interlinked information about worldwide objects that could not otherwise be experienced together (e.g. all artworks with political implications stored in different museums worldwide);
Formally expressing the semantic complexity of the topic (e.g. the levels of meanings of an artwork and its relations to external resources, such as other artworks, texts, etc.).
By providing curated and reliable semantic data about this domain, we aim to help traditional art historical research by offering new computational applications, pushing forward quantitative studies already conducted on the art history field (e.g. Greenwald, 2021).
Our main contribution is the assessment of the available data accuracy, reliability and interoperability in relation to the iconographical and iconological domain of knowledge. Therefore, the major benefit is to provide domain experts with a clear state of the quality of semantic, domain-specific data available online. Other benefits include improving current data reuse following LOD principles and fostering the creation of a shared semantic description framework for iconology and iconography. With this analysis, we show the reusability potential of the existing KGs based on defined icon requirements. Finally, the main findings of this work are shown in a landscape (Figure 1) in which KGs are positioned according to their performance in the chosen metrics.
This paper is structured as follows. In Section 2, we survey existing methodologies for KGs’ evaluations, followed by a comparison of theoretical models of artworks interpretation in Section 3. Section 4 describes the selected graphs, while Section 5 illustrates the evaluation method used. Finally, results are presented in Section 6, and Section 7 describes conclusions and future work.
2. State of the art in knowledge graph evaluation
KGs differ from traditional relational databases in their structure (graph versus table), the reasoning possibilities that can be applied to them, and facilitated interoperability and interconnections (Janev et al., 2020). These differences do require specific methods and metrics to evaluate them. Ji et al. (2022) survey evaluation metrics and methodologies for the tasks of representation learning, knowledge acquisition and completion, with additional analyses over temporal KGs and applications developed from them. Paulheim (2017) provides a series of refinements methods to increase the quality of KGs. Pellegrino et al. (2023) evaluates Cultural Heritage KGs in terms of their suitability for question answering tasks. Zaveri et al. (2016) proposes a conceptual framework for quantitative and qualitative metrics in the evaluation of KGs taken from a study of more than 100 scholarly publications. Various general metrics for knowledge graph quality evaluation and applications thereof are provided in Färber et al. (2018). We re-use parts of these metrics, adapting some to focus on the fields of iconography and iconology (see Section 5). Behkamal et al. (2014) present a similar study, but uses the goal-question metric paradigm to assess the quality of KGs. Ringler and Paulheim (2017) also compare several general domain KGs in their content coverage. It contains interesting reflections in particular regarding coverage of artistic fields, in which YAGO and DBpedia seem to be the most detailed. Heist et al. (2020) uses coverage as well as a metric for evaluation, although this work does not mention cultural heritage related findings. Shenoy et al. (2022) evaluates Wikidata on schema violations and deprecated entities, looking at its history of updates. Freire and Isaac (2020) also evaluate Wikidata’s completeness in the description of data related to cultural heritage. To do so, the information contained in it is compared with the information available on Europeana (Isaac and Haslhofer, 2013), which is used as a gold standard for completeness. This study does not mention specific aspects related to iconography and iconology. Issa et al. (2021) offers a thorough study on the completeness metric when evaluating KGs. Finally, Ruan et al. (2016) introduce the concepts of queriability to KGs, developing a framework for the evaluation of quality in use, applying it to DBpedia and YAGO. Queriability is a very interesting concept when it comes to extracting relatively complex sets of information from KGs, such complex relationships might be present in KGs that describe artworks with high granularity. Although to verify the queriability of the icon content, a first assessment on what is currently included in a knowledge graph is needed.
In summary, prior work evaluates KGs suitability for some automatic tasks, or their content, in terms of various metrics that go from completeness to accuracy to quality in use. Some of them focus on specific fields (like cultural heritage). There is no study yet that evaluates specific aspects related to iconology and iconography in KGs, which would require a specific evaluation due to the complexity of the information expressed by this domain of knowledge (Baroncini et al., 2021; Sartini et al., 2021). Therefore, the contribution of the current paper is to adapt a selection of the general metrics from the literature to the domain-specific needs, with the addition of a newly created metric. As a result, this contribution attempts to give a domain-specific overview of the available data quality according to the domain focus of interest and research questions.
3. Artwork descriptions and interpretations
Nowadays, several approaches for visual images interpretation are available, each considering different aspects (Rose, 2001). This variety is reflected in interpretation methodologies, which can focus on the objects themselves (formal aspects, content or materials), on the creator (psychoanalysis) or on the cultural context to which it belongs (Adams, 2010). Among them, content analysis and understanding are objects of interest in iconography and iconology. Although this field of study was traditionally limited to the interpretation of the artistic subject, the research of Aby Warburg (1889–1929) renewed it (Müller, 2014). His approach considered the content and forms of the artworks as witnesses of social memory, conducting his analysis in an interdisciplinary way to include religion, culture and the recurrence of visual patterns through different ages (Rossi Pinelli, 2019; Warburg, 1999). While iconography can currently be defined as the study of subjects, their attributes and their changes over time, the term iconology reflects Warburg’s approach, focussing on the socio-cultural interpretation of iconographical and formal variations (Baroncini et al., 2021, van Straten, 2012).
Although a methodology for artworks’ comprehension was considered by Warburg (Rampley, 1997), the prevailing theoretical approach in the discipline consists of the subdivision of the artwork’s interpretation into 3 or 4 levels, a framework firstly defined by Erwin Panofsky (Müller, 2014). We refer to Baroncini et al. (2021) for a comparison between the main theories which move from this first formalization attempt. For this study, we adopt Panofsky’s theory to evaluate the level of description of artworks in available graphs due to its historical relevance and as it is cited as a reference for subject description by the main cataloguing standards of the field [3]. However, aspects put forward by other art historians will be considered. Here, three layers are identified, namely pre-iconographical description, iconographical analysis and iconological interpretation. From the first level to the last one, increasing knowledge of conventions, sources and cultural aspects linked to the artwork production are required. When practically applied, the levels constituting the act of interpretation are simultaneous, and the interpretation itself is narrowly dependent on subjective intuition (Müller, 2014).
Firstly, objects such as people, actions, emotions, colours and shapes are recognized (level 1). Then, these objects are interpreted as subjects or iconographies (e.g. Mary) at the second level, which requires the knowledge of the literary sources and visual conventions used in a determined period and context. Then, the reading of iconographies as symptoms of the contemporary society, of the artist’s beliefs and personality or as the expression of meanings voluntarily inserted, is the content of the third level.
The levels of this theory are referenced by cataloguing standards for artworks description, such as the Getty’s Categories for the Description of Artworks (CDWA) [4] and the guide Cataloguing Cultural Objects (CCO) (Baca et al., 2006). Both of them underline that adopting a simplified description of the approach by Panofsky “can be helpful in indexing subjects for purposes of retrieval” [4], [5]. Following the alignment firstly proposed by Shatford (1986), they define the second and the third level, viz. the identification of themes, narratives, iconographies and meanings, as the aboutness (i.e. what the work is about), whereas the first level and eventually the second one are corresponding to the ofness (viz. what can be seen by a non-expert interpreter (Žumer et al., 2012, pp. 207–208; Klenczon and Rygiel, 2014)). If the subject corresponds to the work itself (e.g. the term architecture used for describing a cathedral) and does not refer to a subject depicted by the object (e.g. a drawing representing a cathedral), the term isness shall be used [4]. The concepts of ofness, aboutness and isness are a core aspect of knowledge organization initiatives (ISKO, IFLA) and further discussed in Zeng et al. (2009) and Hjorland (2016).
To illustrate our theory and present an example in which each level of interpretation is covered, we describe Michelangelo’s Tityus interpreted in Panofsky (1972). The drawing (Figure 2) shows a laying, naked man whose liver is being devoured by a vulture (level 1 ofness). It represents the story of Tityus (level 2, aboutness), punished by Apollo for having assaulted his mother Leto by chaining him to a rock in Hades while two vultures eternally devour his liver, considered the seat of physical passions (symbol, level 2, aboutness). The story had been commonly interpreted by Michelangelo’s contemporaries as an allegory of the tortures caused by immoderate love (allegory, level 2, aboutness). On this basis, Panofsky claims that the artist depicted this story as a symbol of his personal passion for Tommaso Cavalieri (level 3, aboutness), to whom he gifted a corpus of drawings pervaded by Neoplatonic meanings (level 3, aboutness). Table 1 shows how this interpretation can be subdivided into levels. For its completeness, this drawing will be considered as an example for artworks’ content and meaning evaluation in KG in Section 5.
4. Selection of the knowledge graphs
To collect the most representative RDF [6] data about the description of the artwork, we need to consider which kind of cultural objects can represent a visual subject and can have a cultural meaning. Potentially, every image representing a subject that can be invested with a cultural meaning can be considered by an iconographical–iconological interpretation. To narrow down the research in the art history field, we focus our selection on paintings, sculptures, frescoes, visual subjects on coins (numismatics) and illuminations. Therefore, in this survey, we considered graphs containing data on cultural heritage, museums, libraries (manuscripts’ drawings and decorations) and numismatics. In addition, we included general purpose KGs likely containing information about artworks such as Wikidata, DBpedia (Auer et al., 2007) and YAGO (Rebele et al., 2016).
We used the following methodology. We first define our object of interest, namely artworks and information about their subject and meaning. Then, we collect the KGs through (1) the analysis of literature concerning a survey or evaluation of CH KGs (Bikakis et al., 2021; Pellegrino et al., 2023; Savnik et al., 2021) and (2) direct search on the web, through a manual keyword search on Google Database Index [7] and other main databases search engines [8], [9], [10]. This led to 56 graphs. These graphs were further pruned according to the criteria of their online availability through a SPARQL endpoint [11]. We considered these criteria fundamental to assessing data that follows the principle of availability and re-usability of the Semantic Web (Wilkinson et al., 2016), according to its shared standards [12].
Only 27 out of 56 graphs were active online, 18 of which had a SPARQL endpoint. The KGs for which the SPARQL endpoint was not responsive and the ones having no information about subjects were discarded. Consequently, we obtained 9 graphs. Table 2 gives an overview of the number of artworks having a subject, distinguishing between Uniform Resource Identifiers (URIs) [13] and literals [14], [15]. This analysis was conducted through SPARQL queries and by consulting the KGs’ documentation. The selection process of our analysis highlights how information about cultural heritage is very scarce when considering data that follows Semantic Web principles, as few domain-specific KGs are available under those conditions. This makes the inclusion of general domain KGs essential to assess how icon aspects are described in the Semantic Web, as the majority of icon data in stored in them. From a structural perspective, we would expect the ontological schemas [16] of domain-specific KGs to describe icon information with a higher degree of granularity compared to general ones. This assumption is proved wrong by our results (section 6), as Wikidata performs better than domain-specific KGs.
One critical aspect we encountered while doing this analysis is the proper identification of what is a work of art. While some graphs use a specific class or property to express it (e.g. fabio: ArtisticWork in Zeri and Lode), others do not have a unique way to identify it. In some cases, e.g. Wikidata, many specific classes are used, subclasses of a general “visual work”. In others, e.g. SARI’s RDS platform, the class “Work” corresponds to many different types of cultural objects, specified by a controlled vocabulary. Although this granularity in the artwork description is appreciable, it may generate a few issues when approaching data quantitatively. First, the selection of what is considered an artwork is left to the user, who may be influenced by subjective decisions in this definition. Second, the high number of entities to be included in a SPARQL query can influence the server response.
In the context of this study, we selected which classes could be considered artworks from the analysis of the documentation or from data retrieval. We decided to focus our attention on paintings and sculptures, when available (if the information present in the KGs made them distinguishable from other artworks), as they are universally considered as artworks with at least a subject. When paintings and sculptures were not available in the studied knowledge graph, we shifted our attention to the most prominent class in the schema that could represent an artwork (as the numismatic items in Nomisma). On the other hand, when the total number of sculptures and paintings was too little for conducting an evaluation (e.g. in SARI’s RDS platform), we included in the analysis broader terms, such as prints, illustrations and graphics. Table 3 summarizes classes that define artworks from the selected KGs, along with properties used to link information relevant to iconography and iconology.
5. Evaluation criteria
Following the approach presented in Wang and Strong (1996), we define metrics that go beyond accuracy, as we are interested in (1) the coverage of the KGs schemas and their data, (2) the references and interlinking with existing taxonomies that identify subjects in art (Iconclass, Getty), (3) alignments and (4) linking to external KGs to foster poly-vocality in art interpretations. These general metrics were adapted for the evaluation of the specific domain of knowledge, to obtain a specific quality assessment on domain data. In addition, these metrics acquire a particular relevance for the domain studies, which analyse the relations between cultural objects, their sources and multiple interpretations. Following the theory explained in Section 3, we are interested in analysing whether the current KGs distinguish between elements that belong to the first, second and third level of interpretation. We are therefore looking for clear distinctions when it comes to the description of natural elements depicted in a painting, the recognition of subjects and symbols, and the reflections of the influence of the cultural period in which the artwork was created on the artwork itself and vice versa.
Taking this into consideration, we applied parts of the framework formulated in Färber et al. (2018) in the evaluation of the chosen KGs. This study proposes the possibility of a weighting system applied to each metric according to the importance of the task in the context of the evaluation. In our case, we give more weight to the evaluation criteria referring to the elements that were addressed the most in the literature of icon studies. Specifically, we assign the maximum weight (1) to those criteria that we consider completely related to iconography and iconology evaluation, 0.8 to those criteria that we consider closely related, and 0.6 to those criteria that we consider partially related. All other criteria are excluded; considering their weight would be 0, they were not computed. Therefore, of all the categories described by Färber et al. (2018), we focus only on column completeness, schema completeness, semantic validity, reference to external vocabularies and interlinking via owl:sameAs [17]. We adapted all metrics cited above to address the specific tasks of evaluation of the icon content. As a result of the adaptation, we decided to rename them to address their new specific purpose. Column completeness was changed into Iconographical and iconological column completeness (IICC), semantic validity became Semantic validity of iconographical and iconological triples (SVIIT) schema completeness became Iconographical and iconological schema granularity (IISG), reference to external vocabularies became References to external taxonomies of art and culture (RETAC) and Interlinking via owl:sameAs became Interlinking of artworks (IA). The differences and specific changes applied to these metrics will be explained in the sub-paragraphs of this section. Finally, we added an entirely new metric to measure intralinking potential for subject comparisons (IPSC).
Table 4 summarizes (1) the re-used metrics plus the newly created one, (2) their adaptation to the icon field and (3) the weight assigned to the metric. We applied these measurements to the KGs listed in Section 4. We then grouped these metrics in two macro-categories, namely (1) structure of the KGs, which includes IISG, IA, RETAC, IPSC and (2) content of the KGs, which includes SVIIT and IICC. The results of the analysis and the formulae used to calculate the overall score will be discussed in section 6.
5.1 Evaluation methodology
Of the chosen metrics, three (interlinking of artworks, references to external taxonomies of art and culture, and intralinking potential for subject comparisons) could be processed automatically by analysing the data, one through an analysis of the schemas of the various KGs (iconographical and iconological schema granularity), and two required qualitative evaluations (semantic validity of iconographical and iconological triples and iconographical and iconological column completeness). For all automatic evaluations, a series of SPARQL queries were launched on the analysed graph, and some will be listed as examples in the following subsections. For the metrics that required a qualitative evaluation of the content, we extracted random representative samples of the KGs and evaluated the graphs manually on those samples through annotations.
All annotations were performed by two annotators. In the annotation process, they could express their inability to evaluate the veracity of some of the triples if the information contained in the knowledge graph was unreachable (broken links) or too scarce to fully assess its quality. We used Cohen’s kappa (using quadratic weights) (Cohen, 1960) to measure the agreement score between the annotators. The triples considered invalid by annotators were mutually excluded when computing these agreement metrics [18]. Given the general agreements of the two annotators for all the different samples annotated, as shown in Table 5, we decided to average the evaluation scores of the two annotators for both the qualitative categories.
In the following paragraphs, the metrics and our computations to obtain them are described in natural language and their mathematical formulae.
5.2 Iconographical and iconological schema granularity
This metric is a re-elaboration of the “Schema completeness” metric in Färber et al. (2018).
Schema granularity aims to verify to what extent the ontologies and vocabularies, and corresponding classes and properties instantiated in the KGs, cover the domain of interest. In this work, we verify to what extent the schema of the knowledge graph is suited for the complete description of icon elements. Based on the comparison of theories of art interpretation discussed in section 3, we formulated the following competency questions (Uschold and Grüninger, 1996):
What are the pre-iconographical elements that appear in a work of art?
Which actions are depicted in a work of art?
What are the subjects of a work of art?
What are the represented symbols in a work of art?
What are the represented stories in a work of art?
What are the represented allegories in a work of art?
What are the intrinsic meanings associated with a work of art?
Which cultural phenomena are reflected in a work of art?
What are the corresponding external taxonomies for the identified iconographical terms?
We then created a gold standard interpretation on the example from Michelangelo’s work, able to answer those competency questions, as shown in Figure 3. We first aligned the properties used in each KG to our example and computed schema granularity as the division between the number of properties of the example that have been aligned, and the total number of properties in the example. Given N as the number of properties of the gold standard, and Nakg as the number of properties of the same gold standard aligned to the properties of the schema of the knowledge graph, we measure the IISG of a knowledge graph as
Table 6 shows those properties that were recognized as expressing icon content and were aligned to the gold standard.
We weigh this metric as 1 because a schema that permits to express icon statements, respecting the required granularity given by the complexity of their field, is essential to correctly and completely store information on this matter.
5.3 Semantic validity of iconographical and iconological triples
This metric was modified from the “Semantic Validity” of Färber et al. (2018), in which its purpose is to define whether all the statements of triples in KGs hold true or not. In our study, we consider the semantic validity of icon triples only: we evaluate whether triples that refer to a subject, depicted element or symbol associated with a painting hold true. To evaluate this, we take a subset of the icon statements in each KG. Those statements link the artwork to one of the elements relative to the three layers of interpretation explained in Section 3, agnostic to the property used. We compute this metric by taking a random sample of 100 iconographical/iconological triples from each knowledge graph, evaluating whether the triple is correct (1), partially correct (0.5) or wrong (0). Given Sictkg as the random set of iconographical triples extracted from a knowledge graph, Sevictkg as the evaluation scores set given for each triple {sc1, sc2 … scx} and x as the sample size [19] to be extracted from the knowledge graph, the SVIIT is measured as follows
This metric offers key insights on the quality of the icon content of KGs, and we give it a weight of 1.
5.4 Iconographical and iconological column completeness
This metric, in Färber et al. (2018), considers the general column completeness of KGs. In our work, we focus only on the column completeness of icon statements. Considering the potentiality expressed in a knowledge graph through the iconographical and iconological schema granularity, we evaluate the column completeness as the schema in use. We extract subgraphs from the analysed KGs that contain all the icon triples associated with 100 randomly selected artworks per KG. This evaluation considers two aspects:
the expected number of layers of an artwork. Generally, a landscape only contains elements belonging to the first layer, a portrait contains the first layer and then the identification of the subject (second layer), and more complex artworks that represent cultural and religious themes can also be analysed at a third, iconological level. Despite the potential for every visual image to have a deeper level of interpretation (van Straten, 2012), we decided to expect a third layer only in artworks presenting an explicit cultural subject. This is meant to not affect the artworks’ evaluation with the bias of over-interpretation, criticized by some scholars (Gombrich, 1948)
the number of layers covered by the current description in the knowledge graph.
We then divide the covered layers by the expected layers for each artwork in the subset. Having a maximum of three layers, the possible scores for each artwork can be 0 (0 covered layers out of 3 expected, 0/2, 0/1), 0.33 (1/3), 0.5 (1/2), 0.66 (2/3), 1 (1/1, 2/2, 3/3). We do not expect artworks to be described meticulously by indicating every single element of level 1, every single recognizable subject, allegory, symbol of level 2 and every single intrinsic meaning and culturally related meaning of level 3 [20]; for this evaluation, having at least one element for every expected level was considered enough. Given A as the set of the randomly sampled artworks in the knowledge graph of size x [21] {a1 … ax}, EL as the array of expected layers (a number from one to three) for each artwork
We consider this metric as important as having a schema that permits a certain degree of granularity in artwork descriptions; therefore we give it a weighing of 1.
5.5 Interlinking of artworks
We adapted the metric “Interlinking via owl:sameAs” described by Färber et al. (2018) to only apply to artworks. “Interlinking” is considered as the connection between entities belonging to different KGs. Although less central than the other used metrics (weight = 0.6), we decided to include it because aligning artworks across different KGs fosters poly-vocality in art interpretation, especially if these KGs have been manually curated [22]. We measure this metric by dividing the number of artworks in a knowledge graph that are connected to their corresponding versions in external KGs by the total number of artworks present in a knowledge graph. The main property used to align artwork across different KGs is owl:sameAs, but we also looked at other possible alignments from the analysed KGs [23].
Given KG as the set of triples {t1 … tn} in a knowledge graph (a triple being a sequence of subject, predicate, object {si, pj, ok}), A as the set of artworks {a1 … am} denoted by si or ok, and Ra as the set of relationships {r1 … rz} that are used to align an artwork in a knowledge graph to the same artwork in other KGs, we consider Aa = {a1 … aw} as a subset of A if
Two example queries launched on DBpedia to count the number of artworks and the number of artworks aligned to different KGs can be seen in listing 1 and 2, respectively.
SPARQL query launched on DBpedia to count the number of artworks
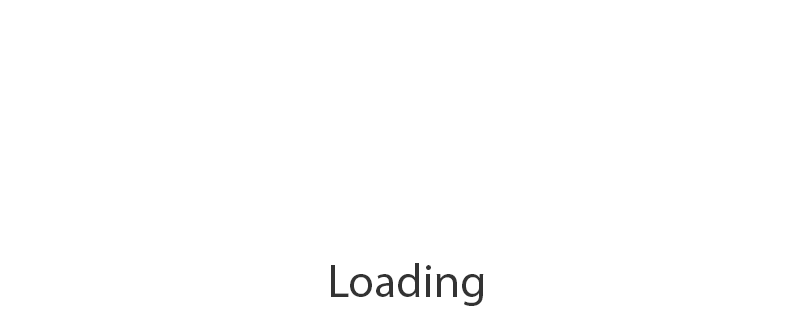
SPARQL query launched on DBpedia to count the number of artworks aligned to external KGs
5.6 References to external taxonomies of art and culture
This metric is a re-elaboration of the “Using external vocabulary” metric of Färber et al. (2018). In our work, we focus on the use of vocabulary that belongs to taxonomies of art and culture, which play an important role in artwork descriptions as they provide permanent URIs for specific subjects, scenes, and other icon elements represented in artworks. Moreover, they are curated by domain experts, and referring to them gives more authoritativeness to the interpretations. For this analysis, we selected four core taxonomies: Iconclass [24], the Getty Art and Architecture Thesaurus [25], the Getty Iconography Authority Vocabulary [26], and the Getty Cultural Object Name Authority Vocabulary [27]. We measure the references to external taxonomies of art and culture by dividing the number of artworks in a knowledge graph that are associated with at least one of them by the total number of artworks present. Given A as the set of artworks in and KG as the set of triples {t1, tn} in a knowledge graph (a triple being a sequence of subject, predicate, object {si, pj, ok}) and T as the set of nodes in a knowledge graph representing a particular subject expressed using a taxonomy of art and culture, we consider an artwork part of the subset At that contains artworks with a taxonomy reference if
The list of taxonomies of art and cultures used for this analysis contains only those that are referenced at least in one of the analysed KGs. Increasing the number of taxonomies referenced would not change the methodology of evaluation (and its formula). We welcome potential changes to this list to address icon aspects of more specific artworks, such as the reference to the Chinese Iconography Thesaurus [28] for a potential analysis on Chinese icon statements in the Semantic Web. References to external taxonomies are strictly related to iconography and iconology but are not essential to give a complete artwork description. For this reason, we weigh this metric 0.8.
The query shown in listing 3 was used to count all the artworks in ArCo referring to a taxonomy of art and culture (Iconclass).
SPARQL query launched on ArCo to count the artworks that have a reference to a taxonomy of art and culture (Iconclass)
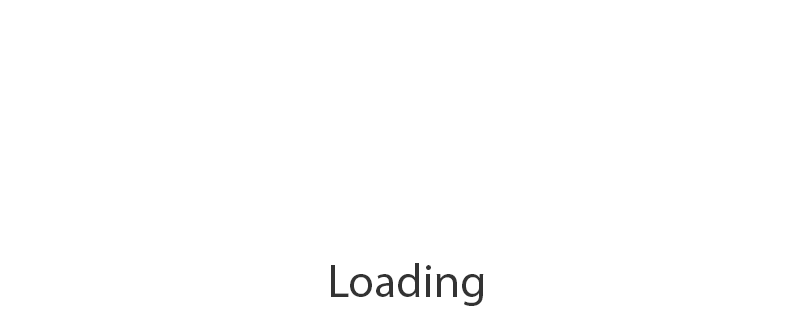
5.7 Intralinking potential for subject comparisons
We introduce this metric to highlight the importance of intralinking subjects in the same knowledge graph. We consider “intralinking” as the connection between entities belonging to the same knowledge graph. Having a URI as a subject of an artwork allows grouping artworks per subject and compares them in respect to having a subject as a literal. Moreover, the same subject can then be aligned to other subjects in different KGs, to foster interlinking in the digital art history LOD field. We measure intralinking potential for subject comparison by dividing the number of subjects that are linked to more than one artwork by the number of total subjects. Given S as the artistic subjects (expressed as URIs) in a knowledge graph and S2 as the artistic subjects that are linked to more than two artworks, we measure the intralinking potential for subject comparison (IPSC) of a knowledge graph as
As this aspect is relevant but not fundamental for iconographical content representation, we weight it 0.6. Two example queries that count the number of subjects (URIs) in Europeana and the number of subjects that are linked to more than one artwork can be seen, respectively, in listing 4 and 5.
SPARQL query launched on Europeana to count all the subjects that are URIs
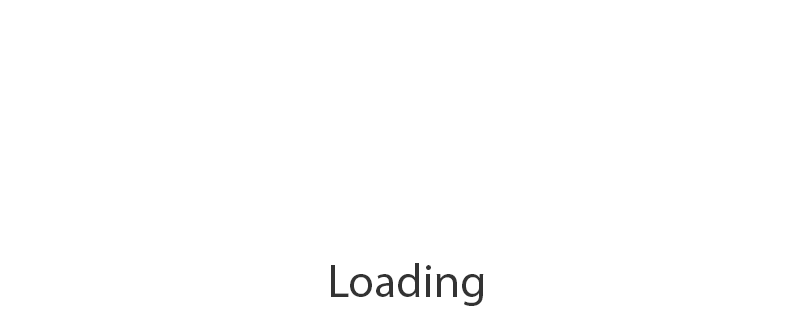
SPARQL query launched on Europeana to count all the subjects that are linked to more than one artwork
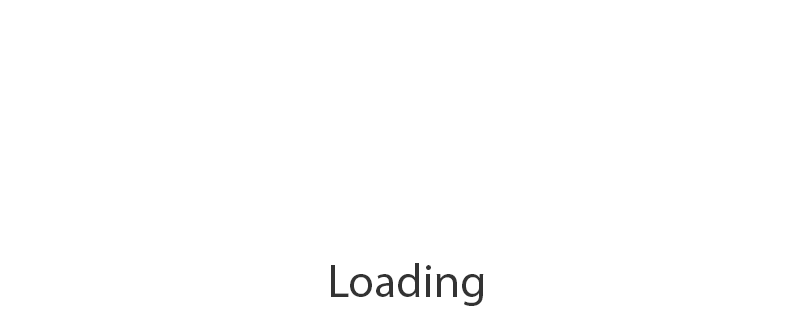
6. Results and discussion
Results obtained from the application of the metrics over the KGs are summarized in Table 7 and visualized in Figure 1. To give a better overview of the results of the metric evaluation, they were then used to place the KGs inside of a two-dimensional landscape. The landscape coordinates are determined by the two macro-aspects, namely content and structure, described in section 5. We averaged the metrics relative to these two macro-categories to obtain a score for content and structure. These averages are computed taking into consideration the weights of each metric. Given Ms and Mc as the sets of scores of a knowledge graph relative to its structure and content, respectively, {IISG, IA, RETAC, IPSC} and {SVIIT, IICC}, WMs and WMc as the sets of weights given to Ms and Mc, respectively, {wiisg, wia, wretac, wipsc} and {wsviit, wiicc}, we computed the structure score (SS) of a knowledge graph as follows
We divided the graphs in four categories, that represent the four quadrants of the landscape, according to their averaged scores, namely high in content and in structure (both scores
Figure 1 shows a clear scenario: the content of data is generally correct, but not thoroughly described. In fact, none of the graphs has acceptable results in the structure quadrants, and most of them (7 out of 9) present high scores in content. Nevertheless, this result is given by higher rates in semantic validity (six KGs score more than 0.8) rather than in column completeness (only 3 KGs score more than 0.7). Among them, despite being a general-purpose graph, Wikidata performs the best results. In fact, it has the best schema granularity, as several properties can be aligned to the prototype schema of Figure 3. In addition, its column completeness scores are higher than some art history graphs. This is because, in contrast with the approach adopted in the other graphs, the first level of interpretation is often described even when a second or third-level subject is identified.
The granularity in the levels’ description may have an influence on the intralinking metric, since the description of simpler and more generalizable elements of the first level of description can positively affect the capability of comparing artworks that share them. This assumption is evidenced by the fact that graphs such as SARI’s platform [29], where the subjects considered are broad concepts (e.g. “persons related to art”), perform better results in intralinking. Although, it is important to underline that the general purpose of the graph and the restricted number of subjects described can affect this evaluation. For example, Nomisma [30], having as subjects only deities, personifications or Roman emperors, performed the maximum score in this metric.
Other relevant qualitative observations can be made over the results obtained. Firstly, we envision that art history KGs such as Zeri&Lode, which precisely identifies second-level subjects with an acceptable percentage of interlinking to vocabularies, could foster subject retrieval and semantic computational capabilities by adding information on more levels of interpretation. Additionally, ArCo, created by automatic conversion of cultural heritage catalogues, despite having a high result in column completeness, has low rates in subjects intralinking (0.172) and in relation to external taxonomies (0.123). This may be due to the highly automatic process through which the knowledge graph was created (Carriero et al., 2019). The automatic creation of URIs for subjects from strings extracted from catalogue data could be improved to avoid duplicates of URIs referring to the same entities, therefore increasing the intralinking potential of the KG. For what concerns references to external taxonomies, Europeana shows the best results. In fact, it is possible to retrieve different types of artworks according to the Getty vocabulary category, allowing feasible reusability and retrieval of information for people knowledgeable about them. Moreover, by defining artwork types in this way, it is also possible to retrieve information without having to know specific classes for types of artworks, shifting from the necessity to know the specific schema of the KGs, to the knowledge of general taxonomies applicable to different linked open data datasets. It is interesting to note that, despite having a perfect score in references to taxonomies of art and culture, Europeana does not have any specific property that links an artwork to a taxonomy (it uses dc:subject) which decreased the score obtained in the schema granularity metric. Finally, the National Data Archive of Hungary (Fülöp et al., 2005) scores worst in the general categories, given the absence of subjects expressed as URIs, the only use of dc:subject to describe icon statements and the complete absence of references to taxonomies.
7. Conclusions and future work
To exploit the capabilities of interlinking, inference and analysis of the semantic technologies applied to icon study of artworks, reliable, complete and well-structured data are required. We assess the data quality of current CH KGs that are openly available, online queryable and having data on artwork subject descriptions. Our results indicate that only a few KGs describe the artwork’s iconography and iconology (Section 4). To assess their content according to different aspects, we adapt five metrics from prior KG evaluation methodologies (Section 5) and add a new metric. This set of metrics is used to evaluate the content and the structure of sub-graphs describing artworks’ icon characteristics. We observe that all KGs poorly perform in the schema structure as resulting from a combination of metrics, but the major part of them have high or acceptable scores for the content evaluation combined metric (Section 6).
This work gives a critical overview of the complexity involved in the correct and exhaustive creation of domain-specific data. Since the artwork icon descriptions are generally correct, the current data can be reliable for data reuse and analysis. Nevertheless, to enhance all the expressivity that may lay in them, a deeper accurate description and a better schema is required. Whereas icon descriptions exist, they are not sufficiently interlinked, searchable and exhaustively described. As a consequence, we recommend (1) a more extended reuse of existing domain-specific controlled vocabularies; (2) development of domain-specific ontologies that thoroughly cover iconography and iconology; and as a result of this, (3) either the creation of new domain data, formally expressed at a finer granularity, or the re-engineering of current data following newly developed ontologies. This recommendation is extended to current studies in the enhancement of iconographical cultural metadata, such as Bobasheva et al. (2022), which focus on adding new knowledge to artistic linked open data. As shown in this study, quantity and correctness of the data cover only one side of the coin. It is also important to express the newly generated knowledge with the correct schema that respects the granularity and complexity of iconography and iconology. Finally, from the general perspective of data quality assessment in a specific domain of knowledge, this evaluation can be considered as a case study, which can be generalized for spotting semantic representation issues in other domains.
Figures
Example of description of an artwork (Tityus, by Michelangelo) interpretation through three levels
Level | Description |
---|---|
1 | Nude, laying man, whose liver is devoured by a vulture |
2 | Tityus; story of Tityius, whose liver is devoured by a vulture; liver as the seat of physical passions; story of Tityus as an allegory of the tortures caused by immoderate love |
3 | Agonies of sensual passion, enslaving the soul and debasing it even beneath its normal terrestrial state according to the Neoplatonic theory; Expression of the agonies of sensual Passion that pervaded Michelangelo after he had met Tommaso Cavalieri, for whom he realized the drawing |
Source(s): Authors’ own creation
Overview of the artwork subject presence in the selected graphs*
Short name | Artwork # | Percentage of artworks having a subject (URI) | Average of subjects (URI) defined per artwork | Percentage of artworks having a subject (literal) | Average of subject (literal) defined per artwork |
---|---|---|---|---|---|
ArCo | 2,111,726 | 45.86% | 1.01 | 100 | 1.22 |
Fondazione Zeri | 20,082 | 99.99% | 1.19 | 0 | 0 |
Nomisma | 566,732 | 21.16% | 1.1 | 0 | 0 |
Wikidata | 669,857 | 26.76% | 3.37 | 0 | 0 |
SARI | 339 | 72.57% | 1.11 | 0 | 0 |
Europeana | 13,861 | 9.32% | 2.38 | 33.8 | 1.64 |
ND_Hungary | 11,655 | 0% | 0 | 55.97 | 6.04 |
DBpedia | 12,250 | 93.93% | 5.697 | 4.8 | 1.09 |
YAGO | 29,324 | 12.75% | 1.02 | 0 | 0 |
Note(s): *As of 01/12/2022
Source(s): Authors’ own creation
Classes and properties related to the recognition of artworks (sculptures and paintings if available) in selected knowledge graphs
Name (abbreviation) | Artwork (paintings and sculptures if possible) |
---|---|
ArCo | <artwork> a arco:HistoricOrArtisticProperty |
Zeri&Lode (Zeri) | <artwork> a fabio:ArtisticWork |
Nomisma | <artwork> nmo:hasObverse <something> |
<artwork> nmo:hasReverse <something> | |
Wikidata | <artwork> wdt:P31 wd:Q3305213 (Painting) |
<artwork> wdt:P31 wd:Q860861 (Sculpture) | |
RDS Platform (SARI) | <artwork> a gndo:Work |
<artwork> a gndo:formOfWorkAndExpression | |
Europeana | <artwork> a http://vocab.getty.edu/aat/300033618 |
<artwork> a http://vocab.getty.edu/aat/300047090 | |
National Digital Data Archive of Hungary (ND_Hungary) | <artwork> a dcmitype:Image |
DBpedia | <artwork> a dbo:Artwork |
YAGO | <artwork> a schema:Painting |
<artwork> a schema:Sculpture |
Source(s): Authors’ own creation
Evaluation metrics, the first five criteria are adapted from Färber et al. (2018), the last criterium is newly developed
Area | Criterium | Adaptation | Weight [0–1] |
---|---|---|---|
Content | Semantic Validity | Semantic Validity of Iconographical and Iconological Triples (SVIIT) | 1 |
Content | Column Completeness | Iconographical and Iconological Column Completeness (IICC) | 1 |
Structure | Schema Completeness | Iconographical and Iconological Schema Granularity (IISG) | 1 |
Structure | Using External Vocabulary | References to External Taxonomies of Art and Culture (RETAC) | 0.8 |
Structure | Interlinking via owl:sameAs | Interlinking of Artworks (via various properties) (IA) | 0.6 |
Structure | Intralinking Potential for Subject Comparisons (IPSC) | 0.6 |
Source(s): Authors’ own creation
Inter-annotator agreement scores as measured by quadratically weighted Cohen’s kappa for semantic validity of iconographical and iconological triples and column completeness per knowledge graph
Knowledge graph | Semantic validity | Column completeness |
---|---|---|
Yago | 1.00 | 0.65 |
Nd Hungary | 0.82 | 0.62 |
ArCo | 0.77 | 0.77 |
Zeri | 0.66 | 0.78 |
Nomisma | 1.00 | 1.00 |
Sari | 0.78 | 0.68 |
Europeana | 0.82 | 0.79 |
DBpedia | 0.89 | 0.66 |
Wikidata | 0.76 | 0.90 |
Source(s): Authors’ own creation
Properties identifying iconographical and iconological content for each selected knowledge graph
Name | Iconographic and iconologic properties |
---|---|
ARCO | arco-cd:hasSubject |
arco-dd:hasIconographicOrDecorativeApparatus | |
arco-cd:iconclassCode | |
arco-cd:subject | |
dc:subject | |
Zeri | fabio:hasSubjectTerm |
Nomisma | nmo:hasPortrait |
nmo:hasIconography | |
nmo:hasControlMark | |
Wikidata | wdt:P180 (depicts) |
wdt:P921 (main subject) | |
wdt:P1257 (depicts iconclass notation) | |
wdt:P4878 (symbolizes) (qualifier of wdt:P180) wdt:P6022 (expression, gesture or body pose) (qualifier of wdt:P180) | |
SARI | gndo:topic |
gndo:gndSubjectCategory | |
Europeana | dc:subject |
ND Hungary | dc:subject |
DBpedia | dc:subject |
dbp:subject | |
dbp:symbol | |
dbp:symbols | |
YAGO | schema:about |
Source(s): Authors’ own creation
Results for each metric over the selected knowledge graphs*
Short name | SVIIT (weight 1) | IICC (weight 1) | IISG (weight 1) | IA (weight 0.6) | IPSC (weight 0.6) | RETAC (weight 0.8) |
---|---|---|---|---|---|---|
ArCo | 0.8278 | 0.74 | 0.3333 | 0.0026 | 0.172 | 0.1238 |
Fondazione Zeri | 0.9925 | 0.5117 | 0.1111 | 0.0005 | 0.266 | 0.5449 |
Nomisma | 0.995 | 0.5 | 0.2222 | 0 | 0.749 | 0.0001 |
Wikidata | 0.9768 | 0.74 | 0.6667 | 0.699 | 0.367 | 0.157 |
SARI | 0.849 | 0.3783 | 0.1111 | 0.997 | 0.5 | 0 |
Europeana | 0.4688 | 0.236 | 0.1111 | 0.0073 | 0.6122 | 1 |
ND_Hungary | 0.13 | 0.5392 | 0.1111 | 0 | 0 | 0 |
DBpedia | 0.655 | 0.7242 | 0.2222 | 0.994 | 0.41 | 0 |
Yago | 0.99 | 0.4825 | 0.1111 | 1 | 0.1675 | 0 |
Note(s): *As of 01/12/2022
Source(s): Authors’ own creation
Notes
For a conceptual definition of a knowledge graph, we refer to Fensel et al. (2020):
KGs are very large semantic nets that integrate various and heterogeneous information sources to represent knowledge about certain domains of discourse.
On a technical level, we define KGs as sets of triples (subject, predicate, object) encoded in a serialization of the Resource Description Framework, or RDF (McBride, 2004)
An ontology is a formal representation of a domain, written using the logic-based language OWL (ontology web language). An ontology conceptualizes a domain by creating classes, properties (with corresponding logical axioms) that belong to that domain. For more information about ontologies and OWL, we refer to https://www.w3.org/TR/owl2-overview/
See the Categories of Description of Works of Art, available at https://www.getty.edu/research/publications/electronic_publications/cdwa/18subject.html and the guide CCO (Baca et al., 2006)
For the alignment of the concept of the subject matter to the main cataloguing standards, we refer to section 16 of Metadata Standard Crosswalk, available at https://www.getty.edu/research/publications/electronic_publications/intrometadata/crosswalks.html
RDF (Resource Description Framework) is a standard framework for describing resources (especially in the Semantic Web) using a subject-predicate-object model. We refer to https://www.w3.org/RDF/ for more information.
SPARQL is the query language that is used to retrieve information from RDF data. SPARQL endpoints are online services, linked to specific KGs, that let users query KGs through SPARQL queries. For additional information about SPARQL and SPARQL endpoints, we refer to https://www.w3.org/TR/rdf-sparql-query/
A URI , in a Semantic Web context, is the unique identifier for resources. URIs of resources can be semantically linked (using RDF properties) to other resources (and their URIs). For additional information on the concept of URIs, we refer to https://www.w3.org/TR/webarch/.
Literals represent basic data types, such as strings, boolean values, integers. They are not assigned a URI, and therefore they can only be referred to as the object of a triple and never as the subject. Literals contain unstructured information (such as natural language descriptions) that might require additional processing before being machine-readable. For additional information about literals, we refer to https://www.w3.org/TR/rdf11-concepts/
For an overview of the relations considered to identify subjects and other icon information, see section 5.2
We consider the ontological schema as the set of ontologies that are used in a knowledge graph as a data model. There exist several general domain schemas, such as Dublin Core https://www.dublincore.org/specifications/dublin-core/, Simple Knowledge Organization System (SKOS) https://www.w3.org/TR/skos-reference/, or Friend of a Friend (FOAF) http://xmlns.com/foaf/0.1/, that are reused in many different KGs. Domain-specific knowledge graph schema might include specifically developed ontologies in their schema, see the ArCo Ontology (https://w3id.org/arco/ontology/arco) for the ArCo knowledge graph or the Nomisma ontology (https://nomisma.org/ontology) for the Nomisma knowledge graph
The cited categories will be thoroughly explained in the following part of this section
Only 3.3% of total evaluated triples was considered invalid
In our case, set as 100
Especially considering that in the field of iconography and iconology, there could be potentially endless different interpretations of a painting, and it is not possible list them all
In our case, set as 100
We acknowledge that polyvocality can be achieved also by giving iconographical and iconological assertions a provenance (even in the same KGs), although for this work we only focus on statements agnostic to the provenance of the interpretation, which would require another specific study
The link to external artworks is expressed (1) in Europeana through the relations dc:relation or edm:relatedTo, (2) in Wikidata through different wikibase:identifier, (3) in ARCO and Zeri&Lode through rdfs:seeAlso, beyond owl:sameAs
The supplementary material for this article can be found online.
All the materials, including queries, Jupyter notebooks to compute the metrics’ scores and annotator’s evaluations are included in the following repository: https://github.com/SofiBar/ArtGraphsLandscapeAnalysis
Statement of responsibility
S. Baroncini is responsible for sections 1, 3, and 4. B. Sartini is responsible for sections 2 and 5. B. Sartini and S. Baroncini are responsible for section 6 and contributed equally to this research. All the authors are responsible for section 7.
References
Adams, L. (2010), The Methodologies of Art: An Introduction, 2nd ed., Westview Press. doi: 10.4324/9780429494444.
Auer, S., Bizer, C., Kobilarov, G., Lehmann, J., Cyganiak, R. and Ives, Z. (2007), “Dbpedia: a nucleus for a web of open data”, The Semantic Web, Springer, pp. 722-735.
Baca, M., Harping, P., Lanzi, E., McRae, L. and Whiteside, A. (2006), Cataloging Cultural Objects: A Guide to Describing Cultural Works and Their Images, American Library Association, Chicago.
Baroncini, S., Daquino, M. and Tomasi, F. (2021), “Modelling art interpretation and meaning. a data model for describing iconology and iconography”, available at: https://arxiv.org/abs/2106.12967
Behkamal, B., Kahani, M., Bagheri, E. and Jeremic, Z. (2014), “A metrics-driven approach for quality assessment of linked open data”, Journal of Theoretical and Applied Electronic Commerce Research, Vol. 9 No. 2, pp. 64-79.
Bikakis, A., Hyvönen, E., Jean, S., Markhoff, B. and Mosca, A. (2021), “Editorial: special issue on semantic web for cultural heritage”, Semantic Web, Vol. 12 No. 2, pp. 163-167.
Bobasheva, A., Gandon, F. and Precioso, F. (2022), “Learning and reasoning for cultural metadata quality: coupling symbolic ai and machine learning over a semantic web knowledge graph to support museum curators in improving the quality of cultural metadata and information retrieval”, Journal on Computing and Cultural Heritage, Vol. 15 No. 3, pp. 1-23, doi: 10.1145/3485844.
Carboni, N. and de Luca, L. (2019), “An ontological approach to the description of visual and iconographical representations”, Heritage, Vol. 2 No. 2, pp. 1191-1210, available at: https://doi.org/10.3390/heritage2020078
Carriero, V.A., Gangemi, A., Mancinelli, M.L., Marinucci, L., Nuzzolese, A.G., Presutti, V. and Veninata, C. (2019), “Arco: the Italian cultural heritage knowledge graph”, in Ghidini, C., Hartig, O., Maleshkova, M., Svátek, V., Cruz, I., Hogan, A., Song, J., Lefrançois, M. and Gandon, F. (Eds), The Semantic Web – ISWC 2019, Springer International Publishing, Cham, pp. 36-52.
Cohen, J. (1960), “A coefficient of agreement for nominal scales”, Educational and Psychological Measurement, Vol. 20 No. 1, pp. 37-46.
Daquino, M., Mambelli, F., Peroni, S., Tomasi, F. and Vitali, F. (2017), “Enhancing semantic expressivity in the cultural heritage domain: exposing the zeri photo archive as linked open data”, Journal on Computing and Cultural Heritage, Vol. 10 No. 4, doi: 10.1145/3051487.
Davis, E. and Heravi, B. (2021), “Linked data and cultural heritage: a systematic review of participation, collaboration, and motivation”, Journal on Computing and Cultural Heritage, Vol. 14 No. 2, pp. 1-18.
Färber, M., Bartscherer, F., Menne, C. and Rettinger, A. (2018), “Linked data quality of dbpedia, freebase, opencyc, wikidata, and yago”, Semantic Web, Vol. 9 No. 1, pp. 77-129.
Fensel, D., Şimşek, U., Angele, K., Huaman, E., Kärle, E., Panasiuk, O., Toma, I., Umbrich, J. and Wahler, A. (2020), Introduction: What Is a Knowledge Graph?, Springer International Publishing, Cham, pp. 1-10, doi: 10.1007/978-3-030-37439-6_1.
Freire, N. and Isaac, A. (2020), “Wikidata’s linked data for cultural heritage digital resources: an evaluation based on the Europeana data model”, International Conference on Dublin Core and Metadata Applications, pp. 59-68.
Fülöp, C., Kiss, G., Kovács, L. and Micsik, A. (2005), “Using a metadata schema registry in the national digital data archive of Hungary”, Research and Advanced Technology for Digital Libraries’, Lecture Notes in Computer Science, Springer Berlin Heidelberg, Berlin, Heidelberg, pp. 314-322.
Gombrich, E.H. (1948), “Icones symbolicae: the visual image in neo-platonic thought”, Journal of the Warburg and Courtauld Institutes, Vol. 11 No. 1, pp. 163-192.
Greenwald, D.S. (2021), Painting by Numbers, Princeton University Press, Princeton, NJ.
Heist, N., Hertling, S., Ringler, D. and Paulheim, H. (2020), “Knowledge graphs on the web - an overview”, Knowledge Graphs for eXplainable Artificial Intelligence.
Hjorland, B. (2016), in Hjørland, B. and Gnoli, C. (Eds), Subject (of Documents), ISKO Encyclopedia of Knowledge Organization. https://www.isko.org/cyclo/subject.
Isaac, A. and Haslhofer, B. (2013), “Europeana linked open data –data.europeana.eu”, Semantic Web, Vol. 4 No. 3, pp. 291-297.
Issa, S., Adekunle, O., Hamdi, F., Cherfi, S.S.-S., Dumontier, M. and Zaveri, A. (2021), “Knowledge graph completeness: a systematic literature review”, IEEE Access, Vol. 9, pp. 31322-31339.
Janev, V., Graux, D., Jabeen, H. and Sallinger, E. (2020), Knowledge Graphs and Big Data Processing, Springer Nature.
Ji, S., Pan, S., Cambria, E., Marttinen, P. and Yu, P.S. (2022), “A survey on knowledge graphs: representation, acquisition, and applications”, IEEE Transactions on Neural Networks and Learning Systems, Vol. 33 No. 2, pp. 494-514.
Klenczon, W. and Rygiel, P. (2014), “Librarian cornered by images, or how to index visual resources”, Cataloging and Classification Quarterly, Vol. 52 No. 1, pp. 42-61, doi: 10.1080/01639374.2013.848123.
McBride, B. (2004), The Resource Description Framework (RDF) and its Vocabulary Description Language RDFS, Springer Berlin Heidelberg, Berlin, Heidelberg, pp. 51-65, doi: 10.1007/978-3-540-24750-0_3.
Müller, M.G. (2014), “Iconography and iconology as a visual method and approach”, in The SAGE Handbook of Visual Research Methods, SAGE Publications, London, pp. 283-297.
Panofsky, E. (1972), Studies in Iconology: Humanistic Themes in the Art of the Renaissance, Westview Press, Boulder, CO.
Paulheim, H. (2017), “Knowledge graph refinement: a survey of approaches and evaluation methods”, Semantic Web, Vol. 8, pp. 489-508.
Pellegrino, M.A., Scarano, V. and Spagnuolo, C. (2023), “Move cultural heritage knowledge graphs in everyone’s pocket”, Semantic Web, Vol. 14 No. 2, pp. 323-359.
Rampley, M. (1997), “From symbol to allegory: Aby Warburg’s theory of art”, Art Bulletin, Vol. 79 No. 1, pp. 41-55.
Rebele, T., Suchanek, F., Hoffart, J., Biega, J., Kuzey, E. and Weikum, G. (2016), “Yago: a multilingual knowledge base from wikipedia, wordnet, and geonames”, International Semantic Web Conference, Springer, pp. 177-185.
Ringler, D. and Paulheim, H. (2017), “One knowledge graph to rule them all? Analyzing the differences between dbpedia, yago, wikidata & co”, Joint German/Austrian Conference on Artificial Intelligence (Künstliche Intelligenz), Springer, pp. 366-372.
Rose, G. (2001), Visual Methodologies, SAGE Publications, Thousand Oaks, CA.
Rossi Pinelli, O. (2019), La Storia Delle Storie Dell’arte, 3 edn, Einaudi, Turin.
Ruan, T., Li, Y., Wang, H. and Zhao, L. (2016), “From queriability to informativity, assessing ‘quality in use’ of dbpedia and yago”, European Semantic Web Conference, Springer, pp. 52-68.
Sartini, B. and Gangemi, A. (2021), “Towards the unchaining of symbolism from knowledge graphs: how symbolic relationships can link cultures”, Book of extended abstracts of the 10th national AIUCD conference, AIUCD, Pisa, pp. 576-580.
Sartini, B., van Erp, M. and Gangemi, A. (2021), “Marriage is a peach and a chalice: modelling cultural symbolism on the semantic web”, Proceedings of the 11th on Knowledge Capture Conference, K-CAP ‘21, Association for Computing Machinery, New York, NY, pp. 201-208, doi: 10.1145/3460210.3493552.
Savnik, I., Nitta, K., Skrekovski, R. and Augsten, N. (2021), “Statistics of knowledge graphs based on the conceptual schema”, CoRR abs/2109.09391, available at: https://arxiv.org/abs/2109.09391
Shatford, S. (1986), “Analyzing the subject of a picture: a theoretical approach”, Cataloging and Classification Quarterly, Vol. 6 No. 3, pp. 39-62, doi: 10.1300/J104v06n03_04.
Shenoy, K., Ilievski, F., Garijo, D., Schwabe, D. and Szekely, P. (2022), “A study of the quality of wikidata”, Journal of Web Semantics, Vol. 72, 100679.
Uschold, M. and Grüninger, M. (1996), “Ontologies: principles, methods and applications”, The Knowledge Engineering Review, Vol. 11.
van Straten, R. (2012), An Introduction to Iconography, 2nd ed., Routledge, London.
Vrandečić, D. and Krötzsch, M. (2014), “Wikidata: a free collaborative knowledgebase”, Communications of the ACM, Vol. 57 No. 10, pp. 78-85, doi: 10.1145/2629489.
Wang, R.Y. and Strong, D.M. (1996), “Beyond accuracy: what data quality means to data consumers”, Journal of Management Information Systems, Vol. 12 No. 4, pp. 5-33.
Warburg, A.M. (1999), The Renewal of Pagan Antiquity - Contributions to the Cultural History of the European Renaissance, Texts and Documents, Getty Publications, Los Angeles, CA.
Wilkinson, M.D., Dumontier, M., Aalbersberg, I.J., Appleton, G., Axton, M., Baak, A., Blomberg, N., Boiten, J.-W., da Silva Santos, L.B., Bourne, P.E., Bouwman, J., Brookes, A.J., Clark, T., Crosas, M., Dillo, I., Dumon, O., Edmunds, S., Evelo, C.T., Finkers, R., Gonzalez-Beltran, A., Gray, A.J.G., Groth, P., Goble, C., Grethe, J.S., Heringa, J., ‘t Hoen, P.A.C., Hooft, R., Kuhn, T., Kok, R., Kok, J., Lusher, S.J., Martone, M.E., Mons, A., Packer, A.L., Persson, B., Rocca-Serra, P., Roos, M., van Schaik, R., Sansone, S.-A., Schultes, E., Sengstag, T., Slater, T., Strawn, G., Swertz, M.A., Thompson, M., van der Lei, J., van Mulligen, E., Velterop, J., Waagmeester, A., Wittenburg, P., Wolstencroft, K., Zhao, J. and Mons, B. (2016), “The FAIR Guiding Principles for scientific data management and stewardship”, Scientific Data, Nature Publishing Group, Vol. 3 No. 1, 160018, available at: https://www.nature.com/articles/sdata201618
Wittkower, R. (1987), Allegory and the Migration of Symbols, Thames & Hudson, London.
Zaveri, A., Rula, A., Maurino, A., Pietrobon, R., Lehmann, J. and Auer, S. (2016), “Quality assessment for linked data: a survey”, Semantic Web, Vol. 7 No. 1, pp. 63-93.
Zeng, M.L., Žumer, M. and Salaba, A. (2009), “Functional requirements for subject authority data (FRSAD): a conceptual model”, in Bates, M.J. and Maack, M.N. (Eds), Encyclopedia of Library and Information Sciences, 3rd ed., CRC Press, pp. 1-16, 0 ed, available at: https://www.taylorfrancis.com/books/9781000031805/chapters/10.1081/E-ELIS3-120049494
Žumer, M., Zeng, M.L. and Salaba, A. (2012), FRSAD: Conceptual Modeling of Aboutness, Libraries Unlimited, Santa Barbara, California.
Acknowledgements
This work has been partially funded by the Emilia Romagna Region (grant agreement no. 462 25/03/2019), the University of Bologna, and the SPICE EU H2020 Project 870811 within the program: SOCIETAL CHALLENGES - Europe In A Changing World - Inclusive, Innovative And Reflective Societies.