Abstract
Purpose
This study examines the role of logotypes in advertising effectiveness on s-commerce platforms by analyzing the visual attention paid by the consumer to fashion branding – wordmarks or combination marks – and their subsequent recall.
Design/methodology/approach
The study examines the main areas of visual representation of the brand (VRB) on the Instagram network and the user’s corresponding areas of interest on a mobile-device screen. Attention and recall of the VRB are assessed in light of different classification variables (users’ gender, age and level of experience in s-commerce tools) to better understand how VRB may be leveraged by fashion retailers to encourage purchasing behavior. To achieve this objective, a mixed experiment design based on the eye-tracking methodology and a self-administered questionnaire is carried out.
Findings
The results indicate that visual attention, gender, age and s-commerce experience all contribute to determining users’ recall of the brand logo to which they are exposed on-screen. By considering the different s-commerce user profiles that exhibit different visualization behaviors, fashion retailers will be better placed to improve their online advertising campaigns and, ultimately, increase brand sales. The findings also point to promising future research directions on the effectiveness of branding strategies.
Originality/value
This highly innovative study provides in-depth insights into advertising effectiveness in terms of attention and recall, according to the main types of VRB for two specific s-commerce tools used by a high-street fashion brand, namely, its profile on Instagram Shop and its profile on Instagram Stories.
Keywords
Citation
Muñoz-Leiva, F., Herzallah, D., Sánchez-Borrego, I.R. and Liébana-Cabanillas, F. (2024), "Surprise me with the visual representation of the brand in social commerce! An eye-tracking study based on user characteristics", European Journal of Management and Business Economics, Vol. ahead-of-print No. ahead-of-print. https://doi.org/10.1108/EJMBE-03-2024-0090
Publisher
:Emerald Publishing Limited
Copyright © 2024, Francisco Muñoz-Leiva, Doaa Herzallah, Ismael Ramón Sánchez-Borrego and Francisco Liébana-Cabanillas
License
Published in European Journal of Management and Business Economics. Published by Emerald Publishing Limited.This article is published under the Creative Commons Attribution (CC BY 4.0) licence. Anyone mayreproduce, distribute, translate and create derivative works of this article (for both commercial and noncommercialpurposes), subject to full attribution to the original publication and authors. The full termsof this licence may be seen at http://creativecommons.org/licences/by/4.0/legalcode
1. Introduction
A growing number of businesses are now using social networks to promote their brands, sell their products, and extend their reach (Molina-Prados et al., 2021). In 2023 alone, brands spent approximately US$270 billion on social media advertising (Nepal, 2024). Global sales generated via social networks were forecast to reach US$992 billion by 2022, marking a growth trend in this form of digital retail. Projections indicate that social commerce (s-commerce) sales are poised to soar to nearly US$2.9 trillion by 2026. S-commerce integrates e-commerce and social media, with its main idea centered on executing various commercial activities through online social networks (Shamim et al., 2024; Herzallah et al., 2024).
According to McLachlan (2020), 60% of individuals discover new products thanks to Instagram. Instagram Shopping has the highest average order value (US$65) of the four largest s-commerce platforms (rivaling Facebook, Pinterest, and Twitter) (Barnhart, 2021). Social networks facilitate the personalization of ads based on user profiles and are also able to increase engagement with the advertised brand. This helps build brand loyalty and enables marketers to reach their audiences more effectively (Lin et al., 2019).
All forms of online advertising, whether static or dynamic, include various types of logos, such as combination marks, wordmarks, imagotypes, and so on, as part of the visual representation of the brand (VRB) in question. The VRB needs to effectively attract the consumer’s attention, such that they remember it, whatever the medium, and this is an increasingly important objective for firms. Understanding how consumers’ attention can best be attracted and how this affects their behavior—particularly in the context of social media— thus constitutes a topical research gap (Badenes-Rocha et al., 2022).
Consequently, it is vital to measure the effectiveness of social network advertising, and especially the VRB, amid the intense competition for user attention and the online saturation that such advertising creates. Previous studies (e.g. Barreto, 2013; Muñoz-Leiva et al., 2019; Hernández-Méndez and Muñoz-Leiva, 2015; Badenes-Rocha et al., 2022) have attempted to determine whether social network users actually pay attention to the paid online ads to which they are exposed, or whether such advertising is ineffective due to so-called “banner blindness”.
Yet, despite the rise of s-commerce, very few academic studies, to date, have evaluated advertising effectiveness in s-commerce—specifically in terms of the efficacy of different types of logos—in the shopping process conducted via mobile devices; and, it has yet to attract significant research interest in the fashion industry context. The goal of this study is therefore to identify the effects of certain socio-demographic variables and extent of user experience in s-commerce platforms on the advertising effectiveness of the VRB on social networks in this industry.
The present article aims to address this research gap through the following contributions: (a) providing a deeper and more comprehensive insight into the advertising effectiveness of the VRB (specifically, wordmarks and combination marks) for two specific s-commerce tools; (b) hypothesizing and empirically examining the influence of attention metrics, certain socio-demographic variables (such as gender and age), and extent of experience in using these s-commerce platforms on VRB recall; and, to achieve the three foregoing aims, (c) conducting an experiment using an infrared corneal reflection eye-tracking methodology combined with a self-administered questionnaire.
2. Theoretical background: justification of the research hypotheses
2.1 Logos: visual representation used in branding
The brand mark or logo plays a pivotal role in product and brand identification. Brand marks, featured in various channels and formats, serve as visual cues that aid in-store recognition, streamlining product selection and retrieval (Morrow, 1992). Additionally, branded giveaways such as pens or T-shirts often feature nothing but the company logo, highlighting the brand identity (Klink, 2003).
While the term “logo” is used generically, in fact, there are multiple logo formats, each with its own unique characteristics. Logos can be categorized into different types, including (Dolah et al., 2021):
- (1)
Lettermark/monogram logos: acronyms or letters that indirectly allude to the full name of the business (e.g. HBO).
- (2)
Wordmarks: represent the full business name, product/service, or institution, without acronyms (e.g. Home Box Office).
- (3)
Combination marks/isologos: integrate symbols, words, images, or letters into a single inseparable image (e.g. Burger King).
- (4)
Imagotypes: combine pictorial and textual components that can be used—and identified by consumers—separately (e.g. Nike).
This study seeks to identify the main determinants of recall—including visual attention—toward different types of logos pertaining to a well-known fashion brand. To fulfill this aim, it is first necessary to understand the process by which attention is captured and then transferred to the VRB.
2.2 The process of attention capture and transfer in s-commerce
Visual attention, memory, and recall are the mental processes that have attracted the most interest among studies that apply neuromarketing tools in the context of communication, along with emotions (Casado-Aranda et al., 2023).
The proposed conceptual model in which the present study is contextualized is shown in Figure 1; it draws on preexisting information-processing and persuasion models, such as the Elaboration Likelihood Model (ELM) proposed by Petty and Cacioppo (1986), and Pieters and Wedel’s (2004) Attention Capture and Transfer by Elements of Advertisements (AC-TEA) model. This model helps us understand how individuals allocate their visual attention vis-à-vis different elements of the VRB in s-commerce tools, and how this attention translates into recall (see the mid-section of Figure 1).
Visual attention is a limited resource that the consumer must distribute among different stimuli present in the same medium. “Attention”, in psychology, is defined as “the concentration of awareness on some phenomenon to the exclusion of other stimuli” (McCallum, 2024). According to Pieters and Wedel (2004), “[a]ttention capture enables higher-order cognitive functions to operate on more parsimonious and salient input” (p. 38, referencing LaBerge, 1995). Indeed, the previous literature has demonstrated that the effect of the consumer’s goals on their behavior, their past experiences, their level of involvement with the object, their attitudes, and their emotions all usually derive from the higher cortical centers (Snyder et al., 1991; Rayner and Castelhano, 2007).
One of the core hypotheses in eye-tracking research is the so-called eye–mind assumption. This hypothesis holds that there is a direct and immediate correlation between what humans are looking at, at a given point in time, and what they are cognitively processing (Just and Carpenter, 1980). That is, according to this assumption, whatever our eyes our looking at indicates what our mind is processing in real time (Pieters and Wedel, 2004). Cognitive psychology studies applied to reading-based tasks have shown that eye movements and fixations adjust dynamically in response to the degree of difficulty of the text. This supports the eye-mind assumption, as it suggests that the eyes move in response to the cognitive demands of textual content.
Turning to the ELM model, this explains how people process persuasive messages and suggests that the processing route (peripheral vs. central) adopted by the recipient depends on their motivation and ability to process the information contained therein. Generally speaking, the stimuli that make up a VRB tend to demand a low cognitive effort, and it can thus be assumed that they will be processed via the peripheral route, acting in their own right as cues that influence the receiver’s attitude, attention, and subsequent recall of the brand. Other elements—such as descriptions, comments, or posts from other users—will be processed through the central route because these are more relevant and motivating stimuli. This route will entail thorough consideration of the message by the individual, thus rendering behavioral changes more likely. What is more, if the stimuli initiate a further process of attention paid to other elements within the scene, a process of attention-transfer will arise—as previously demonstrated by Pieters and Wedel (2004). Attention will thus be directed toward multiple other stimuli, such as the brand’s products, models, competitor ads, other users’ or brands’ profiles, and so on.
In the proposed conceptual model (Figure 1), we can observe the two means by which visual attention is paid to an ad, as described by Pieters and Wedel (2004): (1) “bottom-up” factors, such as marketing stimuli, which determine its perceptual salience (such as the shape and size of a logo), and (2) “top-down” factors, such as the individual’s search objectives, their particular traits or internal states (motivation, involvement with the brand or product, purchasing experience, and so on), and his or her attentional process. Anderson et al. (2015) contend that the person’s socio-demographic characteristics (such as age, gender, or educational level) can also influence their attention patterns based on previous experiences and acquired knowledge, thus acting as top-down factors. Top-down elements encourage subjects to voluntarily pay more or less attention to stimuli that make up the VRB, such as logotypes, as well as to their pictographic and/or textual elements.
The voluntary process of controlling one’s attention top-down—through the selective selection or suppression of the elements of a given scene or object, which, therefore exerts an effect on recall—requires greater effort and, generally, is much slower than in the case of involuntary control factors or influences (bottom-up) (Wedel and Pieters, 2008). Message storage involves connecting the information most recently encoded with that which was previously stored. Hence, it is only relevant, motivating, or otherwise new or striking content that will prompt the individual to invest mental resources in encoding and storage—and that will therefore be recognized and recalled subsequently.
2.3 Research hypotheses
2.3.1 The influence of visual attention on self-reported recall
This study investigates whether users’ task-related selective attention during the shopping process is modified when the VRB appears on-screen (top-down mechanism), generating attention toward it and subsequent recall of it. Effectively capturing a viewer’s interest is, of course, a primary goal of advertising. Active information-processing is a crucial first step toward drawing the consumer’s attention toward an advertising message (Fishbein and Ajzen, 1975). Hence, attention plays a pivotal role in recognizing stimuli such as ads.
The relationship between visual attention toward an object, interest in that object, and behavior has been widely studied in the field of cognitive psychology and the behavioral sciences. Visual attention—understood as a person’s cognitive and perceptual focus directed toward a specific object in a visual setting—has been described as a proxy for interest and preference, particularly when measured using eye-tracking (Badenes-Rocha et al., 2022). Previous research suggests that objects that capture our visual attention also tend to generate greater interest, are more likely to be remembered and, consequently, are also more likely to influence our behavior and subsequent purchasing decisions (Posner and Petersen, 1990; Rayner and Castelhano, 2007). In particular, sustained attention paid to an object is associated with a higher likelihood of intentional behavior, such as purchase intention, reflecting the importance of attracting and maintaining consumer attention (Pieters and Wedel, 2004).
Various theoretical and empirical justifications support a correlation (e.g. Wedel and Pieters, 2000; Hernandez et al., 2017), and even, an impact of attention on memory/recall (e.g. Bakalash and Riemer, 2013). Sanbonmatsu and Kardes (1988) posit that, when an individual happens to experience a high level of stimulation, they tend to focus more on peripheral (more superficial, less important) stimuli than central ones, as the former are easier for the brain to process. The degree of relevance of the task in question also influences the level of attention allocated to it by the individual and, consequently, its memorability.
After capturing the user’s attention, the advertising message and brand will influence recall, as their cognitive processing will lead to the creation of storage mechanisms for the information displayed (Lee and Ahn, 2012). The extant research supports a positive relationship between the degree of visual attention the user pays to various kinds of ads and self-reported recall in different research contexts, as evidenced by studies conducted by Simola et al. (2013), Scott et al. (2016), Muñoz-Leiva et al. (2021), or Gómez-Carmona et al. (2021). Therefore, the following research hypothesis is proposed:
Visual attention has a positive effect on self-reported recall of the VRB displayed in social commerce profiles.
2.3.2 The influence of gender
The relationship between the individual’s gender and the visual attention they pay to an object or element, as well as their recall of it, has been the subject of previous studies from cognitive psychology and the behavioral sciences. In particular, research has shown that there are significant differences in how men and women process information visually and recall objects. These gender differences have major implications for the design of marketing and visual communication strategies.
The meta-analysis conducted by Voyer et al. (2007) shows that there are significant gender differences when it comes to object-identity memory and that these are consistently tipped in favor of women. For instance, in the aforementioned meta-analysis, it was found that women recalled specific details about the observed object, including common or geometrical objects. When it came to recall of masculine objects, the balance tipped in favor of males. Other research indicates that women are more sensitive to esthetic and design aspects than men, which affects their visual attention and, subsequently, their product recall (Meyers-Levy and Loken, 2015).
In specific contexts such as online shopping and advertising, Barreto’s research (2013) indicated that women tend to notice more banners than men, yet there were no statistically significant differences between the genders in terms of the banner ads that were read and/or clicked. This aligns with the findings of Goodrich (2014), who found that women process information more holistically—paying attention to multiple elements simultaneously—which enables them to take in more contextual and emotional details. Delen and Ilter (2021) found that, in the case of products related to personal care or fashion, women presented greater attention and visual retention than men when images of female models were used. Other studies, such as that of Sørum (2018), have also shown that women are capable of recalling ads better than men.
Studies dealing with visual perception have shown that, compared to men, women present a stronger response to ads that convey emotions or contain visual elements related to stories or social contexts (Pieters and Wedel, 2004)—that is, ads that evoke a narrative connection (Goodrich, 2014). Men, in contrast, are more receptive to ads containing clear, direct messages, with less emphasis on the emotional context (Goodrich, 2014).
The study conducted by Meyers-Levy and Loken (2015) also finds that women have a greater tendency to process information more comprehensively, which implies greater attention to both the visual and narrative aspects of ads. It may therefore be that women’s detailed and multisensory processing capacity contributes to better retention of logos on social networks. Furthermore, as social networks constitute spaces in which images are combined with narratives and emotions, women are likely to become more involved with those ads that bring images and textual elements together (Orth and Holancova, 2004), which, in turn, may lead to better retention not only of logotypes but also banners.
Building on these findings and H1, the following research hypothesis is proposed:
Gender has an effect on recall of the VRB displayed in s-commerce profiles. Specifically, women are more likely to recall the VRB than men.
2.3.3 The influence of age
Typically, when websites are dominated or overshadowed by ads and text, visitors tend to spend less time on them; however, this trend is less prevalent among older users, who tend to stay for longer on sites that are ad-heavy. Thus, Website advertising may appeal more to older users. Similarly, Drèze and Hussherr (2003) find that, while adults and young people view the same number of zones on a page, the former focus more on those zones and fixate for longer.
One study, conducted by Djamasbi et al. (2010), revealed that young adults have a greater affinity for eye-catching visual elements and complex graphical interfaces on social media, while older users prefer simpler and clearer interfaces. Adult users have also been found to spend more time on websites containing ad content than their younger counterparts, according to Danaher et al. (2006), Drèze and Hussherr (2003), Hernández-Méndez and Muñoz-Leiva (2015), Yu et al. (2021), and Joseph et al. (2021). In contrast, younger users tend to make shorter visits to websites to perform their desired tasks, and their visual fixations on different areas of the screen are much briefer, including when they notice banner ads. Older users are therefore expected to present better logo recall than younger ones. In this context, the following research hypothesis is proposed:
Age has a positive effect on recall of the VRB displayed in s-commerce profiles. Specifically, the older the person, the more likely they are to recall the VRB.
2.3.4 The influence of s-commerce experience
More experienced Internet users process website information faster than novices because they perform fewer fixations, scanning fewer regions and spending less time on each area. Yet, this does not imply that they are exposed any less to advertising stimuli than novice users (Drèze and Hussherr, 2003). Thorbjørnsen et al. (2002) found that novices are less likely to engage with online advertising because they prioritize content or tasks that are in harmony with the website’s environment over marketing content. Consequently, experienced Internet users are expected to interact with advertising banners more frequently than novices. This pattern has been corroborated by other studies, such as Arenas et al. (2021), analyzing the Microsoft Word user interface with eye-tracking, Guo et al. (2021), investigating mobile news apps with eye-tracking, or Joseph et al. (2021), examining smartphone applications. Generally, users with greater technological proficiency find it easier to navigate technology services and products than those lacking such experience.
The present study is thus grounded on the premise that users with previous experience of purchasing on social networks (Herzallah et al., 2022) will have a different recall than those who have no such experience (Kim and Noh, 2012). Therefore, the following research hypothesis is proposed:
Previous experience of shopping via s-commerce has an effect on recall of the VRB displayed in s-commerce profiles. Specifically, users experienced in s-commerce are more likely to recall the VRB than novice users.
3. Methodology
3.1 Data collection and validity of the experiment
An eye-tracking experiment was conducted during the period April 26–May 25, 2022, in a university laboratory. The research participants were selected through quota sampling via phone or email from a predetermined list and were each compensated with €10 for their time. The final sample comprised 100 individuals aged 16 and above (mean = 35.01 years). Participants (all of whom were based in Granada in southern Spain) were then randomly assigned to one of four groups or quotas based on different permutations of male/female and age range (less than 44 and 45 or over), ensuring a representative sample that mirrored the demographics of the Instagram user population, which is characterized by a predominance of young women (We Are Social, 2021). The percentage distribution of sample participants by gender and age range was:
- (1)
Aged 16–34: 18% were male and 23% were female, totaling 41%.
- (2)
Aged 35–44: 13% were male and 18% were female, totaling 31%.
- (3)
Aged 45–55: 8% were male and 7% were female, totaling 15%.
- (4)
Aged 56 and above: 6% were male and 7% were female, totaling 13%.
Overall, 45% of participants were male, and 55% were female.
In the data-collection phase, once the participants had completed a shopping experience in the Instagram Shop or Instagram Stories, they completed an online survey via Google Forms to assess their opinions on s-commerce, as well as their attitudes and intention toward s-commerce and their logo recall.
The laboratory was equipped with a laptop computer with Tobii Pro Lab v.1.130 software and linked to a Tobii Pro Nano eye-tracker. This system has an accuracy of 0.3° and a precision of 0.10° RMS (utilizing a built-in filter) under optimal conditions, with a sampling rate of 60 Hz.
To enhance the validity and generalizability of the experiment, two distinct s-commerce profiles were examined (the brand’s “Instagram Shop” and “Instagram Stories”), and a sufficiently large sample size was chosen, ensuring that industry-relevant conclusions can be drawn.
3.2 Experiment design, metrics, and data analysis technique used
The true-experiment design was based on a mixed approach, blending different between-group comparisons (experimental groups, GEi) with within-subjects analysis of repeated stimuli from different stages of the purchasing process, using gaze metrics (Oj). This design was chosen to conduct a random selection (R) of participants, controlling two independent variables (gender and age) that were of special interest for the dependent variable (recall), to ensure sample representativeness. Another independent variable, experience level, was also measured. No control group was used. In this way, this true-experiment design enabled between-groups comparisons to be made—two genders, four age ranges, and two experience levels—and cause–effect relationships between the variables to be identified.
The scenarios (scenario 1: Mango Shop; scenario 2: Mango Stories) were shown randomly (reading Figure 2 left-to-right and top-to-bottom, the first four images relate to scenario 1, and the final two images relate to scenario 2). That is, sometimes the experiment began with scenario 1, and sometimes with scenario 2, in no particular order. This precaution was taken to mitigate any possible effect of the sequence of presentation on the attention paid and recall of the scenarios. The overall experiment design and between-groups comparisons were thus as follows:
{Scenario 1 or 2}/{Scenario 2 or 1}
The experimental procedure involved simulating the shopping process for the participant, from the fashion retailer Mango, via real-size images of a smartphone (6.5-inch screen). This online task simulated a browsing experience of Mango’s Instagram profiles (see Figure 2), up to the point of reaching the simulated payment gateway.
Eye-tracking records and analyzes users’ fixations to measure the attention they focus on specific areas of interest (AOI; see Figure 2): the wordmarks and combination marks of the Mango brand, located at the top of the screen. These visual elements were repeatedly displayed during the clothing-selection process and at the payment gateway, differing mainly in size and precise position. In total, seven AOIs were defined for this analysis: one wordmark (AOI1) and one combination mark (AOI2), displayed on the Instagram Shop landing page, two further AOIs comprising a wordmark next to a combination mark (AOI3 and AOI4), displayed during the purchasing process, and one wordmark appearing at the top of the “add to basket” window (AOI5); and, on the Instagram Stories landing page, one wordmark next to a combination mark (AOI6), and a wordmark displayed during the purchasing process (AOI7). One further area of the screen was initially identified as an AOI (AOI18), which related to a link to the company’s e-commerce website (shop-mango.com) that appeared in small print at the top of the screen at certain points in the purchasing process. However, as this was not a logotype, it was eliminated from the analysis.
After defining the AOIs, various oculomotor parameters were established for each of them. The most widely-applied fixation metrics in the scholarly marketing literature were selected, namely: fixation count (FC), total fixation duration (TFD), fixation duration (FD), and time to first fixation (TFF), as employed by Hernández-Méndez and Muñoz-Leiva (2015), Muñoz-Leiva et al. (2019), Espigares-Jurado et al. (2020), and Badenes-Rocha et al. (2022), among others. Next, we present a brief description of each metric.
Many researchers contend that the overall significance or noticeability of an object leads to an increased number of fixations (FC) on it (Jacob and Karn, 2003). This study focused on FC within a specific AOI. However, due to the highly generic nature of this assessment, it needed to be supplemented with more specific metrics linked to information-processing (Holmqvist et al., 2011; Jacob and Karn, 2003). Therefore, we also included TFF on the AOI (measured in seconds), FD (measured in milliseconds), and TFD (also measured in milliseconds).
TFD represents the total amount of time that an individual spends fixating on a given AOI. According to Unema et al. (2005), there is a pattern composed of an initial orientation phase followed by a more detailed examination of critical information, possibly indicating a distinction between ambient and focal processing modes.
Factors such as AOI size, task clarity, and foreground/background contrast of visual targets, among other parameters, can impact the TFF (Holmqvist et al., 2011). For instance, it takes longer to notice or fixate on the specific visual elements under analysis (in this case, the Mango wordmark and combination mark) when there is more visual complexity on-screen. This particular example demonstrates the ability of some stimuli to capture users’ attention and suggests a level of automatic processing with minimal awareness (Colorado et al., 2015).
But, to be effective, advertising must not only captivate viewers’ attention but also remain in their short- and long-term memory (Barreto, 2013). In the present study, banner recall was evaluated using an approach adapted from previous studies (Danaher and Mullarkey, 2003; Hernández-Méndez and Muñoz-Leiva, 2015; Muñoz-Leiva et al., 2019). This was based on a single open-ended question for unaided recall, drawing on short-term working memory (“Please list the names of the brands you remember from your recent visit to Instagram”), followed by aided recall (“Which of these brands do you remember from the entire visit?”). In the latter case, participants were asked to choose from among different brands: Mango, plus other brands that were not used in the study. Finally, to arrive at the final score for recall, responses were rated as follows: three points were assigned when the individual recalled the Mango brand in response to both the open and closed questions; two points when they remembered it in response to one of those questions; and one point when they had no recollection of having seen it.
To test the research hypotheses, several multinomial regression model with backwise selection was used in R 4.1.1 computational software. Gender, age, and experience in the use of s-commerce tools were treated as independent variables or classification covariates in the extracted models. The frequency-of-use categories were: “never”, “yes, sometimes, but in the past”, and “yes, I regularly purchase via social networks, at least twice a year”.
4. Results
4.1 Heatmaps by classification variable
In the case of gender (Figure 3a1 and 3b1), analysis of Mango’s Instagram Shop profile shows that men tend to focus on both types of brand marks (combination mark and wordmark), whereas women tend to focus more on the combination mark. In the case of age, analysis of Instagram Shop reveals that individuals aged 16–34 (Figure 3a2) exhibit a considerable focus on both types of brand marks, while those aged 35–44 (Figure 3b2) show a specific interest in the combination mark. However, individuals aged 56 or over (Figure 3d2) display a deep focus on the products themselves. Turning to the factor of s-commerce experience, the analysis shows that, experienced users (Figure 3a3) exhibit a significant focus on both types of brand marks, while novice users (Figure 3b3) tend to focus more on the products and less on the logos.
In the case of Instagram Stories (Figure 3c1 and 3d1), men demonstrate slightly greater interest in the brand logo than women; while both genders focus on the products, largely because, in the Stories format, the images used are fewer in number but larger in size. All age groups (Figure 3e2, 3f2, 3g2, and 3h2) behave similarly in focusing on the brand mark, and they also focus more on the garments themselves. And finally, both experienced and novice users again tend to concentrate on the products rather than the brand.
As expected, increasing the size of an ad element (such as a photograph of the garment) increased both its perceptual salience and its attentional demand, therefore detracting attention from other elements (such as logotypes).
4.2 Testing the research hypotheses
Turning to the multinomial regression for hypothesis testing, three models were extracted, for which the variable to be explained was recall of the Mango brand’s logo in s-commerce. Model 2 involves the visual-attention independent variables TDF, FC, and TFF (hypothesis H1), while Model 3 includes classification covariates: gender (H2), age range (H3), and experience level (H4). Model 1 encompasses all the aforementioned independent variables.
Table 1, and Table A1 and A2 (in the Appendix), present the regression results for models 1, 2, and 3, respectively. To evaluate the model fit, likelihood ratio tests were conducted, and unexplained variance and some Pseudo R-squared scores (R2) were computed (Table A1 in the Appendix). Model 1 (Table 1) is deemed the most suitable as it considers more significant independent variables than the other models and explains a larger portion of the original variability.
H1 proposes that visual attention positively influences self-reported recall of the brand mark/logo displayed. Keeping all variables constant, each time a participant looks at the brand logo (FC variable), they are 1.647 or 1.693 times more likely to partially or fully recall the brand, respectively, than not to recall it at all, according to the first model (Table 1). Similar outcomes are obtained for the second model (odds ratio = 1.628, p < 0.001; odds ratio = 1.687, p < 0.001; see Table A2). However, the effect of the TFF covariate on partial recall of the Mango brand (for a one-unit increase in TFF) is limited (p = 0.46); but it is significant in the case of full recall (p = 0.0366). As the effect of TFD was not significant in the extracted models, this variable was discarded. Therefore, H1 is partially validated.
H2 proposes that gender affects brand logo recall. Keeping the rest of the covariates constant, Table 1 indicates that women recall the logo better than men: they are more likely to partially recall it (odds ratio = 7.838 and p < 0.001) and more likely to fully recall it (odds ratio = 4.811 and p < 0.001) than women. Therefore, H2 is supported. These results are consistent with those obtained with Model 3 (reported in Table A2), albeit the coefficients are not significant.
H3 proposes that users’ age affects brand logo recall. Table 1 shows an important effect of certain age groups (35–44 and 45–55) compared to the reference age group (15–34) on recall, given that the other variables remain constant. Individuals aged 35 to 44 have a higher likelihood of presenting partial recall than no recall (odds ratio = 5.289 and p < 0.001). Similarly, they are more likely to fully recall it than not at all (odds ratio = 2.055 and p < 0.001). Moreover, individuals in the 45–55 age group are more likely to recall the logo compared to the reference age group (15–34). However, there is an inverse effect among those aged 56 or above on brand logo recall, compared to those aged 15–34. Overall, then, H3 is validated.
H4 proposes that, if the user has past experience of shopping via social networks, this will affect their brand logo recall. Table 1 shows that individuals who do have experience of buying online present a higher level of recall than those with no experience, when all other variables remain constant. They are more likely to recall the logo both partially (odds ratio = 0.000, p < 0.001) and fully (odds ratio = 0.000, p < 0.001). Table A2 shows similar regression estimates for experience. Therefore, H4 is supported.
5. Conclusions
5.1 Theoretical implications
Today, s-commerce facilitates connections between sellers and customers through social platforms and websites (Liao et al., 2021). The review of the academic literature showed that s-commerce has seen rapid growth in recent years. But, this shift toward managing the customer experience in s-commerce presents new challenges for businesses (Pour et al., 2021) and has become an important topic in this field of research on online consumer behavior. Particularly, the question of how to build a trustworthy, accessible, and usable customer experience in s-commerce has garnered attention from numerous experts and academics in recent years (e.g. Tseng, 2023; Mikalef et al., 2023).
Previous studies conducted in the online and mobile environment emphasized that the quality and relevance of advertising content, rather than its display format, determine ad effectiveness. Therefore, the literature recommends that ads should offer clear, direct, logical, and useful information (San José et al., 2004), such that they stand a better chance of being processed via the central route (and, thus, capture more attention), compared to the peripheral route (which is more typical in the case of a VRB). Such recommendations take into account the principles of the ELM (Petty and Cacioppo, 1986) and the AC-TEA model (Pieters and Wedel, 2004). However, variations in the manner in which people process messages (through one route or another) are explained through selective exposure theory, which rests on variables such as personal values or preferences (see Badenes-Rocha et al., 2022, applied to the cause-marketing context, from a multi-method approach combining self-reports and eye-tracking) or the individual’s motivation and capacity, as the receiver, to process the information in question (Pieters and Wedel, 2004).
According to the aforementioned AC-TEA model, attention is the first key step toward triggering cognitive and emotional processing. This study corroborates the importance of capturing attention effectively. Our application contributes to the AC-TEA model by incorporating new findings regarding the impact of user classification variables from a specific context: s-commerce via mobile devices. In particular, the present study enables us to better understand how users of these tools allocate their visual attention across the different elements of the VRB, and how this attention translates into recall; and it identifies how this recall varies depending on the top-down factors of gender, age, and level of experience with s-commerce. These factors, inherent to the individual, encourage greater or lesser memorization via a voluntary attentional process vis-à-vis the logotypes.
The present study thus compared the effectiveness of two different visual representations (wordmarks vs. combination marks) of the brand (Mango) across two different social network tools (Instagram Shop vs. Instagram Stories), using eye-tracking technology combined with questionnaires to measure the participants’ attention and recall. This psycho-physiological tool is considered highly suitable for measuring comprehensive cognitive and affective reactions in communication studies (Hernández-Méndez and Muñoz-Leiva, 2015).
From a more quantitative perspective, just as previous studies have identified (e.g. Hughes et al., 2003; Pieters and Wedel, 2004), we found that attention exerted less of an effect on logo recall, in particular, in terms of FD and TFF metrics. However, the TFD and FC values strongly correlate with the recall of the different logotypes.
In terms of gender, our results stand in contrast to the findings of previous studies. For example, the work of Pieters and Wedel (2004) established that men show a higher level of visual retention when exposed to ads featuring prominent and minimalist graphic elements, while women tend to pay more attention to the contextual and verbal details of the content (Pieters and Wedel, 2004). These differences could be due to: (1) the different cognitive strategies of the two genders, whereby women process more efficiently and tend to recall more visual information than men, and (2) the present research context—commerce via social networks. This context ultimately fosters the retention of more visually “direct”, simple, or striking banners or advertising logos (Rosbergen et al., 1997)—such as those used in this study—among certain groups, such as women or more experienced online shoppers.
Age, on the other hand, seems to have an inverted U-shaped effect on recall, with the 35–55 age group presenting the highest probability of partial or full recall of the logo.
5.2 Managerial implications
Before deciding where to place a logotype on an s-commerce platform, the firm should conduct a comprehensive analysis of its social network profile to identify precisely how users tend to visualize it. In particular, this analysis should examine which elements of content draw users’ attention and how these are processed (via the peripheral or the central route). Armed with this insight, the firm will be better placed to create relevant content for its e-commerce profile and to adopt the best strategy when it comes to placing the different elements—including logotypes—on-screen, which will significantly improve the effectiveness of the VRB (He et al., 2021). In general terms, pictographic elements have been found to achieve greater effectiveness, in terms of attracting visual attention, when they are located toward the top of the screen (Espigares-Jurado et al., 2020; Gholami et al., 2021), this being linked to improved brand recall (Gholami et al., 2021).
For marketers, it is crucial to consider the importance of logo position and type when designing advertising campaigns on s-commerce platforms because these elements can prompt attention toward other elements on the social network, through an endogenous transfer process. That is, attention can be directed to other ad elements (other products, profiles, and so on), quite independently of the factors that stimulated attention to the pictorial elements in the first place (Pieters and Wedel, 2004). These latter authors (Pieters and Wedel, 2004) found that it was the branding, typically represented visually by a logotype, that delivered a markedly superior performance—compared to other ad elements—in terms of successfully transferring the consumer’s attention to pictorial elements (such as a branded product catalog) and textual elements (product description). Furthermore, identifying the visualization patterns of different groups according to demographic variables and degree of experience of s-commerce may help to significantly improve the effectiveness of ad campaigns and, ultimately, contribute to better brand recognition and recall (Gholami et al., 2021).
By understanding how different types of VRB affect consumer attention and recall, depending on demographic variables, advertisers will be better placed to optimize their s-commerce strategies.
Regarding the “gender” variable, women exhibited more attention to the logo and better recall than men. These results are consistent with those of previous studies applied to other research contexts, such as Goodrich (2014), in the context of attitudes toward online ads, who found that women process information more holistically, paying attention to multiple elements simultaneously, which enables them to pick up more contextual and emotional details. In short, women’s tendency to process visual stimuli more deeply and emotionally could explain why they present better recall of logos and banner ads on social networks than men (Orth and Holancova, 2004). This would suggest that women may have a better recall of ads that are more “comprehensive” in the sense of including stories or detailed visual elements (such as in the case of s-commerce stories), while men may find ads that prioritize visual simplicity more memorable. Consequently, businesses could simply tailor two distinct ad strategies for men and women. For example, just as when promoting high-risk items (Wollin, 2003), businesses could use a blend of objective and subjective (more subliminal) advertising for women, while centering mainly on objective advertising for men. This insight can also be applied to the case of logo design.
Regarding age, older people (in the 35–55 age range) were found to recall logos better than younger people. This finding is in line with previous studies, which have established that older users spend more time on websites with advertising content, for instance (e.g. Danaher et al., 2006; Drèze and Hussherr, 2003; Hernández-Méndez and Muñoz-Leiva, 2015; Yu et al., 2021; Joseph et al., 2021). We suggest that firms seeking to achieve broader brand recall via logotypes should therefore target greater effort toward advertising that captures younger audiences.
The present study also found that those participants with experience of purchasing online presented better recall (both partial and full) of the brand logo than those without such experience. This was also found in the previous literature in different contexts—such as Arenas et al. (2021) (Microsoft Word user interface), Guo et al. (2021) (new mobile apps), or Joseph et al. (2021) (mobile apps), among other studies. Hence, firms should channel more resources into attracting attention to their brands among less experienced online shoppers.
Therefore, the results point to an interesting avenue for future research on the effectiveness of VRB in terms of paying visual attention and as a result, determining the optimal on-screen location for the logo—may assist in enhancing business processes, considering the characteristics of the customers who use these s-commerce tools.
6. Limitations and future lines of research
The present research focused on investigating the attention or fixation behavior of participants to measure the effectiveness of the VRB, using cross-sectional data. In contrast, a longitudinal study could offer useful interpretations regarding the behavior of the causal relationships identified over time. In particular, it would be valuable to investigate how brand attention and recall are sustained over time—for instance, by measuring recall at least one week after initial exposure—to provide a more comprehensive understanding of brand retention. This would facilitate comparison between immediate and long-term recall, to provide a more robust analysis of the effectiveness of visual representation in s-commerce environments, as well as to better understand the temporal dynamics of brand recall.
Second, for several reasons, participants’ exploration of Mango’s product profile on Instagram was conducted on a desktop PC—that is, on a computer screen rather than a mobile screen. The experiment could be recreated using a more natural setting, on mobile devices such as tablets and/or smartphones.
Third, with regard to the oculomotor parameters adopted in the experiment, future studies could devote more attention to applying different measures drawn from eye-tracking studies relating to attention (such as pupil dilation, saccade angle, glances, or reaction time), in combination with the kind of self-report measures employed in the present study.
Finally, it would be interesting to combine the eye-tracking methodology and survey study with other neurophysiological measures (such as electroencephalography or galvanic skin response). Such an approach could contribute to a better understanding of individuals’ attentional and affective reactions to the use of logotypes by fashion retailers on s-commerce platforms.
Figures
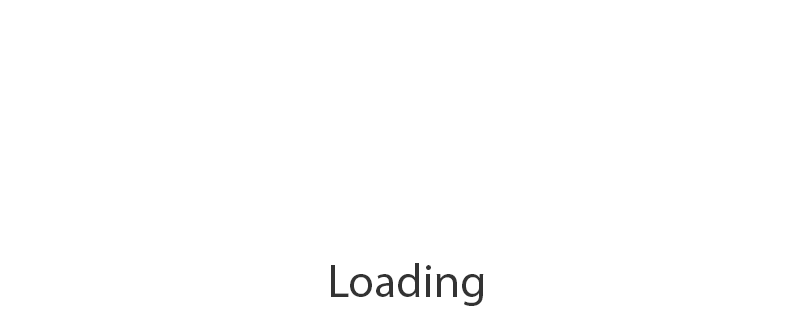
Figure 3
Examples of heatmaps depicting fixation zones on Mango’s Instagram Shop brand profile, and Instagram Stories, categorized by gender: male (a1 and c1) and female (b1 and d1); by age: 16–34 (a2 and e2), 35–44 (b2 and f2), 45–55 (c2 and g2), and 56 and above (d2 and h2); and by experience level: expert (a3 and c3) and novice (b3 and d3)
Logit coefficients, odds ratios and results of tests of hypothesis for Model 1
Category | Partial brand recall (2) | Full brand recall (3) | ||||
---|---|---|---|---|---|---|
Variables | Logit coeff | Odds ratios | p | Logit coeff | Odds ratios | p |
Intercept | 8.435 | 4607.4 | <0.001 | 9.425 | 12397.6 | <0.001 |
TFD | −0.001 | 0.999 | <0.001 | −0.001 | 0.999 | <0.001 |
FC | 0.499 | 1.647 | <0.001 | 0.527 | 1.693 | <0.001 |
TFF | −0.000 | 1.000 | 0.4600 | −0.000 | 1.000 | 0.0366 |
Gender (Femalea) | 2.059 | 7.838 | <0.001 | 1.571 | 4.811 | <0.001 |
Age group (35–44b) | 1.666 | 5.289 | <0.001 | 0.720 | 2.055 | <0.001 |
Age group (45–55b) | 19.705 | 361 × 106 | <0.001 | 19.365 | 257 × 106 | <0.001 |
Age group (over 55b) | −16.451 | 0.000 | <0.001 | 0.617 | 1.853 | <0.001 |
Experience (Novicec) | −9.977 | 0.000 | <0.001 | −8.016 | 0.000 | <0.001 |
Note(s): aCategory under reference: male
bCategory under reference: 15–34
cCategory under reference: experienced
Source(s): Table by authors, using R software
Model effectiveness comparison
Model 1 | Model 2 | Model 3 | |
---|---|---|---|
R2 (McFadden) | 0.1929 | 0.0694 | 0.0751 |
R2 (Cox and Snell) | 0.1889 | 0.0726 | 0.0826 |
R2 (Nagelkerke) | 0.2852 | 0.1096 | 0.1210 |
Residual Dev | 86.7323 | 99.9996 | 107.2374 |
AIC | 122.7323 | 115.9995 | 131.2374 |
Source(s): Table by authors, using R software
Logit coefficients, odds ratios and results of tests of hypothesis for Models 2 and 3
Model | Category | Partial brand recall (2) | Full brand recall (3) | ||||
---|---|---|---|---|---|---|---|
Variables | Logit coeff | Odds ratios | p | Logit coeff | Odds ratios | p | |
Model 2 | Intercept | 1.623 | 5.071 | <0.001 | 2.998 | 20.038 | <0.001 |
TFD | −0.001 | 0.999 | <0.001 | −0.001 | 0.999 | <0.001 | |
FC | 0.487 | 1.628 | <0.001 | 0.523 | 1.687 | <0.001 | |
TFF | −0.000 | 1.000 | 0.027 | −0.000 | 1.000 | 0.009 | |
Intercept | 6.979 | 1074.2 | 0.430 | 8.104 | 3306.6 | 0.419 | |
Gender (Femalea) | 1.614 | 5.021 | 0.102 | 1.773 | 5.891 | 0.061 | |
Age group (35–44b) | 1.362 | 3.904 | 0.155 | 0.739 | 2.094 | 0.269 | |
Age group (45–55b) | 9.566 | 14275.3 | 0.458 | 9.369 | 11720.7 | 0.459 | |
Model 3 | Age group (over 55b) | −0.705 | 0.494 | 0.338 | −0.162 | 0.851 | 0.448 |
Experience (Novicec) | −7.357 | 0.001 | 0.426 | −6.403 | 0.002 | 0.436 |
Note(s): aCategory under reference: male bCategory under reference: 15–34 cCategory under reference: experienced
Source(s): Table by authors, using R software
References
Anderson, N.C., Anderson, F., Kingstone, A. and Bischof, W.F. (2015), “A comparison of scanpath comparison methods”, Behavior Research Methods, Vol. 47 No. 4, pp. 1377-1392, doi: 10.3758/s13428-014-0550-3.
Arenas, A.G., Moorkens, J. and O'Brien, S. (2021), “The impact of translation modality on user experience: an eye-tracking study of the Microsoft Word user interface”, Machine Translation, Vol. 35 No. 2, pp. 205-237, doi: 10.1007/s10590-021-09267-z.
Badenes-Rocha, A., Bigne, E. and Ruiz, C. (2022), “Impact of cause-related marketing on consumer advocacy and cause participation: a causal model based on self-reports and eye-tracking measures”, Psychology and Marketing, Vol. 39 No. 1, pp. 214-226.
Bakalash, T. and Riemer, H. (2013), “Exploring ad-elicited emotional arousal and memory for the ad using fMRI”, Journal of Advertising, Vol. 42 No. 4, pp. 275-291, doi: 10.1080/00913367.2013.768065.
Barnhart, B. (2021), “Social commerce: 16 awesome examples of social selling”, Photoslurp, available at: https://hi.photoslurp.com/blog/social-commerce-examples/
Barreto, A. (2013), “Do users look at banner ads on Facebook?”, Journal of Research in Interactive Marketing, Vol. 7 No. 2, pp. 119-139, doi: 10.1108/jrim-mar-2012-0013.
Casado-Aranda, L.A., Sánchez-Fernández, J., Bigné, E. and Smidts, A. (2023), “The application of neuromarketing tools in communication research: a comprehensive review of trends”, Psychology and Marketing, Vol. 39 No. 1, pp. 1737-1756, doi: 10.1002/mar.21832.
Colorado, D.A., Taba, M.C. and Parra, H.C. (2015), “Las etiquetas nutricionales: una mirada desde el consumidor”, Revista En-contexto/ISSN: 2346-3279, Vol. 3, pp. 121-140.
Danaher, P.J. and Mullarkey, G.W. (2003), “Factors affecting online advertising recall: a study of students”, Journal of Advertising Research, Vol. 43 No. 3, pp. 252-267, doi: 10.1017/s0021849903030319.
Danaher, P.J., Mullarkey, G.W. and Essegaier, S. (2006), “Factors affecting web site visit duration: a cross-domain analysis”, Journal of Marketing Research, Vol. 43 No. 2, pp. 182-194, doi: 10.1509/jmkr.43.2.182.
Delen, E. and Ilter, B. (2021), “The effect of human images in advertisements on consumer attention by product type and gender: an eye-tracking study”, Pazarlama ve Pazarlama Araştırmaları Dergisi, Vol. 14 No. 2, pp. 329-358.
Djamasbi, S., Siegel, M. and Tullis, T. (2010), “Generation Y, web design, and eye tracking”, International Journal of Human-Computer Studies, Vol. 68 No. 5, pp. 307-323, doi: 10.1016/j.ijhcs.2009.12.006.
Dolah, J., Fong, C.Z., Majid, A.Z.A. and Gee, L.L.S. (2021), “Contemporary shape of logo design for design quality and effectiveness”, Jurnal Gendang Alam (GA), Vol. 11 No. 1, p. 3.
Drèze, X. and Hussherr, F.X. (2003), “Internet advertising: is anybody watching?”, Journal of Interactive Marketing, Vol. 17 No. 4, pp. 8-23, doi: 10.1002/dir.10063.
Espigares-Jurado, F., Muñoz-Leiva, F., Correia, M.B., Sousa, C.M., Ramos, C.M. and Faísca, L. (2020), “Visual attention to the main image of a hotel website based on its position, type of navigation and belonging to Millennial generation: an eye tracking study”, Journal of Retailing and Consumer Services, Vol. 52, 101906, doi: 10.1016/j.jretconser.2019.101906.
Fishbein, M. and Ajzen, I. (1975), Belief. Attitude, Intention and Behavior: An Introduction to Theory and Research, Vol. 6, Addison-Wesley, Reading, MA.
Gholami, Y., Taghvaei, S.H., Norouzian-Maleki, S. and Sepehr, R.M. (2021), “Identifying the stimulus of visual perception based on eye-tracking in urban parks: case study of Mellat Park in Tehran”, Journal of Forest Research, Vol. 26 No. 2, pp. 91-100, doi: 10.1080/13416979.2021.1876286.
Gómez-Carmona, D., Muñoz-Leiva, F., Liébana-Cabanillas, F., Nieto-Ruiz, A., Martínez-Fiestas, M. and Campoy, C. (2021), “The effect of consumer concern for the environment, self-regulatory focus and message framing on green advertising effectiveness: an eye tracking study”, Environmental Communication, Vol. 15 No. 6, pp. 813-841, doi: 10.1080/17524032.2021.1914701.
Goodrich, K. (2014), “The gender gap: brain-processing differences between the sexes shape attitudes about online advertising”, Journal of Advertising Research, Vol. 54 No. 1, pp. 32-43, doi: 10.2501/jar-54-1-032-043.
Guo, F., Chen, J., Li, M., Lyu, W. and Zhang, J. (2021), “Effects of visual complexity on user search behavior and satisfaction: an eye-tracking study of mobile news apps”, Universal Access in the Information Society, Vol. 21 No. 4, pp. 795-808, doi: 10.1007/s10209-021-00815-1.
He, Z., Deng, N., Li, X. and Gu, H. (2021), “How to ‘read’ a destination from images? Machine learning and network methods for DMOs’ image projection and photo evaluation”, Journal of Travel Research, Vol. 61 No. 3, pp. 597-619.
Hernandez, M.D., Wang, Y., Sheng, H., Kalliny, M. and Minor, M. (2017), “Escaping the corner of death? An eye-tracking study of reading direction influence on attention and memory”, Journal of Consumer Marketing, Vol. 34 No. 1, pp. 1-10, doi: 10.1108/jcm-02-2016-1710.
Hernández-Méndez, J. and Muñoz-Leiva, F. (2015), “What type of online advertising is most effective for eTourism 2.0? An eye tracking study based on the characteristics of tourists”, Computers in Human Behavior, Vol. 50, pp. 618-625, doi: 10.1016/j.chb.2015.03.017.
Herzallah, D., Muñoz-Leiva, F. and Liebana-Cabanillas, F. (2022), “Drivers of purchase intention in Instagram commerce”, Spanish Journal of Marketing-ESIC, Vol. 26 No. 2, pp. 168-188, doi: 10.1108/sjme-03-2022-0043.
Herzallah, D., Liébana-Cabanillas, F. and Muñoz-Leiva, F. (2024), “Fashioning consumer choices: recommendation, motivation, and purchase intention toward Instagram commerce. A mediation analysis”, International Journal of Fashion Design, Technology and Education, Vol. 17 No. 3, pp. 1-13, doi: 10.1080/17543266.2024.2308557.
Holmqvist, K., Nyström, M., Andersson, R., Dewhurst, R., Jarodzka, H. and Van de Weijer, J. (2011), Eye Tracking: A Comprehensive Guide to Methods and Measures, OUP, Oxford.
Hughes, A., Wilkens, T., Wildemuth, B.M. and Marchionini, G. (2003), “Text or pictures? An eyetracking study of how people view digital video surrogates”, International Conference on Image and Video Retrieval, Berlin, Heidelberg, Springer, pp. 271-280.
Jacob, R.J. and Karn, K.S. (2003), “Eye tracking in human-computer interaction and usability research: ready to deliver the promises”, in The Mind's Eye, North-Holland, pp. 573-605, doi: 10.1016/B978-044451020-4/50031-1.
Joseph, A.W., Shree, D.J., Saluja, K.P.S., Mukhopadhyay, A., Murugesh, R. and Biswas, P. (2021), “Eye tracking to understand impact of aging on mobile phone applications”, International Conference on Research into Design, Singapore, Springer, pp. 315-326.
Just, M.A. and Carpenter, P.A. (1980), “A theory of reading: from eye fixations to comprehension”, Psychological Review, Vol. 87 No. 4, pp. 329-354, doi: 10.1037//0033-295x.87.4.329.
Kim, S. and Noh, M.J. (2012), “Determinants influencing consumers trust and trust performance of social commerce and moderating effect of experience”, Information Technology Journal, Vol. 11 No. 10, pp. 1369-1380, doi: 10.3923/itj.2012.1369.1380.
Klink, R.R. (2003), “Creating meaningful brands: the relationship between brand name and brand mark”, Marketing Letters, Vol. 14 No. 3, pp. 143-157, doi: 10.1023/a:1027476132607.
LaBerge, D. (1995), Attentional Processing: The Brain's Art of Mindfulness, Harvard University Press, Cambridge, MA.
Lee, J. and Ahn, J.H. (2012), “Attention to banner ads and their effectiveness: an eye-tracking approach”, International Journal of Electronic Commerce, Vol. 17 No. 1, pp. 119-137, doi: 10.2753/jec1086-4415170105.
Liao, S.-H., Widowati, R. and Hsieh, Y.-C. (2021), “Investigating online social media users’ behaviors for social commerce recommendations”, Technology in Society, Vol. 66, August, 101655, pp. 1-14 (In press).
Lin, X., Wang, X. and Hajli, N. (2019), “Building e-commerce satisfaction and boosting sales: the role of social commerce trust and its antecedents”, Journal of Retailing and Consumer Services, Vol. 23 No. 3, pp. 328-363.
McCallum, W.C. (2024), “Definition of attention”, Encyclopaedia Britannica, available at: https://www.britannica.com/science/attention
McLachlan, S. (2020), “What is social commerce and why should your BRAND CARE?”, Social Media Marketing and Management Dashboard, available at: https://blog.hootsuite.com/social-commerce/
Meyers-Levy, J. and Loken, B. (2015), “Revisiting gender differences: what we know and what lies ahead”, Journal of Consumer Psychology, Vol. 25 No. 1, pp. 129-149, doi: 10.1016/j.jcps.2014.06.003.
Mikalef, P., Sharma, K., Chatterjee, S., Chaudhuri, R., Parida, V. and Gupta, S. (2023), “All eyes on me: predicting consumer intentions on social commerce platforms using eye-tracking data and ensemble learning”, Decision Support Systems, Vol. 175, 114039, doi: 10.1016/j.dss.2023.114039.
Molina-Prados, A., Muñoz-Leiva, F. and Prados-Peña, M.B. (2021), “The role of customer brand engagement in the use of Instagram as a ‘shop window’ for fashion-industry social commerce”, Journal of Fashion Marketing and Management, Vol. 26 No. 3, pp. 495-515, doi: 10.1108/jfmm-12-2020-0275.
Morrow, D.J. (1992), “An image makeover”, International Business, Vol. 5 No. 3, pp. 66-68.
Muñoz-Leiva, F., Hernández-Méndez, J. and Gómez-Carmona, D. (2019), “Measuring advertising effectiveness in Travel 2.0 websites through eye-tracking technology”, Physiology and Behavior, Vol. 200, pp. 83-95, doi: 10.1016/j.physbeh.2018.03.002.
Muñoz-Leiva, F., Faísca, L.M., Ramos, C.M., Correia, M.B., Sousa, C.M. and Bouhachi, M. (2021), “The influence of banner position and user experience on recall. The mediating role of visual attention”, Spanish Journal of Marketing-ESIC, Vol. 25 No. 1, pp. 82-110, doi: 10.1108/sjme-04-2020-0050.
Orth, U.R. and Holancova, D. (2004), “Men's and women's responses to sex role portrayals in advertisements”, International Journal of Research in Marketing, Vol. 21 No. 1, pp. 77-89, doi: 10.1016/j.ijresmar.2003.05.003.
Petty, R.E. and Cacioppo, J.T. (1986), “The elaboration likelihood model of persuasion”, Advances in Experimental Social Psychology, Vol. 19, pp. 123-205, doi: 10.1016/s0065-2601(08)60214-2.
Pieters, R. and Wedel, M. (2004), “Attention capture and transfer in advertising: brand, pictorial, and text-size effects”, Journal of Marketing, Vol. 68 No. 2, pp. 36-50, doi: 10.1509/jmkg.68.2.36.27794.
Posner, M.I. and Petersen, S.E. (1990), “The attention system of the human brain”, Annual Review of Neuroscience, Vol. 13 No. 1, pp. 25-42, doi: 10.1146/annurev.neuro.13.1.25.
Pour, M.J., Hosseinzadeh, M. and Mansouri, N.S. (2021), “Challenges of customer experience management in social commerce: an application of social network analysis”, Internet Research, Vol. 32 No. 1, pp. 241-272, doi: 10.1108/intr-01-2021-0076.
Rayner, K. and Castelhano, M.S. (2007), “Eye movement during reading, scene perception, visual search and while looking at print advertisements”, in Wedel, M.y and Pieters, R. (Eds), Visual Marketing: from Attention to Action, Lawrence Erlbaum, New Yersey, pp. 9-42.
Rosbergen, E., Pieters, R. and Wedel, M. (1997), “Visual attention to advertising: a segment-level analysis”, Journal of Consumer Research, Vol. 24 No. 3, pp. 305-314.
San José, R., Gutiérrez, A.M. and Gutiérrez, J. (2004), “Determinantes de la eficacia publicitaria del sitio web. Una aplicación del ELM”, Revista Española de Investigación de Marketing. ESIC, Vol. 8 No. 2, pp. 93-121.
Sanbonmatsu, D.M. and Kardes, F.R. (1988), “The effects of physiological arousal on information processing and persuasion”, Journal of Consumer Research, Vol. 15 No. 3, pp. 379-385, doi: 10.1086/209175.
Scott, N., Green, C. and Fairley, S. (2016), “Investigation of the use of eye tracking to examine tourism advertising effectiveness”, Current Issues in Tourism, Vol. 19 No. 7, pp. 634-642, doi: 10.1080/13683500.2014.1003797.
Shamim, K., Azam, M. and Islam, T. (2024), “How do social media influencers induce the urge to buy impulsively? Social commerce context”, Journal of Retailing and Consumer Services, Vol. 77, 103621, doi: 10.1016/j.jretconser.2023.103621.
Simola, J., Kivikangas, M., Kuisma, J. and Krause, C.M. (2013), “Attention and memory for newspaper advertisements: effects of ad–editorial congruency and location”, Applied Cognitive Psychology, Vol. 27 No. 4, pp. 429-442, doi: 10.1002/acp.2918.
Snyder, R., Irving, L.M. and Anderson, S.D. (1991), “Hope and health: measuring thewWill and the ways”, in Snyder, C.R. and Forsyth, D.R. (Eds), Handbook of Social and Clinical Psychology: The Health Perspective, Pergamon Press, Elmsford, NY, pp. 285-305.
Sørum (2018), “Visual attention and recall in website advertisements: an eye tracking study”, in Ahram, T. and Falcão, C. (Eds), Advances in Usability and User Experience. AHFE 2017. Advances in Intelligent Systems and Computing, Springer, Cham, Vol. 607, pp. 205-216.
Thorbjørnsen, H., Supphellen, M., Nysveen, H. and Pedersen, P.E. (2002), “Building brand relationships online: a comparison of two interactive applications”, Journal of Interactive Marketing, Vol. 16 No. 3, pp. 17-34.
Tseng, H.T. (2023), “Shaping path of trust: the role of information credibility, social support, information sharing and perceived privacy risk in social commerce”, Information Technology and People, Vol. 36 No. 2, pp. 683-700, doi: 10.1108/itp-07-2021-0564.
Unema, P.J., Pannasch, S., Joos, M. and Velichkovsky, B.M. (2005), “Time course of information processing during scene perception: the relationship between saccade amplitude and fixation duration”, Visual Cognition, Vol. 12 No. 3, pp. 473-494, doi: 10.1080/13506280444000409.
Voyer, D., Postma, A., Brake, B. and Imperato-McGinley, J. (2007), “Gender differences in object location memory: a meta-analysis”, Psychonomic Bulletin and Review, Vol. 14 No. 1, pp. 23-38, doi: 10.3758/bf03194024.
We Are Social Spain (2021), “Digital 2021 España”, available at: https://wearesocial.com/es/blog/2021/01/digital-2021-espana/ (accessed 31 October 2021).
Wedel, M. and Pieters, R. (2000), “Eye fixations on advertisements and memory for brands: a model and findings”, Marketing Science, Vol. 19 No. 4, pp. 297-312, doi: 10.1287/mksc.19.4.297.11794.
Wedel, M. and Pieters, R. (2008), “A review of eye-tracking research in marketing”, Review of Marketing Research, Vol. 4, pp. 123-147, doi: 10.4324/9781351550932-5.
Wollin, L.D. (2003), “Gender issues in advertising. An oversight synthesis of research: 1970-2002”, Journal of Advertising Research, Vol. 43 No. 1, pp. 111-129.
Yu, J., Peng, X.R. and Yan, M. (2021), “Effects of age on memory for pragmatic implications in advertising: an eye movement study”, Journal of Pacific Rim Psychology, Vol. 15, 18344909211000452, doi: 10.1177/18344909211000452.
Further reading
Hwang, Y.M. and Lee, K.C. (2017), “Using an eye tracking approach to explore gender differences in visual attention and shopping attitudes in an online shopping environment”, International Journal of Human-Computer Interaction, Vol. 34, pp. 1-10, doi: 10.1080/10447318.2017.1314611.
Muñoz-Leiva, F. (2022), “Eye-tracking technology for measuring banner advertising efficacy on e-tourism websites: a methodological proposal”, in Xiang, Z., Fuchs, M., Gretzel, U. and Höpken, W. (Eds), Handbook of e-Tourism, Springer, Cham, doi: 10.1007/978-3-030-48652-5_121.
Putrevu, S. (2004), “Communicating with the sexes: male and female responses to print advertisements”, Journal of Advertising, Vol. 33 No. 3, pp. 51-62, doi: 10.1080/00913367.2004.10639168.
Acknowledgements
This research was partially funded by the Spanish National Research Program (R+D+i Research Project ECO2017-88458-R, “NeuroTourism project”) and the Andalusian R+D+i Research Program (Grant No: B-SEJ-209-UGR18, “Research in NeuroSOCOM” project). We would also like to thank Beatriz García-Carrión for her valuable insights and advice on specific aspects of consumer neuroscience.
Corresponding author
About the authors
Francisco Muñoz-Leiva is full professor in Marketing and Market Research and holds a PhD in Business Sciences from the University of Granada (Spain). His main research interests are the Internet consumer behavior, retail marketing, and, recently, neuromarketing. His recent research has been published in more than 80 papers in journals indexed in WoS, such as Tourism Management, Physiology and Behavior, Journal of Business Research, International Journal of Advertising, Soft Computing, Expert Systems with Applications, Online Information Review, Information and Management, or Computers in Human Behavior. He was included in Stanford University’s “Ranking of the World Scientists: World’s Top 2% Scientists” in 2021, 2022 and 2023.
Doaa Herzallah is a researcher in Digital Marketing and Consumer Behavior. She holds a doctoral degree in Digital Marketing and International Business from the University of Granada. Her research interests lie in the area of digital marketing where she carries out studies on consumer behavior in social media and social commerce. She has published in high-impact journals including International Journal of Human-Computer Interaction, Journal of Research in Interactive Marketing, and Spanish Journal of Marketing-ESIC, among others. She participated in international conferences such as AEMARK, Marketing AEMARK, AJICEDE, International Marketing Trends, Jornadas Hispano-Lusas, ACEDE, among others.
Ismael Ramón Sánchez-Borrego is a researcher specialized in the field of nonparametric regression and survey sampling. He started his research career in 2001 at the University of Granada. He has extensive experience as a researcher, particularly in his postdoctoral period, and has done productive research stays at Colorado State University and at the University of Michigan, having recently supervised a PhD thesis on Web Surveys. He has published 20 papers in the Journal Citation Reports, area of Mathematics and Statistics and Probability and has led a well-funded research project on web surveys, smartphones and non-probability surveys.
Francisco Liébana-Cabanillas is full professor of Marketing and Market Research at the University of Granada (Spain). His main area of research and interest in the field is the effectiveness of mobile and online banking, Internet consumer Behavior, social media and tourism. He has published articles on these topics in various academic journals, such as International Journal of Information Management, Computers in Human Behavior, Expert Systems with Applications, Tourism Management, Technological Forecasting and Social Change, Financial Innovation and Industrial Management and Data Systems, among others. He was also included in Stanford University’s “Ranking of the World Scientists: World’s Top 2% Scientists” from 2020.