Abstract
Purpose
In their paper titled “A Miracle of Measurement or Accidental Constructivism? How PLS Subverts the Realist Search for Truth,” Cadogan and Lee (2022) cast serious doubt on PLS’s suitability for scientific studies. The purpose of this commentary is to discuss the claims of Cadogan and Lee, correct some inaccuracies, and derive recommendations for researchers using structural equation models.
Design/methodology/approach
This paper uses scenario analysis to show which estimators are appropriate for reflective measurement models and composite models, and formulates the statistical model that underlies PLS Mode A. It also contrasts two different perspectives: PLS as an estimator for structural equation models vs. PLS-SEM as an overarching framework with a sui generis logic.
Findings
There are different variants of PLS, which include PLS, consistent PLS, PLSe1, PLSe2, proposed ordinal PLS and robust PLS, each of which serves a particular purpose. All of these are appropriate for scientific inquiry if applied properly. It is not PLS that subverts the realist search for truth, but some proponents of a framework called “PLS-SEM.” These proponents redefine the term “reflective measurement,” argue against the assessment of model fit and suggest that researchers could obtain “confirmation” for their model.
Research limitations/implications
Researchers should be more conscious, open and respectful regarding different research paradigms.
Practical implications
Researchers should select a statistical model that adequately represents their theory, not necessarily a common factor model, and formulate their model explicitly. Particularly for instrumentalists, pragmatists and constructivists, the composite model appears promising. Researchers should be concerned about their estimator’s properties, not about whether it is called “PLS.” Further, researchers should critically evaluate their model, not seek confirmation or blindly believe in its value.
Originality/value
This paper critically appraises Cadogan and Lee (2022) and reminds researchers who wish to use structural equation modeling, particularly PLS, for their statistical analysis, of some important scientific principles.
Keywords
Citation
Henseler, J. and Schuberth, F. (2023), "Partial least squares as a tool for scientific inquiry: comments on Cadogan and Lee", European Journal of Marketing, Vol. 57 No. 6, pp. 1737-1757. https://doi.org/10.1108/EJM-06-2021-0416
Publisher
:Emerald Publishing Limited
Copyright © 2022, Jörg Henseler and Florian Schuberth.
License
Published by Emerald Publishing Limited. This article is published under the Creative Commons Attribution (CC BY 4.0) licence. Anyone may reproduce, distribute, translate and create derivative works of this article (for both commercial and non-commercial purposes), subject to full attribution to the original publication and authors. The full terms of this licence may be seen at http://creativecommons.org/licences/by/4.0/legalcode
1. Introduction: Cadogan and Lee’s fresh look at partial least squares
Structural equation modeling (SEM) comprises a variety of techniques such as analysis of variance, confirmatory factor analysis, confirmatory composite analysis, regression analysis, path analysis and canonical correlation analysis (Bagozzi et al., 1981; Kline, 2015; Schuberth et al., 2018a; Zhang et al., 2021). It allows the analyst to model theories about conceptual variables, their mutual interrelationships and their relationships to observed variables. Consequently, it has become a widely appreciated method among researchers in various social and behavioral science disciplines such as Psychology, Sociology and Business Research – to name only a few. Arguably, the maximum-likelihood (ML) estimator including its robust versions is the most widely used estimator for SEM (Jöreskog, 1970). Aditionally, a number of alternative estimators were introduced to cope with violations of the ML estimator’s assumptions in empirical work, such as the asymptotic distribution free (Browne, 1984) or the two-stage least squares (2SLS) estimator (Bollen, 2001).
During the past few decades, the partial least squares (PLS) algorithm, as an SEM estimator, has become increasingly popular (Wold, 1975, 1982; Lohmöller, 1989), particularly in Marketing and Information Systems Research (Hair et al., 2012c; Ringle et al., 2012). A recent scientific debate highlighting PLS’s shortcomings (Rönkkö and Evermann, 2013; Henseler et al., 2014) stimulated several enhancements to PLS including consistent partial least squares (PLSc, Dijkstra and Henseler, 2015b), a version of PLS that produces consistent estimates for structural models containing latent variables, and a bootstrap-based test to assess the overall fit of models estimated by PLSc (Dijkstra and Henseler, 2015a).
In their article titled “A Miracle of Measurement or Accidental Constructivism? How PLS Subverts the Realist Search for Truth,” Cadogan and Lee take a fresh look at PLS by evaluating its suitability through the lens of different research paradigms. A research paradigm can be understood as a scientific worldview and “a disciplinary matrix – commitments, beliefs, values, methods, outlooks, and so forth shared across a discipline” (Schwandt, 2007, p. 217). In this spirit, Cadogan and Lee (2022) discuss whether PLS should be an element of the according disciplinary matrix if researchers subscribe to scientific realism or constructivism and to a lesser extent to instrumentalism or pragmatism. They conclude that:
PLS has no utility as a realist scientific tool, but may be of interest to constructivists, since it is uniquely designed to construct different stories of the world depending on the research context.
Yet, is it true that PLS subverts the realist search for truth, as Cadogan and Lee’s title suggests? Should PLS really be removed from the scientific realists’ disciplinary matrix in marketing research?
Cadogan and Lee have a point in that a substantial part of the literature on PLS promotes a mismatch between model and estimator, which leads to inconsistent estimates, i.e. the estimates do not converge in probability toward the true population parameters. However, Cadogan and Lee’s critique is overly broad, because it extends to PLS variants that are potentially valuable to researchers pursuing a realist worldview and to PLS use cases that pose no threat to a realist inquiry. Therefore, this commentary takes a more nuanced look at PLS, thereby allowing the analyst to make more fine-grained selection decisions regarding the estimator of SEM. It will demonstrate that for all covered research paradigms there are consistent PLS estimators that facilitate scientific inquiry.
2. Using “PLS” as a catch-all is an oversimplification
In their article, Cadogan and Lee (2022, Footnote 7) use “PLS” as an umbrella term for various PLS estimators, such as PLSc, ordinal partial least squares (OrdPLS) or robust PLS. However, to lump all PLS estimators together is not well-founded, because they have different objectives and show different statistical properties for different models. Table 1 provides a concise overview of various PLS estimators that have been proposed to estimate structural equation models.
PLS was developed by Wold (1966) as an approach suitable for principal component analysis and (generalized) canonical correlation analysis – at the time still known as nonlinear iterative least squares and nonlinear iterative partial least squares, respectively (Tenenhaus et al., 2005). A few years later, the same author proposed PLS as an estimator for structural models containing latent variables (Wold, 1974, 1982). In this case, PLS determines weights to form composites and subsequently uses these composites to estimate the relationships between the latent variables. As various researchers (including Herman Wold himself) noted, PLS estimates are not consistent for this type of model; they are only consistent at large, i.e. only if both the number of observations and the number of indicators converge to infinity, will PLS estimates converge in probability to the respective population parameters (Hui and Wold, 1982; Dijkstra, 1985; Rönkkö and Evermann, 2013). It was shown only recently that PLS produces consistent estimates for models containing interrelated composites (Dijkstra, 2013, 2017; Cho and Choi, 2020).
Various modifications have been developed for PLS to encounter violations of PLS’ assumptions in empirical research and problems in a collected data set. For instance, Cantaluppi and Boari (2016) invented OrdPLS to deal with ordinal categorical observed variables in a psychometric way. Similarly, Schamberger et al. (2020) developed robust partial least squares (robust PLS) to deal with datasets contaminated by unsystematic outliers.
To overcome PLS’s inconsistency for structural equation models containing latent variables, Dijkstra and Henseler (2015a, 2015b) developed PLSc, which is based on PLS, but applies a correction for attenuation to produce consistent estimates for structural models containing latent variables. It can cope with non-recursive structural models, e.g. models that contain feedback loops, and correlated random measurement errors within a block of indicators (Dijkstra and Henseler, 2015a; Rademaker et al., 2019). Schuberth et al. (2018b) proposed ordinal consistent partial least squares (ordinal consistent partial least squares) to estimate structural models containing latent variables measured by ordinal categorical variables, and Schamberger et al. (2020) introduced robust PLSc as a way to deal with data sets containing unsystematic outliers. Moreover, a version of PLSc has been proposed that uses a ridge least squares estimator to yield the structural parameters in case of multicollinearity (Jung and Park, 2018). Recognizing that PLSc estimates are consistent but not asymptotically efficient for structural models containing latent variables, Huang (2013) proposed PLSe1 and PLSe2 to overcome this issue.
The following subsections focus only on PLS and PLSc to demonstrate the problem of summarizing all estimators for SEM that are based on the partial least squares principle under the umbrella term “PLS.” As the previous paragraphs have shown, their modifications were mainly developed to relax further assumptions made by PLS and PLSc.
2.1 Suitability of “PLS” for the realist variable framework
In their article, Cadogan and Lee present the realist variable framework, which is a theory about causal relationships between unobservable conceptual variables and their causal relationships with observed variables. Transferring this theory into a statistical model results in a structural model containing latent variables, i.e. each unobservable conceptual variable is modeled as a latent variable that is related to a set of observed variables, and the latent variables are embedded in a structural model. Subsequently, Cadogan and Lee (2022, Footnote 5) argue that PLS including PLSc are incompatible with the realist variable framework and structural models containing latent variables.
To investigate the suitability of PLS and PLSc for the realist variable framework and thus for scientific realists, one could imagine a fictitious researcher studying the relationship between three conceptual variables ξ1, ξ2 and η. As the researcher is a scientific realist, he/she applies the realist variable framework (Cadogan and Lee, 2022; Figure 1) and follows the steps as the framework directs: the researcher assumes that the meaning of the three studied conceptual variables is properly defined; according to this researcher’s beliefs, the two unobservable conceptual variables ξ1 and ξ2 cause a share of the variance in the conceptual variable η; the researcher believes that the unobservable conceptual variables ξ1 and ξ2 cause variation in their respective observed variables x11, x12, x13 and x21 and x22. Additionally, he/she believes that the conceptual variable η causes the full variation in its observed variable y, i.e. y is a perfect measurement of η; the fictitious researcher believes that the remaining variances in the observed variables x11 to x22 and the conceptual variable η are caused by other unobserved variables ε11 to ε22 and ζ. Further, he/she claims that these unobserved causes are not correlated with one another. Similarly, the fictitious researcher postulates that the unobserved causes ε11 to ε22 are uncorrelated with the conceptual variables, and that the unobserved cause ζ is uncorrelated with the conceptual variables ξ1 and ξ2.
The assumed data generating process, i.e. the population model, is presented at the top of Figure 1. To preserve clarity, the variances of the disturbance terms are rounded to second decimal place. Thus, the world indeed functions according to the researcher’s beliefs. Subsequently, the researcher specifies a structural equation model in accordance with his/her theory and applies PLS and PLSc to estimate the relationships between the variables. Additionally, the researcher applies the ML estimator (Jöreskog, 1969, 1970), which is widely acknowledged for estimating structural models containing latent variables and thus serves as a reference. To avoid issues caused by sampling variation, let us say that the researcher uses the population variance-covariance as input for the three estimators. Thus, the parameters are actually not estimated, but calculated.
As shown in Figure 1 and discussed in the literature, PLS is not able to retrieve the population parameters. Cadogan and Lee are thus correct that PLS is hardly suitable for estimating structural models containing latent variables, because even if a researcher uses the entire population to calculate the parameters, he/she would draw the wrong conclusions from the model. In contrast to PLS, both ML and PLSc are able to retrieve the parameters of the population model, and they produce identical results.
Considering the statistical properties of ML and PLSc, the two are very similar. Both ML and PLSc are consistent and asymptotically normal (Bollen, 1989; Dijkstra and Henseler, 2015b). Consequently, for an increasing sample size, ML and PLSc estimates converge in probability to the population values if the model is correctly specified. In fact, the main difference between their properties is that ML estimates are known to be asymptotically efficient (Bollen, 1989), while this has not been shown for PLSc estimates. However, various simulation studies have demonstrated that ML and PLSc show a similar finite sample behavior (Dijkstra and Henseler, 2015b; Yuan et al., 2020). The same behavior can be observed for more complex structural models containing quadratic and interaction effects of latent variables. In this case, PLSc performs similarly to latent moderated structural equation modeling (Dijkstra and Schermelleh-Engel, 2014). The latter is an ML estimator for models containing interaction terms of latent variables (Klein and Moosbrugger, 2000).
Against the background that both PLSc and ML produce the exact same results on a population level, show very similar statistical properties and behave similarly in finite samples; it is not clear why PLSc would be disqualified as a method for scientific realist inquiry. Further, it is not clear why the model estimated by PLSc “contains no theoretical causal contact between unobservable conceptual variables and data” (Cadogan and Lee, 2022), whereas the same model, but estimated by ML, does. Cadogan and Lee also broadly derogate the principle of correction for attenuation. In their words, “dividing b, the observed relationship between X and Y, by some denominator does little to pacify the realist’s concerns on this front.” There are several issues here. First, Cadogan and Lee seem to misunderstand how the correction for attenuation is executed in PLSc: not the path coefficients b between two proxies, but their correlation should be divided by the geometric mean of their reliabilities. Second, correction for attenuation is an established psychometric approach dating back to Spearman (1904b), which should not simply be discarded by a coup de main. Third, rejecting correction for attenuation would also imply that all approaches relying on proxies for latent variables, such as factor scores regression with a correction for attenuation (Wall and Amemiya, 2000; Devlieger and Rosseel, 2017), should be rejected for scientific realist inquiry. Apparently, Cadogan and Lee do not follow a common understanding in statistics that distinguishes between the estimator and the estimand (the estimated quantity, see Mosteller and Tukey, 1987), and they overlook the fact that it matters little how an estimator arrives at an estimate, as long as it has certain statistical properties.
2.2 Not all conceptual variables have to be measured; some can be built
The realist variable framework assumes that unobservable conceptual variables cause the variance in their associated observed variables, and it relies on the reflective measurement model to model these relationships statistically. However, in various disciplines conceptual variables are assumed to be formed by or to emerge from a set of variables within their environment. For instance, gene and brain regions can be regarded as biological composites of single nucleotide polymorphisms or voxels, respectively (Jung et al., 2012, 2016; Romdhani et al., 2014). Similarly, stress and job performance can be regarded as concpets that emerge as linear combinations from a set of variables (Hancock, 1997; Murphy and Shiarella, 1997).
The measurement theory (Spearman, 1904a) and its template, the reflective measurement model, are hardly suitable to operationalize conceptual variables that are not measured, but are formed. Against this background, researchers have proposed other approaches and auxiliary theories to model and assess such conceptual variables. For instance, the composite model was proposed to model conceptual variables that are aggregated (Edwards, 2001) or formed (Henseler, 2017; Schuberth et al., 2018a; Henseler, 2021). Analogous to measurement theory, Henseler and Schuberth (2021) have introduced synthesis theory as an auxiliary theory for formed conceptual variables. In a statistical model, formed conceptual variables are represented by emergent variables (Coan and Gonzalez, 2015; Henseler and Schuberth, 2021; Yu et al., 2021), not latent variables [1].
The composite model assumes that there is “a definitorial relation between a construct and its indicators. This means that the construct is made up of its indicators or elements” (Henseler, 2017, p. 179). Further, the model assumes that the variables forming the construct act along a single dimension, i.e. all the information between the blocks of observed variables is conveyed solely by the composites (Dijkstra, 2017). Hence, the composite model can help us to understand whether the ingredients make up a new coherent whole or whether they are simply a random collection of elements. This idea resembles the act of design in which designers arrange ingredients to create something new.
On the one hand, the composite model does not have immediate implications for realists, because if the ingredients exist, so does the composite. On the other hand, the composite model can help us to understand whether a set of elements act along a single dimension, which corresponds to an emergent property of the composite.
There are various estimators that can be used to consistently estimate composite models: not only PLS but also generalized structured component analysis (GSCA, Hwang and Takane, 2004) and Kettenring’s (1971) approaches to generalized canonical correlation analysis, such as the maximum variance method (MAXVAR) (Dijkstra, 2017; Cho et al., 2020).
Figure 2 juxtaposes the performance of PLS, GSCA and MAXVAR for a composite model. The model is similar to the structural model containing latent variables from Figure 1, but the constructs ξ1, ξ2 and η are modeled as emergent variables, not as latent variables. As shown in Figure 2, all three estimators are capable of retrieving the parameters of the population model.
3. Partial least squares as an estimator for structural equation modeling vs partial least squares-structural equation modeling
Cadogan and Lee (2022) refer to PLS as “the kind of PLS modelling of the sort that Hair et al. (2019b) and Dijkstra and Henseler (2015b) promote” (Footnote 7). This explanation could be understood to imply that Hair et al. (2019b) and Dijkstra and Henseler (2015b) work with the same understanding of PLS. However, little could be further from truth [2]. The difference between the two understandings of PLS is highlighted by Hair et al. (2019b, p. 570) who stated that Dijkstra and Henseler’s (2015b) PLSc “adds very little to the body of knowledge, and by its name is deceptive,” thus distancing themselves from Dijkstra and Henseler (2015a). Whereas Dijkstra and Henseler (2015a, 2015b) regard PLSc and PLS as estimators for SEM, Hair et al. (2019b) regard PLS-SEM (including the method of confirming measurement quality, Hair et al., 2020; Schuberth, 2021) as an overarching approach. Therefore, one cannot pose just one conceptualization of PLS that the two groups of scholars jointly promote. Figure 3 visualizes these different understandings of PLS.
Three features of Figure 3 are particularly worth noticing. As the shaded areas emphasize, Dijkstra and Henseler (2015a, 2015b) and subsequent publications (Henseler et al., 2016; Henseler, 2017; Müller et al., 2018; Henseler, 2021; Benitez et al., 2020) ascribe a relatively small (but nevertheless important) role to PLS, PLSc, PLSe1, OrdPLS and the likes within SEM. The different PLS approaches serve solely as estimators in SEM. The estimator should be chosen according to the specified model, i.e. PLS for models relating composites and PLSc for models relating latent variables (Henseler, 2017). In contrast, PLS-SEM as promoted by Hair et al. (2011, 2012b, 2012c, 2014, 2017a, 2017b, 2019a, 2019b) is regarded as an overarching approach, method, technique or analytic framework (Ringle et al., 2012), and therefore not just a part of SEM. PLS-SEM has its own kind of model (PLS models) and its own model evaluation steps (Hair et al., 2020). A second major distinction between SEM and PLS-SEM is the aspect of model identification. As PLS and PLSc serve only as estimators within SEM, model identification remains a crucial step, also when PLS or PLSc are used (Henseler, 2021). As one means of ensuring model identification, Henseler et al. (2016) introduced the dominant indicator approach, which fixes the sign of one indicator loading. In contrast, PLS-SEM is claimed to have “no identification problems” (Hair et al., 2017b, p. 18); it is “not constrained by identification and other technical issues” (Hair et al., 2017b, p. 27). Finally, whereas the assessment of overall model fit is a crucial element of SEM, it does not form part of PLS-SEM’s canonical list of stages. See also Section 5.
4. Redefining reflective measurement in partial least squares-structural equation modeling
Reflective measurement as represented by a common factor model is the dominant approach to model unobservable conceptual variables. In line with Cadogan and Lee (2022), “if one subscribes to realism, then the common factor model maps over the realist’s conceptual model, in which unobservable and observable variables are causally linked.” Figure 4 depicts a common factor model with reflective indicators. This appears to correspond with the understanding in PLS-SEM in which for:
[…] reflective indicators, the direction of the arrows is from the construct to the indicator variables, indicating the assumption that the construct causes the measurement (more precisely, the covariation) of the indicator variables (Hair et al., 2017b, p. 13).
Yet, PLS-SEM, which relies on PLS, does not consistently estimate parameters of a common factor model. See also Section 2.1. PLS-SEM proponents appear to be well aware of this fact. For instance, PLS-SEM represents constructs as linear combinations of its indicators (Sarstedt et al., 2016). Similarly, “[i]nstead of following a common factor model logic […], PLS-SEM calculates composites of indicators that serve as proxies for the concpets [sic] under research” (Hair et al., 2017b, p. 33).
In case of reflective measurement, PLS-SEM relies on PLS Mode A as an outer weighting scheme. PLS Mode A yields indicator weights that are proportional to the true common factor loadings (Dijkstra, 2015). The statistical model underlying PLS Mode A is depicted in Figure 5. The indicator weights are constrained to be proportional to the true loadings (using a proportionality factor c such that the variance of ξ equals 1). As can be seen, the construct ξ in Figure 5 differs from the construct ξ in Figure 4. Concretely, whereas the construct ξ in Figure 4 is measured without measurement error, the construct ξ in Figure 5 does contain a measurement error, the amount of which can be determined as:
Although PLS-SEM proponents seem to be aware of the fact that PLS Mode A is different from the reflective measurement model that SEM has put forward, they continue to equate the two models and pretend that PLS-SEM is appropriate for reflective measurement models. For instance, in their glossary Hair et al. (2017b) explain reflective measurement as:
[…] a type of measurement model setup in which measures represent the effects (or manifestations) of an underlying construct. Causality is from the construct to its measures (indicators). Also referred to as Mode A in PLS-SEM.
Similarly, Hair et al. (2017c) confirm that PLS-SEM “[h]andles reflectively […] specified constructs” (p. 620) and according to Hair et al. (2017b, p. 19), PLS-SEM “[e]asily incorporates reflective […] measurement models.” Consequently, there is a large risk for researchers applying PLS-SEM that the actual statistical model does not match their theory.
This problematic practice of equating PLS Mode A with reflective measurement becomes most evident in the writings of Hair et al.; even so, one comes across it in a large part of the PLS literature. In the light of this situation, Henseler (2021) warns that some highly cited PLS-related publications exhibit the same problem. See, for example, Cassel et al. (1999), Chin (1998), Chin et al. (2003), Esposito Vinzi et al. (2010), Haenlein and Kaplan (2004), Hair et al. (2017a), Hair et al. (2012a; 2013), Hair et al. (2014), Hair et al. (2012b), Hair et al. (2017a), Hair et al. (2017d), Hair et al. (2012c), Henseler et al. (2009), Hulland (1999), Lowry and Gaskin (2014), Peng and Lai (2012), Reinartz et al. (2009), Ringle et al. (2012), Sarstedt et al. (2014), Sosik et al. (2009) and Tenenhaus et al. (2005). Against this background, Cadogan and Lee (2022) are right in finding “PLS[-SEM]’s symbolic language maps over that of common cause SEM, making it harder to recognize that PLS[-SEM] does not adopt a realist stance.”
5. Assessment of model fit
Cadogan and Lee (2022) emphasize the importance of “methods which at least explicitly propose data generating mechanisms, and which when used appropriately can ‘test’ the mechanisms against observable data” for scientific realists. In the context of SEM, testing the mechanisms against observable data involves the assessment of overall model fit, which means that the discrepancy between the sample variance-covariance matrix and the estimated model-implied counterpart is investigated. This is also promoted for models estimated by PLSc (Dijkstra and Henseler, 2015a; Benitez et al., 2020). Against this background, it is surprising that Cadogan and Lee did not discuss this opportunity for structural models containing latent variables estimated by PLSc. As Dijkstra and Henseler (2015b) proposed, structural equation models containing latent variables estimated by PLSc can be assessed for overall model fit by a bootstrap-based test. This approach is not tied to PLSc and also finds application in the SEM literature under the name “Bollen-Stine bootstrap” (Bollen and Stine, 1992). To draw a conclusion about whether the specified model shows a perfect fit in the population, the bootstrap-based test – as its name suggests – relies on the bootstrap. Specifically, it compares the value of a discrepancy function that measures the difference between the observed variables’ sample variance-covariance and their model-implied counterpart, to a critical value obtained from the bootstrap reference distribution of this discrepancy measure. Notably, the bootstrap procedure is based on a transformed data set to ensure that the observed variables’ sample variance-covariance matrix agrees with the specified model, i.e. the variance–covariance matrix of the transformed data set equals the variance–covariance matrix the estimated model implies. Although Beran and Srivastava (1985) have derived the statistical properties of the bootstrap-based test, a simulation study additionally confirmed that the bootstrap-based test is suitable for structural equation models containing latent variables estimated through PLS (Dijkstra and Henseler, 2015a).
In contrast to the literature that regards PLS and PLSc as estimators within SEM (Dijkstra and Henseler, 2015b; Henseler et al., 2016; Benitez et al., 2020), overall model fit assessment is ascribed little value in PLS-SEM (Hair et al., 2019a, 2020). Instead, PLS-SEM relies on a set of heuristic rules to confirm measurement models and assess structural models (Hair et al., 2020). Particularly the notion that (measurement) models can/should/must be confirmed (Hair et al., 2020) is at odds with the Popperian dictum that models can never be confirmed, only rejected. In the past, various researchers have criticized the use of the assessment criteria for reflective measurement models in PLS-SEM (Rönkkö et al., 2016; Schuberth, 2021). This is mainly due to the following reasons. First, as shown in the previous section, the reflective measurement model in PLS-SEM differs from the reflective measurement model SEM put forward. As the assessment criteria used to confirm PLS-SEM reflective measurement models were developed under the assumption that the reflective measurement model is true, their validity for evaluating PLS-SEM reflective measurement models is limited. Second, PLS-SEM applies PLS to estimate reflective measurement models’ parameters, which is known to produce inconsistent estimates for this type of model. Consequently, only questionable conclusions can be drawn from these criteria about reflective measurement models, as they are based on inconsistent PLS estimates.
In addition to assessing the overall fit of structural models that include latent variables, the overall fit of models with emergent variables can also be assessed (Dijkstra, 2017; Schuberth et al., 2018b). Composite models’ overall fit assessment relies on the same principle as latent variable models’ assessment, i.e. the difference between the observed variables’ sample variance-covariance and their estimated model-implied counterpart is examined. However, instead of applying the variance-covariance implied by a latent variable model, the model-implied variance-covariance of the composite model needs to be considered. For more details about the assessment of composite models, the interested reader is referred to Dijkstra (2017) and Schuberth et al. (2022).
6. Discussion
The focal point of Cadogan and Lee’s treatise was the extent to which PLS is an appropriate method for researchers who adhere to a particular research paradigm. While many researchers might subscribe to only a single research paradigm, electing to conduct a study that includes multiple research paradigms requires empathy and understanding of all research paradigms covered. Not only do Cadogan and Lee manage this challenge well, they also demonstrate that they subscribe to all four covered research paradigms.
Obviously, scientific realism is close to Cadogan and Lee’s hearts. Not only do they devote their paper to realists’ research quality; they are also confident that they are not alone with this worldview, because “many marketers embrace the realist stance” (Cadogan and Lee, 2022).
In past publications, both Cadogan and Lee pursued an instrumentalist worldview. If structural equation models turn out to be significantly wrong, but researchers nevertheless deem them adequate for drawing conclusions about the world (as for instance in the following papers Cadogan or Lee co-authored: Boso et al., 2013; Cadogan et al., 2009; Hooley et al., 2005), then they pursue an antirealist paradigm. Under such circumstances, that the researchers used a model as a tool to better understand the world, identifies them as instrumentalists.
The study of Cadogan and Lee itself is clearly of a pragmatist nature. The research question about PLS’s suitability for researchers following a certain paradigm is all about the usefulness of PLS and not about its truth or existence. Moreover, Cadogan and Lee’s (2022) final and most pronounced conclusion is normative: “[T]he method […] should not be PLS.” This is a pragmatist worldview par excellence: In order to achieve purpose Y [here: realist inquiry], follow the norm X [here: “Do not use PLS”].
Although readers could get the impression that the group of researchers Cadogan and Lee would least want to adhere to are the constructivists, in some instances they exhibit behavior that they ascribed to constructivists, namely, “construct different stories of the world” (Cadogan and Lee, 2022, Abstract). Some of these constructed stories result from failure to distinguish between PLS as an estimator of structural equation models on the one hand, and PLS-SEM on the other hand. Other constructed stories emerge from the practice of contextomy, thus distorting the source’s intended meaning. Cadogan and Lee ascribe statements and values to other researchers that are at odds to those researchers’ real statements and opinions. Table 2 lists a selection of questionable statements Cadogan and Lee made and juxtaposes them with corresponding corrections.
Cadogan and Lee (2022) make an important contribution by pointing to the interrelation between scientific paradigms and highlighting that the use of a certain method might mean different things to researchers who follow different paradigms. The starting point for this commentary was Cadogan and Lee’s (2022) central statement that PLS subverts realist search for truth. The previous sections have shown that this statement is not universally true. If researchers carefully specify their model and use consistent estimators, that can be based on PLS, there is no subversion whatsoever. Table 3 summarizes the conclusions about PLS estimators’ suitability for different research paradigms. It also makes it visible that not the estimator makes a difference, but the chosen model. Finally, this commentary concludes in the form of three recommendations.
Recommendation 1: Researchers should select a model that adequately represents their theory.
Researchers should select a statistical model that best describes their theory. If their theory is about unobservable conceptual variables that are measured by a set of observed variables as in the realist variable framework, the reflective measurement model might be a suitable candidate. Notably, recent literature has urged caution to reflexively use the common factor model in modeling unobservable conceptual variables (Rhemtulla et al., 2020). Moreover, if the theory is about conceptual variables that are formed or aggregated, the composite model appears to be a more suitable alternative for modeling such theories. To avoid misunderstandings, researchers should formulate their model explicitly.
Recommendation 2: Researchers should carefully reflect on the properties of an estimator for a certain model and not bother about whether the estimator is called “PLS.”
Various estimators have been proposed for structural models containing latent variables, such as the ML estimator, the generalized least squares estimator, PLS and PLSc. In deciding about whether to use an estimator for a certain model, researchers should consider the estimator’s statistical properties for this model. Desirable estimator properties are (asymptotic) unbiasedness, consistency and (asymptotic) efficiency [3]. As shown in Section 2.1, PLS does not show any of these properties for structural models containing latent variables. Therefore, researchers are advised not to use PLS to estimate this type of model. However, PLSc was specifically developed to estimate latent variable models and has very similar statistical properties as the ML estimator, i.e. it is consistent and asymptotically normal. Hence, there is nothing that speaks against using PLSc to estimate a latent variables model. To conclude, researchers:
[…] should check any tool that they may be considering using, to establish its correspondence with their commitments regarding the reality of the conceptual variables and causal forces in their theories (Cadogan and Lee, 2022).
This includes inspecting the suitability of the employed estimator for the specified model.
Recommendation 3: Researchers should critically assess their model.
If SEM is used to describe phenomena of the world, researchers should do their utmost to examine whether the model is a good description by exploiting the constraints the model imposes. One way to do so is to assess the overall model fit by means of statistical testing, regardless of whether the conceptual variables are modeled as latent variables or as emergent variables. As various simulation studies demonstrated, the overall fit of latent variable models and composite models estimated by PLSc and PLS, respectively, can be assessed.
Figures
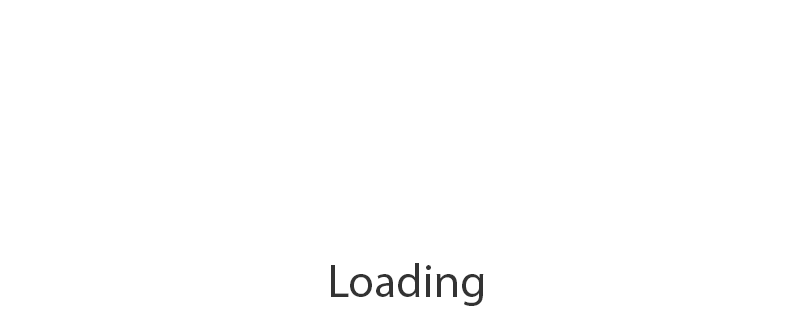
Figure 3.
Two different conceptions of PLS: PLS as an estimator in SEM (Henseler, 2021) vs PLS-SEM as an overarching framework (Hair et al., 2017b)
Estimators for structural equation models based on PLS
Estimator | Inventor(s) | Purpose |
---|---|---|
PLS | Wold (1974, 1975, 1982) | A computationally efficient but inconsistent estimator for structural equation models containing latent variables (however, it provides consistent estimates for the composite model) |
ordinal PLS | Cantaluppi and Boari (2016) | A modification of PLS that can cope with ordinal categorical observed variables in a psychometric way |
robust PLS | Schamberger et al. (2020) | A modification of PLS that can cope with unsystematic outliers |
PLSc | Dijkstra and Henseler (2015a, 2015b) | An extension of PLS that provides consistent estimates for structural models containing latent variables |
PLSe1 | Huang (2013) | A one-step improvement methodology based on PLSc-estimated factor loadings and 2SLS-estimated structural parameters |
PLSe2 | Huang (2013) | An optimal generalized least squares methodology using a PLSc-implied covariance matrix |
ordinal PLSc | Schuberth et al. (2018b) | A modification of PLSc that can cope with ordinal categorical observed variables in a psychometric way |
robust PLSc | Schamberger et al. (2020) | A modification of PLSc that can cope with unsystematic outliers |
PLSc via regularization | Jung and Park (2018) | A modification of PLSc that can cope with multicollinearity issues in the structural model |
Some corrections to Cadogan and Lee (2022)
Questionable statement of Cadogan and Lee (2022) | Proposed correction |
---|---|
“The outputs that PLS produces lie outside the scope of scientific realist inquiry” | Like the ML estimator, PLSc produces consistent estimates for reflective measurement models. Hence, if SEM using ML is suitable for scientific realist inquiry, SEM using PLSc should also be regarded as suitable. Traditional PLS produces estimates for composite models. Scientific realism stays neutral with regard to composition |
“PLS … is uniquely designed to construct different stories of the world depending on the research context” | PLS is designed to create composites of observed variables of which the correlation matrix has ‘maximum distance’ to the unit matrix |
“[R]ecent work by PLS experts explicitly attempts to link PLS with constructivism.” “… Henseler’s (2017) observation that tools such as PLS are consistent with a constructivist perspective.” “Henseler’s (2017) view of tools like PLS as aligning with constructivism, rather than realism …” | Henseler (2017) suggested that the composite model aligns well with constructivism. Composite models can be estimated using, among others, ML and PLS, which means that ML and PLS are equally strongly linked to constructivism. Henseler (2017) did not view PLS as aligning less with realism |
“[T]he numerical results, predictions, and relationships that the PLS method returns are not estimates of real world things, but rather, are explicitly constructed by the analysis method, and hence the analysts themselves” | Every statistical method’s numerical results are produced by the analysis method. PLSc provides consistent parameter estimates for reflective measurement models (Dijkstra and Henseler, 2015a, 2015b), and PLS Mode B provides consistent parameter estimates for composite models (Dijkstra, 2017). Consistency means that estimates converge in probability toward the true value of the parameters given the model is correctly specified |
“Further, some PLS advocates agree, identifying PLS as an approach that is entirely constructivist, located in a world where researchers modeling with a ‘composite can be thought of as designers: They design this construct’, explicitly mixing up ‘ingredients … [and arranging them] to form a new entity’ (Henseler, 2017, p. 180), rather than explicitly attempting to measure unobservable variables that ‘exist in nature’ (Henseler, 2017, p. 178). This constructivist interpretation has seriously problematic implications for realists …” (p. 23) | It is questionable whether anybody would agree that PLS is an entirely constructivist approach. Henseler (2017) definitely did not identify it as such. Henseler (2017) recommended specifying reflective measurement models for unobserved conceptual variables that are assumed to exist in nature, and then estimating the reflective measurement model parameters using PLSc. He recommended the composite model as a representation for human-made concepts. Composite models assume “a definitorial relation between a construct and its indicators. This means that the construct is made up of its indicators or elements” (Henseler, 2017, p. 179). In composite models, “the relationships between the indicators and the construct are not cause-effect relationships but rather a prescription of how the ingredients should be arranged to form a new entity” (Henseler, 2017, p. 180). Not all researchers modeling with a composite can be thought of as designers; only those “who introduce a composite can be thought of as designers” (Henseler, 2017, p. 181, emphasis added) |
“PLSc’s use of common factors does not elevate PLS to a method that meets the realist’s aspirations for hypothetical causal contact” | Since “causal contact” is a property of a model and not an estimator, and PLSc estimates for reflective measurement models do not differ qualitatively from other estimators for reflective measurement models, SEM with PLSc is a viable methodological option for realists |
“[T]he design of PLS means that it will produce different results as a consequence of the varying contexts (e.g., social environments) in which it is undertaken” | The results of PLS remain the same no matter who undertakes the analysis. However, as with maximum likelihood, PLS takes the whole model into account, which means that a construct’s factor loadings can differ if different variables are related to it |
“[T]hings that are constructed, like the results from a PLS analysis” | In structural equation modeling, model parameters are estimated, not constructed, no matter which estimator (ML, generalized least squares, unweighted least squares, PLS, etc.) is used |
“ Nelson and Stolterman (2012) describe the world in archetypally constructivist terms: for instance they claim that ‘[h]umans did not discover fire – they designed it’ (p. 11), and thus that ‘scientists … can be understood more as design critics than natural scientists’ (p. 27)” | Nelson and Stolterman (2012, p. 11) do not describe the world, but human achievements: “Humans did not discover fire – they designed it. The wheel was not something our ancestors merely stumbled over in a stroke of good luck; it, too, was designed. The habit of labeling significant human achievements as ‘discoveries,’ rather than ‘designs,’ discloses a critical bias in our Western tradition whereby observation dominates imagination.” While constructivists hold that knowledge about the world is constructed (Lyotard, 1984), Nelson and Stolterman (2012) make the point that a large part of today’s world is constructed. At the same time, they do not regard scientific knowledge as being designed: “In the theoretical world of science, we do not think about natural laws or truths as being designed. But, in the real world – the present environment that surrounds all of us – we understand that we ‘create’ as well as ‘discover’ this reality. This is because the real world has many facets of an artificial world and is very much a designed world. In fact scientists have begun to label the present epoch as the Anthropocene era because of the dominant effect human activity has had on global systems, making them ever more unnatural and artificial. Based on this, scientists describing and explaining the world can be understood more as design critics than natural scientists” (p. 27) |
“ Henseler (2017) affirms that PLS’s compositions, and the relationships it creates between those compositions, belong in the realm of ‘critical design’, where PLS users essentially ‘imagine that-which-does-not-yet-exist, to make it appear’ Nelson and Stolterman (2012, p. 12)” | Neither Henseler (2017) nor Nelson and Stolterman (2012) use the term ‘critical design,’ so Cadogan and Lee’s claim cannot be affirmed. Nelson and Stolterman (2012) do not say anything about PLS users, but equate design with “the ability to imagine that-which-does-not-yet-exist” (p. 12) |
Suitability of PLS-based methods (combination of model and estimator) per research paradigm
Method | Suitable for […] | ||||
---|---|---|---|---|---|
Model | Estimator | Realism | Instrumentalism | Pragmatism | Constructivism |
Reflective measurement model | PLS | ✗ | ✗ | ✗ | ✗ |
PLSc | ✓ | ✓ | ✓ | (✓) | |
ordinal PLSc | ✓ | ✓ | ✓ | (✓) | |
robust PLSc | ✓ | ✓ | ✓ | (✓) | |
PLSe1 | ✓ | ✓ | ✓ | (✓) | |
PLSe2 | ✓ | ✓ | ✓ | (✓) | |
Composite model | PLS | (✓) | ✓ | ✓ | ✓ |
ordinal PLS | (✓) | ✓ | ✓ | ✓ | |
robust PLS | (✓) | ✓ | ✓ | ✓ |
✓ = suitable, (✓) = potentially suitable, ✗ = not suitable
Notes
The notion of an emergent variable has been chosen to emphasize that the variable is not simply a composite, but a composite that conveys all the information between its components and its consequences, and that it is on the same level of abstraction as a latent variable.
Since Theo K. Dijkstra passed away in 2020, he can no longer confirm this personally. However, based on prior personal communication with him, the authors are certain that Dijkstra’s view on PLS was completely different to that of Hair et al. (2019b).
This does not mean that estimators lacking these properties are intrinsically “bad”. For instance, there are situations in which researchers deliberately sacrifice certain properties, such as unbiasedness, to obtain estimates with a smaller standard error.
References
Bagozzi, R.P., Fornell, C. and Larcker, D.F. (1981), “Canonical correlation analysis as a special case of a structural relations model”, Multivariate Behavioral Research, Vol. 16 No. 4, pp. 437-454.
Benitez, J., Henseler, J., Castillo, A. and Schuberth, F. (2020), “How to perform and report an impactful analysis using partial least squares: guidelines for confirmatory and explanatory is research”, Information and Management, Vol. 2 No. 57, p. 103168.
Beran, R. and Srivastava, M.S. (1985), “Bootstrap tests and confidence regions for functions of a covariance matrix”, The Annals of Statistics, Vol. 13 No. 1, pp. 95-115.
Bollen, K.A. (1989), Structural Equations with Latent Variables, John Wiley & Sons, New York, NY.
Bollen, K.A. (2001), “Two-stage least squares and latent variable models: simultaneous estimation and robustness to misspecifications”, in Cudeck, R., Du Toit, S. and Sörbom, D. (Eds), Structural Equation Modeling: Present and Future, a Festschrift in Honor of Karl Jöreskog, Scientific Software, Chicago, pp. 119-138.
Bollen, K.A. and Stine, R.A. (1992), “Bootstrapping goodness-of-fit measures in structural equation models”, Sociological Methods and Research, Vol. 21 No. 2, pp. 205-229.
Boso, N., Story, V.M. and Cadogan, J.W. (2013), “Entrepreneurial orientation, market orientation, network ties, and performance: study of entrepreneurial firms in a developing economy”, Journal of Business Venturing, Vol. 28 No. 6, pp. 708-727.
Browne, M.W. (1984), “Asymptotically distribution-free methods for the analysis of covariance structures”, British Journal of Mathematical and Statistical Psychology, Vol. 37 No. 1, pp. 62-83.
Cadogan, J.W. and Lee, N. (2022), “A miracle of measurement or accidental constructivism? How PLS subverts the realist search for truth”, European Journal of Marketing, this issue.
Cadogan, J.W., Lee, N., Tarkiainen, A. and Sundqvist, S. (2009), “Sales manager and sales team determinants of salesperson ethical behaviour”, European Journal of Marketing, Vol. 43 Nos 7/8, pp. 907-937.
Cantaluppi, G. and Boari, G. (2016), “A partial least squares algorithm handling ordinal variables”, in Abdi, H., Esposito Vinzi, V., Saporta, G., Russolillo, G. and Trinchera, L. (Eds), The Multiple Facets of Partial Least Squares and Related Methods, Springer, Cham, pp. 295-306.
Cassel, C., Hackl, P. and Westlund, A.H. (1999), “Robustness of partial least-squares method for estimating latent variable quality structures”, Journal of Applied Statistics, Vol. 26 No. 4, pp. 435-446.
Chin, W.W. (1998), “The partial least squares approach for structural equation modeling”, in Marcoulides, G. (Ed.), Modern Methods for Business Research, Psychology Press, New York, NY, pp. 295-336.
Chin, W.W., Marcolin, B.L. and Newsted, P.R. (2003), “A partial least squares latent variable modeling approach for measuring interaction effects: results from a Monte Carlo simulation study and an electronic-mail emotion/adoption study”, Information Systems Research, Vol. 14 No. 2, pp. 189-217.
Cho, G. and Choi, J.Y. (2020), “An empirical comparison of generalized structured component analysis and partial least squares path modeling under variance-based structural equation models”, Behaviormetrika, Vol. 47 No. 1, pp. 243-272.
Cho, G., Hwang, H., Sarstedt, M. and Ringle, C.M. (2020), “Cutoff criteria for overall model fit indexes in generalized structured component analysis”, Journal of Marketing Analytics, Vol. 8 No. 4, pp. 189-202.
Coan, J.A. and Gonzalez, M.Z. (2015), “Emotions as emergent variables”, in Barrett, L.F. and Russell, J.A. (Eds), The Psychological Construction of Emotion, Guilford Press, New York, NY, pp. 209-225.
Devlieger, I. and Rosseel, Y. (2017), “Factor score path analysis”, Methodology, Vol. 13 No. Supplement 1, pp. 31-38.
Dijkstra, T.K. (1985), Latent Variables in Linear Stochastic Models: Reflections on “Maximum Likelihood” and “Partial Least Squares” Methods, Vol. 1, Sociometric Research Foundation, Amsterdam.
Dijkstra, T.K. (2013), “Composites as factors: canonical variables revisited”, Working Paper.
Dijkstra, T.K. (2015), “All-inclusive versus single block composites”, Working Paper, pp. 1-15.
Dijkstra, T.K. (2017), “A perfect match between a model and a mode”, in Latan, H. and Noonan, R. (Eds), Partial Least Squares Path Modeling: Basic Concepts, Methodological Issues and Applications, Springer, Cham, pp. 55-80.
Dijkstra, T.K. and Henseler, J. (2015a), “Consistent and asymptotically normal PLS estimators for linear structural equations”, Computational Statistics and Data Analysis, Vol. 81 No. 1, pp. 10-23.
Dijkstra, T.K. and Henseler, J. (2015b), “Consistent partial least squares path modeling”, MIS Quarterly, Vol. 39 No. 2, pp. 297-316.
Dijkstra, T.K. and Schermelleh-Engel, K. (2014), “Consistent partial least squares for nonlinear structural equation models”, Psychometrika, Vol. 79 No. 4, pp. 585-604.
Edwards, J.R. (2001), “Multidimensional constructs in organizational behavior research: an integrative analytical framework”, Organizational Research Methods, Vol. 4 No. 2, pp. 144-192.
Esposito Vinzi, V., Chin, W.W., Henseler, J. and Wang, H. (2010), “Editorial: perspectives on partial least squares”, in Esposito Vinzi, V., Chin, W.W., Henseler, J. and Wang, H. (Eds), Handbook of Partial Least Squares: Concepts, Methods and Applications, Springer, Berlin, Heidelberg, pp. 1-20.
Haenlein, M. and Kaplan, A.M. (2004), “A beginner’s guide to partial least squares analysis”, Understanding Statistics, Vol. 3 No. 4, pp. 283-297.
Hair, J.F., Ringle, C.M. and Sarstedt, M. (2011), “PLS-SEM: indeed a silver bullet”, Journal of Marketing Theory and Practice, Vol. 19 No. 2, pp. 139-152.
Hair, J.F., Ringle, C.M. and Sarstedt, M. (2012a), “Editorial: partial least squares: the better approach to structural equation modeling?”, Long Range Planning, Vol. 45 Nos 5/6, pp. 312-319.
Hair, J.F., Sarstedt, M., Pieper, T.M. and Ringle, C.M. (2012b), “The use of partial least squares structural equation modeling in strategic management research: a review of past practices and recommendations for future applications”, Long Range Planning, Vol. 45 Nos 5/6, pp. 320-340.
Hair, J.F., Sarstedt, M., Ringle, C.M. and Mena, J.A. (2012c), “An assessment of the use of partial least squares structural equation modeling in marketing research”, Journal of the Academy of Marketing Science, Vol. 40 No. 3, pp. 414-433.
Hair, J.F., Ringle, C.M. and Sarstedt, M. (2013), “Editorial: partial least squares structural equation modeling: rigorous applications, better results and higher acceptance”, Long Range Planning, Vol. 46 Nos 1/2, pp. 1-12.
Hair, J.F., Sarstedt, M., Hopkins, L. and Kuppelwieser, V.G. (2014), “Partial least squares structural equation modeling (PLS-SEM): an emerging tool in business research”, European Business Review, Vol. 26 No. 2, pp. 106-121.
Hair, J.F., Hollingsworth, C.L., Randolph, A.B. and Chong, A.Y.L. (2017a), “An updated and expanded assessment of PLS-SEM in information systems research”, Industrial Management and Data Systems, Vol. 117 No. 3, pp. 442-458.
Hair, J.F., Hult, G.T.M., Ringle, C.M. and Sarstedt, M. (2017b), A Primer on Partial Least Squares Structural Equation Modeling (PLS-SEM), 2nd ed., Sage, London.
Hair, J.F., Hult, G.T.M., Ringle, C.M., Sarstedt, M. and Thiele, K.O. (2017c), “Mirror, mirror on the wall: a comparative evaluation of composite-based structural equation modeling methods”, Journal of the Academy of Marketing Science, Vol. 45 No. 5, pp. 616-632.
Hair, J.F., Sarstedt, M., Ringle, C.M. and Gudergan, S.P. (2017d), Advanced Issues in Partial Least Squares Structural Equation Modeling, Sage, Thousand Oaks, CA.
Hair, J.F., Risher, J.J., Sarstedt, M. and Ringle, C.M. (2019a), “When to use and how to report the results of PLS-SEM”, European Business Review, Vol. 31 No. 1, pp. 2-24.
Hair, J.F., Sarstedt, M. and Ringle, C.M. (2019b), “Rethinking some of the rethinking of partial least squares”, European Journal of Marketing, Vol. 53 No. 4, pp. 566-584.
Hair, J.F., Howard, M.C. and Nitzl, C. (2020), “Assessing measurement model quality in PLS-SEM using confirmatory composite analysis”, Journal of Business Research, Vol. 109, pp. 101-110.
Hancock, G.R. (1997), “Structural equation modeling methods of hypothesis testing of latent variable means”, Measurement and Evaluation in Counseling and Development, Vol. 30 No. 2, pp. 91-105.
Henseler, J. (2017), “Bridging design and behavioral research with variance-based structural equation modeling”, Journal of Advertising, Vol. 46 No. 1, pp. 178-192.
Henseler, J. (2021), Composite-Based Structural Equation Modeling: Analyzing Latent and Emergent Variables, Guilford Press, New York.
Henseler, J. and Schuberth, F. (2020), “Using confirmatory composite analysis to assess emergent variables in business research”, Journal of Business Research, Vol. 120, pp. 147-156.
Henseler, J. and Schuberth, F. (2021), “Auxiliary theories”, in Henseler, J. (Ed.), Composite-Based Structural Equation Modeling: Analyzing Latent and Emergent Variables, Chap. 2, The Guilford Press, London, New York, pp. 25-37.
Henseler, J., Hubona, G. and Ray, P.A. (2016), “Using PLS path modeling in new technology research: updated guidelines”, Industrial Management and Data Systems, Vol. 116 No. 1, pp. 2-20.
Henseler, J., Ringle, C.M. and Sinkovics, R.R. (2009), “The use of partial least squares path modeling in international marketing”, Advances in International Marketing, Vol. 20, pp. 277-320.
Henseler, J., Dijkstra, T.K., Sarstedt, M., Ringle, C.M., Diamantopoulos, A., Straub, D.W., Ketchen, D.J., Hair, J.F., Hult, G.T.M. and Calantone, R.J. (2014), “Common beliefs and reality about PLS: comments on Rönkkö and Evermann (2013)”, Organizational Research Methods, Vol. 17 No. 2, pp. 182-209.
Hooley, G.J., Greenley, G.E., Cadogan, J.W. and Fahy, J. (2005), “The performance impact of marketing resources”, Journal of Business Research, Vol. 58 No. 1, pp. 18-27.
Huang, W. (2013), “PLSe: efficient estimators and tests for partial least square”, PhD Thesis, University of California.
Hui, B.S. and Wold, H.O.A. (1982), “Consistency and consistency at large of partial least squares estimates”, in Jöreskog, K.G. and Wold, H.O.A. (Eds), Systems under Indirect Observation: Causality, Structure, Prediction Part II, North-Holland, Amsterdam, pp. 119-130.
Hulland, J. (1999), “Use of partial least squares (PLS) in strategic management research: a review of four recent studies”, Strategic Management Journal, Vol. 20 No. 2, pp. 195-204.
Hwang, H. and Takane, Y. (2004), “Generalized structured component analysis”, Psychometrika, Vol. 69 No. 1, pp. 81-99.
Jöreskog, K. (1969), “A general approach to confirmatory maximum likelihood factor analysis”, Psychometrika, Vol. 34 No. 2, pp. 183-202.
Jöreskog, K.G. (1970), “A general method for estimating a linear structural equation system”, ETS Research Bulletin Series, Vol. 1970 No. 2, pp. 1-41.
Jung, K., Takane, Y., Hwang, H. and Woodward, T.S. (2012), “Dynamic GSCA (generalized structured component analysis) with applications to the analysis of effective connectivity in functional neuroimaging data”, Psychometrika, Vol. 77 No. 4, pp. 827-848.
Jung, K., Takane, Y., Hwang, H. and Woodward, T.S. (2016), “Multilevel dynamic generalized structured component analysis for brain connectivity analysis in functional neuroimaging data”, Psychometrika, Vol. 81 No. 2, pp. 565-581.
Jung, S. and Park, J. (2018), “Consistent partial least squares path modeling via regularization”, Frontiers in Psychology, Vol. 9.
Kettenring, J.R. (1971), “Canonical analysis of several sets of variables”, Biometrika, Vol. 58 No. 3, pp. 433-451.
Klein, A. and Moosbrugger, H. (2000), “Maximum likelihood estimation of latent interaction effects with the LMS method”, Psychometrika, Vol. 65 No. 4, pp. 457-474.
Kline, R.B. (2015), Principles and Practice of Structural Equation Modeling, 4th ed., Guilford Press, New York, London.
Lohmöller, J.-B. (1989), Latent Variable Path Modeling with Partial Least Squares, Physica, Heidelberg.
Lowry, P.B. and Gaskin, J. (2014), “Partial least squares (PLS) structural equation modeling (SEM) for building and testing behavioral causal theory: when to choose it and how to use it”, IEEE Transactions on Professional Communication, Vol. 57 No. 2, pp. 123-146.
Lyotard, J.-F. (1984), “The postmodern condition: a report on knowledge”, Manchester University Press.
Mosteller, F. and Tukey, J.W. (1987), “Data analysis, including statistics”, in Jones, L.V. (Ed.), The Collected Works of John W. Tukey: Philosophy and Principles of Data Analysis 1965–1986, Wadsworth & Brooks/Cole, Monterey, CA, pp. 601-720.
Müller, T., Schuberth, F. and Henseler, J. (2018), “PLS path modeling – a confirmatory approach to study tourism technology and tourist behavior”, Journal of Hospitality and Tourism Technology, Vol. 9 No. 3, pp. 249-266.
Murphy, K.R. and Shiarella, A.H. (1997), “Implications of the multidimensional nature of job performance for the validity of selection tests: multivariate frameworks for studying test validity”, Personnel Psychology, Vol. 50 No. 4, pp. 823-854.
Nelson, H.G. and Stolterman, E. (2012), The Design Way: Intentional Change in an Unpredictable World, 2nd ed., The MIT Press, Cambridge, MA.
Peng, D.X. and Lai, F. (2012), “Using partial least squares in operations management research: a practical guideline and summary of past research”, Journal of Operations Management, Vol. 30 No. 6, pp. 467-480.
Rademaker, M.E., Schuberth, F. and Dijkstra, T.K. (2019), “Measurement error correlation within blocks of indicators in consistent partial least squares”, Internet Research, Vol. 29 No. 3, pp. 448-463.
Reinartz, W., Haenlein, M. and Henseler, J. (2009), “An empirical comparison of the efficacy of covariance-based and variance-based SEM”, International Journal of Research in Marketing, Vol. 26 No. 4, pp. 332-344.
Rhemtulla, M., van Bork, R. and Borsboom, D. (2020), “Worse than measurement error: consequences of inappropriate latent variable measurement models”, Psychological Methods, Vol. 25 No. 1, pp. 30-45.
Ringle, C.M., Sarstedt, M. and Straub, D.W. (2012), “Editor’s comments: a critical look at the use of PLS-SEM in MIS Quarterly”, MIS Quarterly, Vol. 36 No. 1, pp. 3-14.
Romdhani, H., Hwang, H., Paradis, G., Roy-Gagnon, M.-H. and Labbe, A. (2014), “Pathway-based association study of multiple candidate genes and multiple traits using structural equation models”, Genetic Epidemiology, Vol. 39 No. 2, pp. 101-113.
Rönkkö, M. and Evermann, J. (2013), “A critical examination of common beliefs about partial least squares path modeling”, Organizational Research Methods, Vol. 16 No. 3, pp. 425-448.
Rönkkö, M., McIntosh, C.N., Antonakis, J. and Edwards, J.R. (2016), “Partial least squares path modeling: time for some serious second thoughts”, Journal of Operations Management, Vols 47/48 No. 1, pp. 9-27.
Sarstedt, M., Ringle, C.M., Smith, D., Reams, R. and Hair, J.F. (2014), “Partial least squares structural equation modeling (PLS-SEM): a useful tool for family business researchers”, Journal of Family Business Strategy, Vol. 5 No. 1, pp. 105-115.
Sarstedt, M., Hair, J.F., Ringle, C.M., Thiele, K.O. and Gudergan, S.P. (2016), “Estimation issue with PLS and CBSEM: where the bias lies!”, Journal of Business Research, Vol. 69 No. 10, pp. 3998-4010.
Schamberger, T., Schuberth, F., Henseler, J. and Dijkstra, T.K. (2020), “Robust partial least squares path modeling”, Behaviormetrika, Vol. 47 No. 1, pp. 307-334.
Schuberth, F. (2021), “Confirmatory composite analysis using partial least squares: setting the record straight”, Review of Managerial Science, Vol. 15 No. 5, pp. 1311-1345.
Schuberth, F., Henseler, J. and Dijkstra, T.K. (2018a), “Confirmatory composite analysis”, Frontiers in Psychology, Vol. 9, p. 2541.
Schuberth, F., Henseler, J. and Dijkstra, T.K. (2018b), “Partial least squares path modeling using ordinal categorical indicators”, Quality and Quantity, Vol. 52 No. 1, pp. 9-35.
Schuberth, F., Rademaker, M.E. and Henseler, J. (2022), “Assessing the overall fit of composite models estimated by partial least squares path modeling”, European Journal of Marketing, in print.
Schwandt, T.A. (2007), The Sage Dictionary of Qualitative Inquiry, 3rd ed., Sage, Thousand Oaks, CA.
Sosik, J., Kahai, S. and Piovoso, M. (2009), “Silver bullet or voodoo statistics? A primer for using the partial least squares data analytic technique in group and organization research”, Group and Organization Management, Vol. 34 No. 1, pp. 5-36.
Spearman, C. (1904a), “‘General intelligence’, objectively determined and measured”, The American Journal of Psychology, Vol. 15 No. 2, pp. 201-292.
Spearman, C. (1904b), “The proof and measurement of association between two things”, The American Journal of Psychology, Vol. 15 No. 1, pp. 72-101.
Tenenhaus, M., Vinzi, V.E., Chatelin, Y.-M. and Lauro, C. (2005), “PLS path modeling”, Computational Statistics and Data Analysis, Vol. 48 No. 1, pp. 159-205.
Wall, M.M. and Amemiya, Y. (2000), “Estimation for polynomial structural equation models”, Journal of the American Statistical Association, Vol. 95 No. 451, pp. 929-940.
Wold, H.O.A. (1966), “Estimation of principal components and related models by iterative least squares”, in Krishnaiah, P. (Ed.), Multivariate Analysis, Academic Press, New York, NY, pp. 391-420.
Wold, H.O.A. (1974), “Causal flows with latent variables: partings of the ways in the light of NIPALS modelling”, European Economic Review, Vol. 5 No. 1, pp. 67-86.
Wold, H.O.A. (1975), “Path models with latent variables: the NIPALS approach”, in Blalock, H., Aganbegian, A., Borodkin, F., Boudon, R. and Capecchi, V. (Eds), Quantitative Sociology”, International Perspectives on Mathematical and Statistical Modeling, chap. 11, Academic Press, New York, pp. 307-357.
Wold, H.O.A. (1982), “Soft modeling: the basic design and some extensions”, in Jöreskog, K.G. and Wold, H.O.A. (Eds), Systems under Indirect Observation: Causality – Structure – Prediction Part II, chap. 1, North-Holland, Amsterdam, pp. 1-54.
Yu, X., Zaza, I., Schuberth, F. and Henseler, J. (2021), “Representing forged concepts as emergent variables using composite-based structural equation modeling”, ACM SIGMIS Database: the DATABASE for Advances in Information Systems, Vol. 52 No. SI, pp. 114-130.
Yuan, K.-H., Wen, Y. and Tang, J. (2020), “Regression analysis with latent variables by partial least squares and four other composite scores: consistency, bias and correction”, Structural Equation Modeling: A Multidisciplinary Journal, Vol. 27 No. 3, pp. 333-350.
Zhang, M.F., Dawson, J.F. and Kline, R.B. (2021), “Evaluating the use of covariance-based structural equation modelling with reflective measurement in organizational and management research: a review and recommendations for best practice”, British Journal of Management, Vol. 32 No. 2, pp. 257-272.
Acknowledgements
The first author served as a reviewer of Cadogan and Lee (2022). Additionally, the first author gratefully acknowledges financial support from FCT Fundação para a Ciência e a Tecnologia (Portugal), national funding through a research grant from the Information Management Research Center – MagIC/NOVA IMS (UIDB/04152/2020). The first author also acknowledges a financial interest in the composite-based SEM software ADANCO and its distributor, Composite Modeling. Both authors contributed equally and are listed in alphabetical order. Open access was made available through a deal between Emerald and the Association of Universities in the Netherlands (VSNU).
Erratum: The publisher of European Journal of Marketing wishes to inform readers that the article “Partial least squares as a tool for scientific inquiry: comments on Cadogan and Lee”, by Jörg Henseler and Florian Schuberth (2022), DOI: 10.1108/EJM-06-2021-0416 should have included an acknowledgement that as a comment the article was not subject to double blind peer review. This error was introduced during the production process. The publisher sincerely apologises for this error and for any inconvenience caused.