Abstract
Purpose
This paper aims to examine how bank stress tests affect bank tax planning.
Design/methodology/approach
The study uses US bank stress test bank size thresholds and a regression discontinuity design to investigate the effect of the Dodd-Frank Act and the instituted bank stress tests on bank tax planning. We use different measures of tax planning, including bank-specific measures and measures of tax avoidance, tax aggressiveness, and effective tax planning from recent literature. Our regression discontinuity and difference-in-differences regression analyses include bank and year fixed-effects and lagged bank characteristics to control for potential endogeneity.
Findings
This study finds that stress tests have the unintended consequences of intensifying tax planning and increasing tax avoidance. Stress-test banks increase tax avoidance by accelerating charge-offs, net interest, and non-interest expenses. However, this increase in tax planning is not optimally maximized, leading to lower effective tax planning compared to non-stress-test banks. Banks with a substantial increase in tax avoidance under the Dodd–Frank Act tend to increase their risk, investing in high-risk-weight assets and lending in riskier loan categories. These findings are consistent with tax minimization conditions under added regulatory attention and policy uncertainty.
Originality/value
Literature on bank tax planning is limited. Most tax avoidance literature excludes financial institutions such as bank holding companies mainly due to differences in business practices and regulatory frameworks. This study is the first to investigate tax planning behavior among US banks. The current study thus extends the research field by examining the effect of bank transparency regulations, such as bank stress tests, on bank tax planning activities. Our findings have a direct bank policy implication. They show that stress testing has the unintended consequences of increasing tax planning activities and consequently increasing risk-taking on banks with high tax avoidance, which goes against the goals of stress testing regulations.
Keywords
Citation
Francis, B.B., García, R.E. and Harithsa, J.G. (2024), "Taxes under stress: bank stress tests and corporate tax planning", China Accounting and Finance Review, Vol. ahead-of-print No. ahead-of-print. https://doi.org/10.1108/CAFR-03-2024-0035
Publisher
:Emerald Publishing Limited
Copyright © 2024, Bill B. Francis, Raffi E. García and Jyothsna G. Harithsa
License
Published in China Accounting and Finance Review. Published by Emerald Publishing Limited. This article is published under the Creative Commons Attribution (CC BY 4.0) licence. Anyone may reproduce, distribute, translate and create derivative works of this article (for both commercial and non-commercial purposes), subject to full attribution to the original publication and authors. The full terms of this licence may be seen at http://creativecommons.org/licences/by/4.0/legalcode
1. Introduction
Banks are important intermediaries in corporate tax planning (Gallemore, Gipper, & Maydew, 2019; Cheng, Francis, Li, Shen, & Wu, 2023) [1]. However, despite playing an important intermediary role, not much is known about banks’ own tax planning activities [2]. This lack of evidence is particularly acute given the recent far-reaching regulatory changes brought about by the Dodd–Frank Wall Street Reform and Consumer Protection Act of 2010 (Dodd–Frank Act or DFA), which have important implications for bank holding companies’ (BHCs) tax planning activities. In this paper, we take advantage of the implementation of the DFA to provide evidence on banks’ tax planning activities. This analysis focuses on the extent to which the Comprehensive Capital Analysis Review (CCAR) stress tests, under the DFA’s purview, encourage tax planning in the form of tax avoidance and tax aggressiveness. Evidence of tax planning is important on two accounts. First, it would suggest that bank stress tests also have the unintended consequence of increasing banks’ tax planning. Second, it would hurt the primary source of revenue (taxes) used to bail out banks during the 2008 Financial Crisis, essentially biting the hands that fed them.
Tax avoidance constitutes a legal activity through which a firm explicitly tries to reduce or defer its income tax liabilities [3]. We conjecture that CCAR stress testing, with the primary goal of ensuring that banks are well-capitalized to withstand adverse economic shocks, could result in tax avoidance through the mechanism of increased bank-level regulatory and tax-related uncertainty. For example, Ahnert, Vogt, Vonhoff, and Weigert (2020) find that there is a substantial drop in equity prices and a widening of credit default swap (CDS) spreads for failing banks on the announcement date of stress tests, magnifying bank-level regulatory uncertainty for stress-test banks relative to non-stress-test banks, which is likely to increase tax avoidance activities [4].
We contend that the overhaul of the financial sector through the DFA increases bank regulatory uncertainty generated by its stress-test and non-stress-test-related features. For instance, the DFA created non-stress-test-related broad measures and standards to regulate financial firms’ risk management, resolution plans, credit exposure reporting, executive compensation and reward, among others, impacting financial firms’ public perception and political discord [5]. For instance, the DFA created new regulatory oversight bodies, such as the Consumer Financial Protection Bureau and the Stability Oversight Council. Importantly, some of the new DFA changes generated increased tax uncertainty with the inclusion and the subsequent pullback of new reporting rules that would impact the tax bill of banks. One example is Title VII of the DFA, which mandated stricter rules, including the mark-to-market system on derivative contracts (Miller & Ann Ruane, 2012).
In this paper, we focus on regulatory uncertainty and use CCAR bank stress tests as identification. CCAR stress tests’ features and changes increase regulatory uncertainty for several reasons. First, stress tests increase regulatory and investor attention for treated banks that must comply with additional forward-looking transparency and new capital requirements, thus increasing a bank’s operational and revenue uncertainty (Kupiec, 2020). Second, stress-test-related policy changes have also occurred since the CCAR was first implemented, adding uncertainty and increased political discord. For instance, the threshold for stress-test banks has moved several times since 2011, from $100B in 2011–2012 to $50B in 2013–2016, then increased to $250B for 2017–2019 and moved back to $50B in 2020 due to the COVID-19 pandemic. Lastly, yearly changes to regulatory stress-testing scenarios and parameters can escalate the pressure on banks to meet the stipulated capital requirements and ratios.
Banks failing stress tests are likely to trigger reactions from not only policymakers but also investors since the results are made public, thus increasing bank performance uncertainty (Ahnert et al., 2020; Kupiec, 2020). Consequently, under higher regulatory uncertainty, we argue that banks have higher incentives to avoid taxes for several reasons. First, the cash saved from tax planning could be utilized to maintain capital buffers in compliance with stress tests. Failing stress tests could, apart from reputation concerns, trigger liquidity constraints (Goldstein & Sapra, 2014), which banks will look to avoid. Second, banks would desire to lower their performance uncertainty – thus, they might accelerate their net charge-offs (that is, write off bad loans) to signal fiscal stability and less riskiness. Employing bank-specific tax planning activities such as writing off bad debt and accelerating general expenses can help banks facing regulatory attention lower their risk. For instance, aside from lowering their risk under DFA-mandated transparency requirements, banks can take advantage of tax shields and tax credits from charge-offs and interest expenses.
Although we argue that increased regulatory uncertainty leads to a rise in tax planning, it is also possible that the forward-looking transparency requirements and regulatory attention created by the DFA could lead to less tax planning (Kerr, 2019). For example, Hanlon and Slemrod (2009) provide evidence that firms face investor and taxpayer backlash in the form of decreased stock prices in reaction to tax planning. Concerns about reputation and penalties from policymakers may also prevent firms from engaging in tax planning activities (Bankman, 2004; Chen, Chen, Cheng, & Shevlin, 2010; Graham, Hanlon, Shevlin, & Shroff, 2014; Mansi, Qi, & Shi, 2020). Voluntary disclosures have a strong disciplining role in firm tax avoidance activities (Boubaker, Derouiche, & Nguyen, 2021) – the same could be true for regulations such as stress tests that require mandatory disclosures. Therefore, ex ante, it is unclear whether bank stress testing would result in more or less tax planning in stress-test banks relative to non-stress-test banks. Hence, the net impact of stress testing on taxes paid is an empirical issue.
Notwithstanding the fact that CCAR stress tests do not explicitly regulate the tax planning activities of banks, stress testing creates changes in bank portfolio selection behavior (García & Steele, 2022), which directly affect bank taxes. Tax planning is one capital-preserving channel through which banks can maintain capital reserve requirements or save for future tax liability. Despite the added consultant fees, reporting costs and general reputation costs associated with tax planning, we contend that banks prefer tax planning activities to other capital-preserving channels (such as bank relationship lending, added collateral, amendments to covenant agreements and decreases in labor, among others), as they are less likely to affect bank operations (Edwards, Schwab, & Shevlin, 2016; Nguyen & Nguyen, 2020) [6]. We add to the literature by investigating whether stress-testing of banks – and the regulatory uncertainty created by them – exacerbate bank tax planning.
To test our conjecture that bank stress tests impact tax planning, we follow Gallemore et al. (2019) by focusing on tax avoidance and tax aggressiveness. We construct our tax planning measures using the cash and GAAP effective tax rates (Robinson, Sikes, & Weaver, 2010; Gawehn, 2020). We create our tax aggressiveness index (TAI) by following Balakrishnan, Blouin, and Guay (2019), who benchmark firms relative to their peers according to asset size and year. We implement a regression discontinuity design (RDD) by exploiting the policy thresholds mandated by the CCAR stress testing regime under the DFA for 2011–2016, which gives us a quasi-experimental setting to test our hypotheses. Under CCAR, banks with assets of at least $100B for 2011–2012 and with at least $50B for 2013–2016 were required to be stress tested. These banks form our treated group, while banks right below the corresponding policy threshold for the given year form our control group.
We find that supervisory bank stress tests have the unintended consequences of increasing treated banks’ tax avoidance and aggressive tax planning activities relative to control banks. Importantly, our results are also economically meaningful. For instance, the Cash ETR decreases (tax avoidance increases) between 0.2% and 2.4% points for the annual measure and 0.2% points when we use a 3-year rolling average. We find similar results when we use tax aggressiveness as our tax planning measure. Specifically, we find an increase of 0.2% points at the annual level and an increase of 1.0% points for the 3-year Tax Aggressiveness Index or TAI rolling average, indicating that stress-test banks tend to be more tax aggressive than the non-stress-test banks, consistent with our Cash ETR findings. We also use the annual and the 3-year rolling average for the GAAP effective tax rate (GAAP ETR) as another measure of tax avoidance (Dyreng, Hanlon, & Maydew, 2008) and find consistent results.
Additionally, we examine bank-specific tax planning activities. Banks are very complex and different from non-financial firms, so we rely on two additional measures, charge-offs and the acceleration of the general expenses. Taking advantage of tax shields by writing off bad loans is a unique tool only loan-granting institutions possess. Stress tests could increase bank-specific tax planning under DFA by accelerating the recognition of bad debt through charge-offs and accelerating tax-deductible expenses, including net interest and non-interest expenses, both of which offer tax shield benefits. Using our RDD, we find an increase in net interest expenses. This is consistent with the argument that following the imposition of the CCAR, stress-test banks increase debt financing for which interest payments have tax treatment advantages.
Given that stress-test banks may have to invest more resources in tax planning activities under the added transparency requirement, this raises the question of how efficient treated banks are in tax planning under stress testing. To investigate this question, we use Schwab, Stomberg, and Williams (2022)’s Effective Tax Planning score, which measures the relative efficiency of turning resources invested in tax planning activities into higher after-tax returns. We find that stress-test banks are significantly less effective at tax planning than non-stress-test banks. This evidence suggests that although the regulatory attention and uncertainty that come with stress testing increase bank tax planning activities, treated banks are less efficient at tax planning than banks in the control group. This finding implies that stress-test banks are either over-investing in the inputs for tax planning or not optimizing their after-tax returns, given their current tax planning input levels.
Next, we test the mechanisms through which the bank regulatory and tax-level uncertainty impact banks’ tax planning behavior. We document that stress-test banks receive higher overall regulatory uncertainty and Internal Revenue Service (IRS) attention. We proxy for regulatory uncertainty using political risk and political tax risk measures (Hassan, Hollander, Van Lent, & Tahoun, 2019). We triangulate our results using the communicated bank portfolio uncertainty in their 10-K filings related to the composition and the set regulatory requirements of the bank’s portfolio (Owusu & Gupta, 2023; García, Harithsa, & Owusu, 2024). Finally, we corroborate our results using bank performance-related uncertainty, such as their earnings and cash flow volatilities (Allayannis & Weston, 2003). We proxy for IRS attention using measures developed by Bozanic, Hoopes, Thornock, and Williams (2017) using text analysis.
The testing of the channels through which stress tests impact banks’ tax planning activities proceeds in two stages. Using regression discontinuity, we first show that banks above the policy thresholds experience increased regulatory and tax-related uncertainty for stress-test banks relative to non-stress banks. This finding is consistent with the regulatory uncertainty sentiment, which seems to deteriorate using the annual measure. Next, we directly test using a difference-in-differences causal interaction approach (or triple differences) whether the increased uncertainty drives tax planning activity in stress-test banks. We then analyze the tax planning activities of banks with a significantly large increase in uncertainty post-CCAR compared to the pre-CCAR period. We find evidence suggesting that tax planning activities are prevalent in banks that face higher regulatory uncertainty, tax-related regulatory uncertainty and sentiment-related uncertainty. These findings support our argument that stress-testing of banks leads to an increase in bank-level regulatory and tax-related uncertainty, which results in higher tax avoidance [7].
Lastly, we analyze the implications of tax planning (particularly tax avoidance) on bank performance, capital ratios and lending, among other characteristics. Using banks that experienced a significant increase in tax avoidance under the DFA, we find no evidence that financially constrained or low-performing banks drive bank tax avoidance, further supporting our finding that bank-level regulatory uncertainty rather than bank performance is a key moderator for bank tax avoidance. We do, however, find that banks with increased tax avoidance under stress testing: (1) take on more risk, evidenced by the higher risk density and the transfer from lesser risk-weight assets to holding more risk-weight assets; (2) face an increased cost of equity; and (3) face increased legal consulting fees, suggesting that higher tax planning increases these costs. Taken together, these results show that under DFA, banks with the highest increase in tax avoidance post-CCAR took on more risk, thus conflicting with the risk-mitigating intention of stress testing.
Our baseline results withstand a series of robustness checks. First, we implement the standard McCrary manipulation test to ensure that banks in our sample do not violate local continuity by choosing to be below the threshold. Second, we test that our baseline tax avoidance results are not driven only by the initial policy threshold of $100B for 2011–2012. Third, we test for the stability of our baseline results under the inclusion and exclusion of covariates. Fourth, we implement pre-tests, employing the RDD for our banks in the pre-regulation period (2004–2010), to validate the CCAR stress tests as the significant driver of changes to tax avoidance behavior. Lastly, we conduct falsification tests, wherein we create false policy thresholds of ±10% of the actual policy thresholds, which show that the impact on tax avoidance at the threshold is due to the passage of the stress testing policy under the DFA.
We contribute to three strands of literature. First, we contribute to the sparse literature on tax avoidance and BHCs (Gallemore et al., 2019; Gawehn & Müller, 2020; Gawehn, 2020) and to the existing literature on tax avoidance and the costs and benefits it brings to the firm (Desai & Dharmapala, 2006, 2009; Hanlon & Slemrod, 2009; Rego & Wilson, 2012; Graham et al., 2014; Armstrong, Blouin, Jagolinzer, & Larcker, 2015; Goh, Lee, Lim, & Shevlin, 2016).
Second, we add to the literature that looks at how bank-level regulatory and tax-related uncertainty is impacted both in isolation (Hutchens & Rego, 2015; Hanlon, Maydew, & Saavedra, 2017) and in tangent (Gallemore & Labro, 2015; Drake, Lusch, & Stekelberg, 2019; Dyreng, Hanlon, & Maydew, 2019, 2021) with tax avoidance. We also contribute to an emerging strand of literature on uncertainty and textual analysis sentiments (Inger, Meckfessel, Zhou, & Fan, 2018; Hassan et al., 2019; Fernandes, Igan, & Pinheiro, 2020; Nguyen, 2020). Notably, we extend the literature on firm-level regulatory and political risk (Hassan et al., 2019), which uses textual analysis to analyze earnings call transcripts. Finally, we contribute to the growing macroprudential stress test and bank characteristics literature (Gambacorta & Shin, 2016; Bassett & Berrospide, 2017; Acharya, Berger, & Roman, 2018; García & Steele, 2022) by studying the impact of stress testing and tax avoidance.
The rest of this paper is laid out as follows. Section 2 provides background on the Dodd–Frank regulation. Section 3 presents a brief survey of tax avoidance literature, theoretical arguments and hypotheses development. Section 4 introduces our data sources and definitions of our variables. Section 5 describes the identification strategy employed. Section 6 discusses our findings, with further robustness checks provided in Section 7. Section 8 concludes.
2. DFA and stress testing institutional background
The objective of bank stress tests is to capture and remedy shortcomings in bank functioning, hence ensuring viability and long-term sustainability. Early stress tests were conducted internally by banks and were backward-looking till a more forward-looking approach to capture changing trends in banking was adopted in the early 1990s (Hirtle, Kovner, & Plosser, 2020). However, the banking industry’s role in exacerbating the Financial Crisis of 2007–2009 led to the passage of the DFA of 2010, which included provisions for macroprudential stress testing and oversight of the banking sector. The DFA implemented the Supervisory Capital Assessment Program (SCAP), under which the Federal Reserve supervised stress tests for all banks with at least $100B in assets [8]. The SCAP evolved into the CCAR in 2011, which expanded in 2013 to include banks with at least $50B or more in total assets (Berrospide & Edge, 2019; Clark, Francis, Garcia, & Steele, 2019; García & Steele, 2022). In more recent years, the threshold for stress test banks has moved several times, increasing to $250B for 2017–2019 and back to $50B in 2020 due to the COVID-19 pandemic [9].
The Federal Reserve and other federal regulatory agencies involved in stress testing require BHCs to maintain minimum capital requirements [10]. In addition to maintaining these capital ratio requirements, large banks above the corresponding policy thresholds are required to comply with stress testing their balance sheet according to the given adverse economic scenarios. These include distress to economic variables such as the gross domestic product, housing prices or unemployment, among others (Berrospide & Edge, 2019; Hirtle et al., 2020; García & Steele, 2022). The stress tests’ adverse economic scenarios evolved to ensure that tail risk is accounted for and tested for adequate capital planning [11]. The stress test results are disclosed for transparency purposes, allowing the financial market to penalize those that fail and reward those that pass the tests in order to bolster public confidence in the banking system post-crisis (Kohn & Liang, 2019; Cornett, Minnick, Schorno, & Tehranian, 2020; Nguyen, Nguyen, & Sila, 2019).
In addition to implementing CCAR stress tests, the DFA created broader measures and standards affecting financial firms’ risk management, resolution plans, credit exposure reporting, executive compensation and reward and public perception. For instance, new regulatory bodies were created. The Consumer Financial Protection Bureau was created as an oversight committee to monitor financial institutions and protect consumers from fraudulence, unfair lending, mortgage and credit card practices. The Stability Oversight Council was designed to react to “emerging financial risks” and report policy recommendations to Congress such as alterations to the leverage and liquidity of financial firms. The added financial safeguards and regulatory, investor and public attention brought about increased uncertainty to financial firms, particularly BHCs.
The DFA also sought to reform the derivatives market (e.g. Title VII of The Dodd–Frank Act). According to the provisions in Title VII, swaps would be exchanged centrally through a clearinghouse rather than over-the-counter and consequently have an imposed margin requirement. Additionally, contracts would follow a mark-to-market regime of the Internal Review Code 1256. That is, banks and insurance firms that employ derivatives for risk management would face adverse tax consequences as if the contract had been sold at the end of the year, whether sold or not – which would have immense implications for bank tax reporting and tax volatility. For instance, if interest rates shifted even slightly, a firm employing interest rate swaps would face large uncertainty in forecasting its income tax bill. Furthermore, the mark-to-market system can potentially increase a firm’s tax loss carry-forward. Section 1601 under Title XVI modified the ruling. It stated that a wide variety of derivatives (such as exchange rate swaps, interest rate swaps, credit default swaps and securities futures contracts, to name a few) were not to be included as a “section 1256 contract,” thus ensuring they faced no increased uncertainty surrounding their taxable incomes. Implementing Title VII and restoring the status quo with Title XVI likely created an atmosphere of tax-related uncertainties.
Our paper studies US-based bank regulations and their associated bank regulatory uncertainty, however, some international banking regulations may interact with US-based regulations. For example, liquidity ratio requirements (as part of a global framework instituted in response to the financial crisis, set forth by Basel III) were implemented in the USA in 2013 for all banks above $10B in assets, with more stringency imposed on large BHCs over $250B in assets. Several other provisions, such as rules for securitization and equity investments, were instituted starting in 2017. Several mandates under Basel III (such as supplemental leverage ratio) were instilled only for globally systemically important banks (G-SIBs) or for banks over $700B in assets. In the USA, the capital requirements set forth by the Collins Amendment prevail over those under Basel III (Paskelian & Bell, 2013). Consequently, to circumvent potential confounding international factors, our analysis covers the period from 2000 to 2016 and focuses on banks with total assets between $10B and $200B. Thus, we can attribute any changes to tax planning at our thresholds to the CCAR stress tests and not to changes implemented under Basel III.
3. Related literature and hypotheses development
Empirical research finds that tax planning can have a mixed impact on firm value. For example, some literature shows the positive firm value (Desai & Dharmapala, 2009; Wilson, 2009; Blaylock, 2016; Drake et al., 2019; Garrett & Suárez Serrato, 2019). However, shareholder’s tax planning benefits come with certain costs like increased cost of debt (Hasan, Hoi, Wu, & Zhang, 2014; Cheng et al., 2023), higher external audit fees (Donohoe & Robert Knechel, 2014), declining stock prices (Hanlon & Slemrod, 2009), firm value (Wahab & Holland, 2012), higher managerial rent extraction and reduced corporate transparency and information transparency (Desai & Dharmapala, 2006; Balakrishnan et al., 2019; Kerr, 2019; He, Ren, & Taffler, 2020; Allen, Francis, Wu, & Zhao, 2016). Recent work by Tang (2019) shows that, on average, the benefits of increased tax savings are likely to far outweigh the costs.
Literature on bank tax planning is limited. Most tax avoidance literature excludes financial institutions such as BHCs mainly due to differences in business practices and regulatory frameworks. In a concurrent study, Gawehn and Müller (2020) compare the level of tax avoidance in banks relative to non-banks and find that banks have higher Cash ETR (and hence, less tax avoidance) than non-bank firms. In a review of the limited literature, Gawehn (2020) provides several reasons why banks might try to keep their tax expenses low, such as lower profits from lending and deposits due to low interest rates and automation, compliance with mandated capital ratios and shareholder expectations. Interestingly, while potential bank clients prefer banks that are tax-intermediary compared to banks that do not perform tax-intermediary activities in addition to their traditional financial intermediary roles (Gallemore et al., 2019), banks perceive tax avoidance by their clients negatively, which results in the imposition of higher loan costs and spreads for firms that display aggressive tax planning (Hasan et al., 2014).
While these studies examine the impact of tax avoidance relative to non-bank firms or on bank customers, they do not study the phenomenon of tax avoidance within banks. One exception in the literature is a concurrent study by Jin, Liu, Zhang, and Zhao (2022), who find that financially constrained banks are more likely to tax avoid but are less likely to do so following the passage of the DFA [12]. We extend and contribute to the sparse literature by examining changes in tax planning by banks as a result of exogenously imposed bank stress testing and the related regulatory uncertainty within a quasi-experimental setting. Thus, we propose two competing arguments.
First, we conjecture that bank stress tests lead to tax planning by increasing regulatory and bank-level uncertainty. While the overall goal of regulation is welfare maximization, satisfying the requirements of the regulation is often burdensome and can lead to higher costs (Kalmenovitz, 2019). Recent literature on policy uncertainty, which includes uncertainty about regulation, tax and government spending, among others, shows that uncertainty affects firm-level behavior (Baker, Bloom, & Davis, 2016; Gulen & Ion, 2016; Nguyen & Nguyen, 2020). Regarding the tax literature, Hanlon et al. (2017) show that firms with higher tax uncertainty increase cash savings for future requirements. In our setting, the extant literature shows evidence of increased informational uncertainty brought on by the CCAR stress tests, which is indicative of imprecision in the news perceived by investors (Fernandes et al., 2020) [13]. Stress testing impacts the regulatory capital of the banks and their risk-taking activities. Hence, stress testing puts additional financial uncertainty on banks. The added pressures from investors and regulators, together with the different Dodd–Frank tax-related and stress test policy changes, contribute to bank-level regulatory uncertainty. Therefore, banks might look to offset potentially related losses by increasing their cash savings via tax planning activities. We argue that banks are likely to engage in tax avoidance activities as opposed to traditional cost-minimizing methods such as cutting labor costs, capital expenditure, sales and advertising-related costs, among other expenses, because doing so is less likely to affect bank operations negatively (Edwards et al., 2016; Nguyen & Nguyen, 2020). We, therefore, predict the following:
Bank stress tests increase bank regulatory uncertainty, which increases tax planning activities.
However, it is also possible that transparency requirements and regulatory attention may lead to less tax avoidance since it would be easier to detect red flags (Kerr, 2019; He et al., 2020). Additionally, forward-looking transparency requirements such as bank stress tests may set higher capital requirements and loan loss reserves, negatively affecting taxable income. Consequently, augmenting the probability or uncertainty of receiving an IRS penalty serves as a disincentive for tax avoidance and aggressive tax planning. Hence, we hypothesize that:
Bank stress tests increase bank regulatory uncertainty, which decreases tax planning activities.
Therefore, ex ante, it is unclear whether bank stress testing would result in more or less tax avoidance in treated banks relative to control banks. Hence, the net impact of stress testing on taxes paid is an empirical question.
3.1 Bank tax planning activities
Gawehn (2020) contend that corporate taxation can influence bank behavior through multiple dimensions – organization form choices, tax incidence, debt financing, profit shifting, financial reporting transparency and customer tax avoidance. We conjecture that under regulatory attention and bank stress testing, banks engage in tax planning activities that do not significantly alter their bank operations. We focus on three broad forms of bank tax planning activities: (1) management of loan loss reserves, loan loss provisions and charge-offs; (2) management of debt financing and interest payments for tax shield benefits; and (3) acceleration of tax-deductible expenses.
First, loan loss reserve or loan loss allowance is a bank’s contra-asset set aside for potential bad loans or losses. Each quarter, the loan loss allowance rises by the amount of the loan loss provision (an expense item). The loan loss allowance is reduced by the level of the net charge-offs (total charge-offs minus total loan recovery). The net charge-offs can also be added to the loan loss reserves for net recoveries. In the USA, the net charge-offs are used for tax deduction purposes based on when a loan loss is first recognized [14]. Therefore, US banks can only receive tax shield benefits on net charge-offs but not on loan loss allowance or provisions. An increase in loan loss allowance does not necessarily increase charge-offs. However, it increases expenses and reduces capital without resulting in a corresponding tax deduction. Andries, Gallemore, and Jacob (2017) find that in countries where loan loss provisions are tax-deductible, a 1% increase in corporate tax rates increases loan loss provisions by approximately 5%. Therefore, we predict that US stress-test banks would recognize or write off more bad loans earlier. The benefits to stress-test banks for doing this are two-fold: it helps remove risky loans from their balance sheets while obtaining a tax shield for the increase in charge-offs. Hence, we contend that:
Charge-offs – Stress-test banks increase tax planning relative to non-stress-test banks by increasing charge-offs.
Another noteworthy characteristic of bank financial accounting is the tax treatment of debt financing. The literature has established that the preferential tax treatment of debt financing (the interest payments are tax-deductible) versus equity financing encourages banks to become more leveraged (Schepens, 2016; Andries et al., 2017), which goes against the objective of stress testing. Hence, we conjecture that:
Debt Financing and Interest Payments – Stress-test banks increase tax planning via increasing debt financing and interest payments.
According to practitioners and tax consultants, banks can use a variety of tax deductions without drastically affecting their operations under bank stress testing to increase their tax benefits [15]. These tax deduction activities include the acceleration of tax deductions for qualifying prepaid expenses by deducting certain short-term prepaid items the quarter they were paid; increasing depreciation and expensing of qualified fixed assets through bonus depreciation and Section 179 expenses; paying compensation and related incentives early or amending related agreements to fix accruals at year-end; and the accelerating charitable contributions and donations. In terms of managing their portfolio, Foster (2017) argues that banks can also obtain tax deduction benefits by selling loans or securities with losses; deferring the sale of loans or securities with gains; acquiring and holding tax-exempt securities such as municipal bonds; selling other real estate owned property with post-foreclosure write-downs; performing a cost segregation study on new and existing buildings; and deducting loan origination costs in the year paid. Therefore, we predict that:
Tax-Deductible Expenses and Tax-Exempt Securities – stress-test banks increase the acceleration of bank tax-deductible expenses and acquisition of tax-exempt securities (such as municipal bonds).
4. Data and variable definitions
In our analysis, we use the universe of US BHCs with at least $10B in total assets [16], giving us an average of 97 BHCs – of which 35 are large BHCs with at least $50B in assets that form our treated group of banks, and the rest are regional banks with $10B–$50B in total assets that form our control group. We focus our study on the sample period from 2000 to 2016, we stop at 2016 for several reasons. First, we want to capture the immediate effects of the first few years of bank stress testing using the different policy thresholds of $100B for years 2011–2012 and the $50B for 2013–2016, Second, by stopping at 2016, we avoid confounding effects due to new Basel III revised rules targeting capital and risk-weighted assets requirements that took effect on 2017, as described in Section 2. Third, there were new stress test threshold changes that were implemented after 2016. The CCAR stress-test threshold was increased to $100 in 2017 (for the year 2018) and to $250B in 2018 (starting in 2019) with the passage of the EGRRCPA, which meant that only a select few of the largest banks would be stress-tested. This threshold was reverted to $50B in 2020 due to the COVID-19 pandemic. Thus, as in García et al. (2024), we focus on the 2000–2016 years to focus on the immediate effects of the policy, to avoid potential compounding effects and issues related to constant policy changes and rollbacks.
We collect data on bank characteristics for 2000–2016 from the Bank Regulatory Database available through Compustat, which includes the FR Y-9C Consolidated Statements for Financial Companies data report generated by the Federal Reserve. We use the data on bank characteristics to create our different bank tax planning measures and to select the set of controls in our analysis. To investigate how efficient banks are at tax planning under added regulation, we use the effective tax planning measure from Schwab et al. (2022), who created the measure using data envelopment analysis based on the Scholes, Wilson, and Wolfson (1992) paradigm of effective tax planning [17].
We use the publicly available firm-level political risk dataset provided by Hassan et al. (2019) to proxy for bank regulatory uncertainty and attention [18]. The authors use textual analysis to develop firm-level measures for different types of political risks faced by public firms in the USA; we employ the political risk, the political risk sentiment and the political tax risk in our paper. To measure bank portfolio uncertainty, as perceived and communicated by banks, we employ bank portfolio uncertainty sentiment data (Owusu & Gupta, 2023; García et al., 2024). Our data comes from Owusu and Gupta (2023), who create bank risk culture sentiment measures using natural language processing on the unstructured text in 10-K filings to extract the risk culture features associated with different risk culture drivers and sentiments. We focus on bank portfolio uncertainty since one of the key purposes of the stress tests is the disclosure of portfolio and balance sheet information to regulators.
We use the future expectations of earnings and cash flow volatility as measures for bank performance uncertainty. Stable cash flows and earnings signal less of a need for firms to rely on external financing to investors, managers and analysts (Minton & Schrand, 1999) and reduce the firm’s borrowing costs (Trueman & Titman, 1988). Thus, according to corporate risk management theory, the volatility of these measures is considered to be indicative of high uncertainty in the firm, which is directly linked to a loss in firm value (Allayannis & Weston, 2003). We employ the standard deviation of the diluted and the basic earnings per share, excluding extraordinary items as our measures for earnings volatility, and the standard deviation of the cash flow scaled by number of shares and cash flow scaled by total assets for two measures of cash flow volatility.
We employ the cash effective tax rate (Cash ETR), defined as the cash taxes paid scaled by pre-tax income net of special items, as our primary measure of tax avoidance, and the GAAP ETR (Dyreng et al., 2008; Hanlon & Slemrod, 2009) as our secondary measure [19]. Although the usage of effective tax rates is more prevalent in the literature pertaining to non-financial firms, it is a relatively straightforward measure that can be calculated for banks as well – banks keep track of the annual cash paid in taxes as well as the pre-tax income. For example, Gawehn and Müller (2020) recognized and used the ETRs to compare banks and non-banks. Given that our analysis only compares banks to other banks, we justify our usage of ETRs as a sound measure of tax avoidance. The Cash ETR is more robust to accrual manipulation of the two measures and accounts for some tax-related benefits that are not recorded on the income statement (Cheng, Huang, Li, & Stanfield, 2012) [20]. We eliminate all observations with negative pre-tax income and winsorize our variables at 1% and 99% levels.
Tax aggressiveness (comprising activities like tax shelter, for example), according to Hanlon and Heitzman (2010), is at the more extreme end of the tax avoidance continuum. In contrast, explicitly legal tax avoidance activities, such as firm investments in municipal bond instruments, are considered non-aggressive [21]. Hence, a low Cash ETR obtained through the purchase of risk-free instruments such as municipal bonds does not qualify as tax aggressiveness. Balakrishnan et al. (2019) create a measure of tax aggressiveness that benchmarks a firm’s tax avoidance compared to other firms; a firm is considered tax aggressive if its tax planning activities are unusual when compared to industry peers in the same year. We create our bank-year controlled measure of tax aggressiveness by following Balakrishnan et al. (2019). Additionally, we generate three-year rolling average tax avoidance (and tax aggressiveness) variables as longer-run measures are considered to be more stable (Dyreng et al., 2008) and signify sustained tax planning practices over a period extending beyond the passage of the regulation, thus indicating the propensity of banks to manage their taxes over the longer run compared to the annual measures of the same. A negative sign on Cash ETR coefficient indicates an increase in tax avoidance, which is the expected sign consistent with our hypothesis (wherein we conjecture that stress tests lead to an increase in tax avoidance, which is a decrease in cash paid in taxes). Our hypothesis presumes a positive sign on the TAI.
Knowing that banks are complex and differ from non-financial firms because banks have fewer tax shelters, we use different measures of tax planning other than ETRs to triangulate our results. Banks are unique in that, as loan-granting institutions, they can exploit tax shields from interest expenses and net charge-offs. We thus employ these bank-specific tax planning measures as well. The Schwab et al. (2022)’s effective tax measure captures Scholes et al. (1992)’s theoretical framework, which defines effective tax planning as the required consideration of both explicit and implicit taxes, together with the non-tax costs of implementing tax planning strategies. By definition, the effective tax planning measure differs from our tax avoidance measures such as Cash ETR because it includes an optimization condition of maximizing after-tax returns given current inputs such as research and development (R&D) expenditure, gross property, plant and equipment (PP&E), tax haven operations, intangible assets, inventory and leverage [22]. The effective tax planning score ranges from 0 to 1, with one showing the highest efficiency possible. The measure is relative to the efficient frontier, the best firm score within the industry-year among the corresponding peer group. According to Schwab et al. (2022), a score of 0.4 indicates that a bank is 40% as effective at tax planning as the best bank in their industry-year group. The bank could reach the efficient frontier by increasing its after-tax return by 150% or by achieving the same after-tax returns through a 60% decrease in inputs.
Given that we explore tax planning activities in banks in this paper, another interesting question is the impact of the regulation on the attention of the IRS. This is a pertinent question, given that regulatory requirements for private or public tax-related disclosure requirements can lead to changes in IRS attention and the way firms make changes to these disclosures (Bozanic et al., 2017). We employ the measure made available by Bozanic et al. (2017), who calculate IRS attention as the number of times financial documents pertaining to banks are downloaded from the SEC EDGAR database in a particular year, using an IP address that belongs to the IRS.
Table 1 provides more detailed variable construction and definitions. Table 2 presents the descriptive statistics of the tax planning measures (Panel A), the bank characteristics (Panel B) and the regulatory uncertainty and portfolio risk measures (Panel C) for the Dodd–Frank years of 2011–2016, for stress-test versus non-stress-test banks [23]. On average, banks in our dataset have a Cash ETR and GAAP ETR of 22.3% and 25.5%, respectively (See Panel A). According to Balakrishnan et al. (2019), the ETRs being lower than the corresponding statutory tax rate (35%) is consistent with tax planning. The Cash ETR (GAAP ETR) is 19.8% (24.8%) in stress-test banks versus 23.4% (25.8%) in non-stress-test banks, which is indicative of more tax avoidance on average in stress-test banks. Interestingly, stress-test banks’ tax aggressiveness is several percentage points higher than non-stress-test banks. The effective tax planning scores show the average bank to be around 50% as effective in tax planning as the best bank within their industry-year peer group, with stress-test banks having a slightly higher average relative to non-stress-banks. Stress-test banks receive almost five times the attention from the IRS as non-stress-test banks (49.58 yearly downloads on average versus 10.08). Panel B shows bank characteristics such as risk density (or risk-weighted assets), capital ratios, lending variables and balance sheet variables, among others. It shows that treated banks tend to have lower risk density (0.72) than non-stress-test banks (0.76) while also showing a net income roughly 24 times the average net income for the non-stress-test banks. Lastly, Panel C shows that stress-test banks, on average, have a higher regulatory uncertainty but lower regulatory uncertainty sentiment than non-stress-test banks.
5. Empirical strategy
We use a RDD to measure the effects of mandatory transparency disclosure requirements via stress tests on bank tax planning. We implement a sharp RDD approach given the set policy cut-off based on the total assets above which banks must comply with stress testing. As discussed in Section 2, this cutoff is $100B for 2011 and 2012 and $50B for 2013–2016. Given that large and regional banks tend to be on differential growth trends with respect to asset size and other key variables, our RDD allows us to capture the local average treatment effects of banks’ stress tests on their tax planning and thus directly compare banks that are very similar to each other but differentiated by the stress testing compliance requirements.
Our baseline model implements the following RDDs using linear and quadratic specifications, in Equations (1) and (2), respectively, to achieve functional form flexibility in which β1 is the average local treatment effect of interest:
Regarding the vector of fixed effects, δ, it includes bank and year fixed effects [25]. The fixed effects help us control for unobserved bank-level attributes that might affect tax planning (such as bank business practices, executive compensation and composition, executive ability, regulator-bank relationship and auditors, among others) and the year fixed effects help for variation in state and federal tax regulations, federal district regulatory attention and macroeconomics conditions that may impact the demand and supply of bank business services, among others. The inclusion of covariates and fixed-effects should ensure comparable treatment and control groups since the comparison is made within the same subject rather than between different subjects. Lastly, the interaction terms STit∗AssetNormit and
For robustness purposes, we provide the linear and quadratic RDD specifications [26]. We report the results for each local regression specification (linear and quadratic) by estimating the mean square error (MSE) optimal bandwidth by taking the minimum optimal bandwidth of the most common MSE-optimal procedures (Imbens & Kalyanaraman, 2012; Calonico, Cattaneo, & Titiunik, 2014; Ludwig & Miller, 2007) [27].
One of the drawbacks of using optimal bandwidths is that the data-driven bandwidth selection can result in widely varying bandwidth sizes according to the covariates or dependent variables included in the specification. Hence, we take two steps to ensure a similar number of observations across the different dependent variables in our study. First, we set our model requiring that the optimal bandwidth includes at least 65 observations, unless otherwise stated, ensuring we maintain a balance between stress test and non-stress-test banks for our main model specifications [28]. Second, to circumvent concerns regarding highly localized findings, we encompass a wider window of banks by specifying a bandwidth to include all banks between $10B and $200B in asset size (labeled as All Relevant Banks in our tables) [29].
Lastly, we conduct a series of robustness checks to ensure the soundness of our methodology and findings. In particular, we focus on ensuring that the bank stress test regulation is the key driver of the effects and that banks are not manipulating their size to fall under the threshold to evade the regulation and the added regulatory attention. To that end, we conduct running variable manipulation tests, balanced covariate and covariate sensitivity tests, pre-tests and falsification tests (see Section 7).
6. Results: bank tax planning under stress
We begin our empirical analyses by first analyzing bank behavior around CCAR thresholds of interest, allowing us to compare stress-test and non-stress-test banks for 2011–2016. Our quasi-experimental setting supports the RDD assumption that banks close to the policy thresholds possess similar characteristics, emphasizing that only the treatment of stress testing drives the behavioral effects. Figure 1 presents the discontinuity or the local average treatment effect for risk-weighted assets around the standardized policy cut-off in the top panel, and the Cash ETR at the bottom. The risk-weighted assets graph confirms the findings from recent literature showing that stress tests have the intended results in reducing the risk in the treated banks relative to the control group (Acharya et al., 2018; Cortés, Demyanyk, Li, Loutskina, & Strahan, 2020; Cornett et al., 2020; García & Steele, 2022; García et al., 2024). Our study’s novel contribution is that stress tests also have the unintended consequences of increasing tax planning, as evidenced by the lower Cash ETR (higher tax avoidance) for banks immediately above the policy threshold relative to those right below.
The above tax planning evidence is further supported by the results obtained using our primary empirical strategy as described in Section 5. Table 3 presents our baseline results using our RDD for the annual and three-year rolling average bank tax avoidance and tax aggressiveness measures – which we winsorized at 1% and 99% levels for the lower and the upper bound. We find that the local average treatment effect for cash taxes paid decreases between 0.66% and 2.39% points for banks under an optimal bandwidth and between 0.19% and 0.47% points for all relevant banks between $10B and $200B in asset size, these results are robust at 99% confidence level. The Cash ETR (our main variable of interest) shows both localized as well as broader effects for tax planning. This evidence implies that stress-test banks increase their level of tax avoidance relative to non-stress-test banks under the DFA. The magnitude of the effect is economically substantial. Given that the mean pre-tax income for banks in our sample is $581 million, a reduction in the Cash ETR of 0.66–2.39% points represents $3.84–$13.86 million in annual tax savings for an average bank. This relationship persists even when we employ a three-year rolling average for tax avoidance, including all relevant banks (a drop between 0.17% points). These findings are corroborated when we use the GAAP ETR as a measure of tax avoidance based on income tax expenses, which decreases between 0.67 and 1.4 (0.58–0.88% points) for the annual (three-year moving average) measure. However, upon including all relevant banks by relaxing the optimal bandwidth condition, we find no significance for GAAP ETR. We do not see the localized effects persisting for a broader sample of banks for either the GAAP ETR or the TAI, suggesting that the regulation itself could have localized effects rather than broader effects [30]. Importantly, we also find evidence that stress-test banks increase aggressive tax planning (0.2–1.0% points) due to the implementation of stress tests [31]. Our overall results show localized effects for bank tax planning as well as broader effects [32].
6.1 Bank tax planning activities
Given the evidence discussed above, an interesting question is, how do banks conduct tax planning or tax avoidance under stress testing? We hypothesize that banks increase tax avoidance under stress tests through our defined broad forms of tax planning activities discussed in Section 3. We find strong evidence that stress-test banks tax avoid by increasing charge-offs between 0.1% and 0.2% relative to the control group of banks, suggesting that treated banks accelerate the recognition of bad loans to take advantage of tax deductions. This translates to an increase of roughly $53M to $186M in bad loans written off in stress-test banks compared to non-stress-test banks. We also find some evidence, though weak, to suggest that stress-test banks tend to have lower levels of loan loss provisions (a significant decrease of 18.5% only in the quadratic specifications for our all relevant banks sample), providing some indication of the use of income smoothing mechanisms to boost their capital reserves on account of tax-avoiding and added transparency brought on by the DFA (Balla, Romero, & Rose, 2012). Additionally, the evidence shows that treated banks engage in debt financing, which provides tax shield benefits, and that interest payments, particularly, the net interest paid (between 0.076% and 0.39%, depending on the bandwidth specifications), tend to be higher for these banks relative to less tax-avoiding banks. Finally, our results show that stress-test banks accelerate the recognition of non-interest expenses (an increase of 0.25–1.34% points, depending on the bandwidth specification); see Table 4.
6.2 Bank effective tax planning
Our findings indicate that stress-test banks increase tax planning under the DFA. Given the regulatory attention and transparency requirement associated with the DFA, it raises the question of how effective stress-test banks are in tax planning. We use the Effective Tax Planning Score from Schwab et al. (2022) to investigate this question. We find that stress-test banks tend to be approximately 18–37% points less effective at tax planning relative to non-stress-test banks, according to the most conservative results from our linear specification. That is, for every aggregate unit of inputs invested in tax avoidance purposes, non-stress-test banks can get 18–37% points more in after-tax returns than stress-test banks, as shown in Table 5. This evidence suggests that although the regulatory attention and uncertainty that come with stress testing increase bank tax avoidance activities, treated banks are less efficient at tax avoidance than banks in the control group. This is consistent with the notion that tax avoidance would be more difficult under the added transparency requirement. According to Schwab et al. (2022), firms, in our case treated banks, could reach the efficient frontier (the highest effective tax planning score of the best firms within their respective industry-year) by either increasing their after-tax returns given their current level of inputs or by achieving the same after-tax return by decreasing their inputs accordingly. This finding suggests that under DFA, stress-test banks are either over-investing in the inputs for tax avoidance or not optimizing their after-tax returns given their current input levels. This evidence highlights important bank tax avoidance implications that we further discuss in Section 6.4.
6.3 Testing the tax planning mechanisms
Next, we explore potential mechanisms through which bank stress testing affects bank tax avoidance. Bozanic et al. (2017) find that public and private financial disclosure requirements, including SEC financial disclosure requirements, influence regulatory attention from different agencies, (e.g. the IRS). Similarly, Table 6 shows that stress tests show some evidence of an increase in IRS attention for treated banks.
Generally, we claim the DFA, which brought about added regulatory attention and transparency requirements, creates regulatory uncertainty in banks, contributing to a rise in banks’ tax planning. Our analysis to test the uncertainty mechanism proceeds in two stages. First, we employ the RDD to examine the effect of the DFA on uncertainty and report the results in Table 7. We show that the regulatory uncertainty, proxied with political risk, significantly increases for stress-test banks relative to non-stress-test banks (between 0.03 and 0.05). We also find that the tax-related regulatory uncertainty (using the political tax risk as a proxy) increases in one of our specifications, while the regulatory uncertainty sentiment (using the political risk sentiment as a proxy) decreases by 0.08 standard deviations using the MSE optimal bandwidth. We recognize that the regulatory uncertainty, which shows both localized as well as broader effects on stress testing, is the most direct channel affected by stress testing, while the impacts on the other constructs are less clear based on the empirical analysis. Using the uncertainty sentiment associated with the portfolio risk culture driver (Owusu & Gupta, 2023; García et al., 2024) as a proxy, we find that the annual regulatory uncertainty increases between 0.30 and 1.42 standard deviations for the banks above the policy threshold at the localized level as well as for broader effects, significant at the 99% confidence level.
We triangulate our results using standard measures for firm-level performance uncertainty (Allayannis & Weston, 2003). First, we use two measures for earnings volatility, namely, the standard deviations of the diluted and the basic earnings per share excluding extraordinary items (Earnings Volatility1 and Earnings Volatility2, respectively). Second, we use two measures for cash flow volatility – the standard deviations of the cash flow scaled by a number of shares and cash flow scaled by total assets (Cash Flow Volatility1 and Cash Flow Volatility2, respectively). We report the results in Table 8. While they are largely insignificant when we employ the relaxed bandwidth that tests all relevant banks between $10B and $200B in total assets [33], we find a statistically significant increase in earnings volatility (roughly +0.30 standard deviations) and in cash flow volatility (between +0.004 and +1.08 standard deviations) for stress-test banks, signifying an increase in uncertainty in stress-test banks compared to non-stress-test banks.
Next, we directly test whether the increased uncertainty drives tax planning activity in stress-test banks using a difference-in-differences methodology. We create dummy variables that equal one if a bank is in the top tercile of banks with the largest increase in the corresponding index (the regulatory uncertainty, the regulatory uncertainty sentiment, the tax-related regulatory uncertainty and the portfolio uncertainty) from pre-Dodd–Frank (2005–2010) to post-Dodd–Frank (2011–2016) and a value of zero otherwise – using banks with total assets between $10B and $200B. We find that tax planning activities are prevalent in banks in the top tercile with the largest increase in regulatory and tax-related uncertainty, as evidenced by a 0.13–0.24% points decline in Cash ETR at the annual and three-year rolling average level and an increase in Tax Aggressiveness of 0.2% points at the three-year moving average level. Our results for the portfolio uncertainty are insignificant; see Table 9. These findings support our argument that regulatory uncertainty and attention relatively increase for the treated banks under DFA, and this increase in regulatory uncertainty leads to higher tax planning, providing a causal link between regulatory uncertainty and tax planning.
In the next section, we discuss the tax avoidance implications under CCAR stress tests: that is, the impact on capital ratios, bank lending, performance, cost of capital and restatements of banks with a large increase in tax avoidance.
6.4 Bank tax avoidance implications under stress tests
So far, we have shown that mandatory stress tests result in higher tax planning in large stress-test banks. However, such increased tax planning is not optimally maximized in terms of after-tax returns as indicated by the effective tax planning results. An important question is, what is the consequence in terms of the bank performance for tax-avoiding banks under stress tests? For example, does the exhibition of tax avoidance post-CCAR implementation impact bank profitability or risk asset allocation? To answer these questions, we create a measure which we call the Post-DFA Intensity in Tax Avoidance, a dummy variable that takes a value of one if a bank is in the top tercile of banks with the largest increase in tax avoidance based on the Cash ETR from pre-Dodd–Frank (2005–2010) to post-Dodd–Frank (2011–2016) and a value of zero otherwise using banks with total assets between $10B and $200B. Using this new measure and causal-interaction difference-in-differences or triple difference-in-differences approach, we study these implications to determine whether tax planning activities under stress tests have a moderating impact on bank riskiness, profitability, cost of capital, loan and portfolio characteristics, bank restatements and advisory-related fees.
We start by examining the implication of bank tax avoidance on bank risk-taking. Recent literature shows that stress tests help to reduce the risk-taking behavior of treated banks relative to non-stress-test banks by reducing credit supply to risky corporate, CRE and small business borrowers (Bassett & Berrospide, 2017; Acharya et al., 2018; Cortés et al., 2020; Calem, Correa, & Lee, 2020; García & Steele, 2022). In particular, García and Steele (2022) show that bank-level measures such as the risk-weighted assets drop immediately following the implementation of stress tests and that such reduction in risk comes from stress test banks shifting to assets with lower risk-weight categories.
We analyze bank risk-taking behavior using our Post-DFA Intensity in Tax Avoidance measure interacted with banks undergoing stress tests. These banks exhibit significantly higher risk-weighted assets (4.2% points higher). The increased risk density of banks is further illustrated in Panel B of Table 10, where we find that banks with a large jump in tax avoidance reallocate assets from the lower risk-weighted categories (50%) into the highest risk-weighted assets (100%). The evidence indicates these banks increase their risk-taking activities on average relative to other banks. Our findings corroborate those of Hutchens, Rego, and Williams (2020), who find that tax avoidance activities are positive and significantly related to firm risk.
Next, we look at the implication of bank tax avoidance on bank lending and portfolio allocation. One of the feared unintended consequences of stringency in capital requirements for stress test banks is the potential reduction in bank lending in order to satisfy capital reserve conditions. However, extant literature shows that treated banks do not suffer these consequences; in fact, they maintain or increase their loan levels but focus on lending to less risky borrowers (Bassett & Berrospide, 2017; Acharya et al., 2018; Chu, Zhang, & Zhao, 2019; Bahaj & Malherbe, 2020; Cortés et al., 2020; García & Steele, 2022; Ananou, Chronopoulos, Tarazi, & Wilson, 2021) [34]. When looking at banks with a post-DFA increase in tax avoidance, we do not find a significant increase in total loans as a percentage of total assets points; see Panel C of Table 10. However, we observe a decrease in CRE and a weak increase in commercial and industrial loans (C&I) by −2.9% and 1.7%, respectively.
Panel D of Table 10 exhibits the same tests for portfolio characteristics. We find that treated banks with a jump in tax avoidance post-DFA increase their off-balance-sheet (OBS) asset items and available-for-sale (AFS) securities by 5% and 4.6%, respectively. The AFS securities usually include US treasury, government agency, corporate and municipal securities – some of which are tax-exempt securities (particularly municipal securities). These banks concurrently decrease their held-to-maturity (HTM) securities (−2.4%), cash and deposits due (−5.4%) and federal funds (−1.5%). These findings suggest that banks that increase their tax planning activities shift some of their cash and federal funds into substantially more risky assets by increasing their holdings of OBS Assets (El-Gazzar, Pastena, & Lilien, 1989) while increasing their footprint in categories that include tax-exempt securities such as the AFS securities.
We also examine the effects of tax avoidance on bank performance and profitability (Panel A of Table 11). Our analysis reveals no statistically significant causal interaction effects on gross profitability, Tobin’s Q, return on equity, return on assets or net interest margin. In other words, we find no evidence that banks engaging in increased tax avoidance post-DFA are financially constrained or underperforming. This may also suggest that increased tax planning activities helped these banks meet capital and regulatory requirements without compromising profitability. Our findings are consistent with those of Jin et al. (2022) that find that financially constrained banks generally engage in more tax avoidance but are less likely to do so after the DFA.
Regarding the cost of capital, our results show that banks with a large jump in tax avoidance activities post-CCAR exhibit an increased cost of equity, consistent with findings that tax avoidance creates information risk, which leads to an increase in the cost of capital (Chen, Huang, Pereira, & Wang, 2011; Shin & Woo, 2017).
Lastly, we look at the implication of bank tax avoidance on restatements and accounting-related fees. Surprisingly, we find that stress-test banks with a large increase in tax avoidance post-DFA do not experience an increase in the probability of receiving a restatement; see Panel C of Table 11. This is perhaps because these banks tend to invest more in tax and financial reporting resources in the form of accounting, auditing and legal expertise to conduct tax planning, consistent with Chen, Chang, and Lee (2020). We find statistically significant evidence of an increase in legal expenses. We also see a rise in accounting and auditing expenses. However, the coefficient is not statistically significant. We find no effects on other consulting costs. These findings suggest that banks with a post-DFA increase in tax avoidance under DFA likely increase their use of legal services to help navigate regulatory hurdles and exploit tax loopholes while minimizing the negative consequences of loss of reputation and higher IRS penalties associated with higher tax planning.
7. Robustness checks
In our final set of tests, we examine the robustness of our baseline results that show increased tax planning in stress-test banks. In particular, we analyze the sensitivity of our results to our experimental setting and empirical design.
For robustness check and comparison purposes, we summarize our key baseline results on tax planning in Table 12, showing an increase in tax avoidance and tax aggressiveness due to the implementation of the DFA. We discuss first our set of robustness tests focusing on our experimental setting followed by a set of robustness tests focusing on our empirical design assumptions. The results are presented in Tables 13 and A7.
First, we restrict our RDD analysis to 2013–2016, for which the policy threshold was lowered to $50B from $100B in previous years. Table 13 presents the findings for our tax avoidance and tax aggressiveness measures, which are consistent with our baseline results, indicating that our results are not being driven solely by the first set of stress tests using the $100B policy threshold [35].
Next, we validate our baseline RDD results by conducting our RDD experiments for a period before the passage of the CCAR stress testing regulation (pre-tests). Table 14 shows the results for our key baseline variables for the pre-Dodd–Frank years of 2004–2010 and the pre-recession years of 2004–2007. The coefficients for both our primary and secondary measure of tax avoidance, Cash ETR and the Tax Aggressiveness Index respectively, are statistically insignificant across both linear and quadratic specifications for both periods of analysis. Thus, our pre-tests validate our hypotheses.
We also conduct falsification tests, wherein we run our RDD model with false cutoffs to explore whether the policy thresholds are the ones driving the results. We use false thresholds at ±10% of the true thresholds of $100B for 2011–2012 and $50B for 2013–2016. If we were to find that the effects of stress testing on tax avoidance are similar in significance to our baseline results, then, our main experimental findings would be invalidated, suggesting that the differential outcomes are likely driven by factors external to bank stress tests. Using a false cutoff of 10% below the true policy thresholds, we find a change in signs compared to the baseline results for tax avoidance and tax aggressiveness measures, hence supporting our main findings (Columns 1–2 in Table 15). When we look at the false cutoff of 10% above the actual policy thresholds, we see either a change in sign or no statistical significance for our tax avoidance and tax aggressiveness measures (Columns 3–4).
Our last set of robustness checks focuses on directly testing two of the main assumptions of the RD design. The first fundamental RDD assumption is local continuity in our study’s assignment variable or bank size. Recent research (Ballew, Iselin, & Nicoletti, 2021) documents that small banks below regulation thresholds of $10B slow down growth by engaging in divestitures to avoid costs imposed on banks above certain asset size thresholds. If large banks are to manipulate asset size and hence self-select which side of the threshold they fall under in order to avoid regulatory compliance, our results would not be accurate considering the violation in measuring the causal impact of stress testing on tax avoidance. Hence, the assumption of local continuity is imperative to highlight the causal treatment effect of CCAR stress testing on bank tax avoidance and tax aggressiveness. We use the standard McCrary (2008) manipulation test to evaluate the hypothesis of continuity of the running variable’s density against the alternative hypothesis of discontinuity at the cutoff [36]. If a discontinuity exists, it would suggest that banks sort themselves below the policy cutoff to avoid having to comply with stress tests. As shown by García and Steele (2022) and confirmed by our results in Figure 2, there does not appear to be a discontinuity in the density. These results help ensure that the only reason for different outcomes around the threshold is the treatment [37].
The other main assumption of the RD design is that the baseline covariates should be balanced to maintain a local natural experiment’s special characteristics. That is, bank characteristics should have similar distributions on both sides of the cutoff as long as the bandwidth is sufficiently small. A typical test for this is to implement a simple RDD, as in Equation (1), with each covariate as the dependent variable for different bandwidth sizes. Coefficients that are insignificant from zero would indicate balanced covariates. Our set of controls includes different performance and lending ratios. We show the balanced covariates test in Table A6, following García and Steele (2022) and replicating their results. The findings show that apart from the ratio of foreign government loans, the control variables are balanced and persistent, even though some may be endogenous. Hence, stress tests likely affect these variables as well. Similarly, we include the one-period lags as our controls to reduce potential reverse causality concerns.
Lastly, we test the sensitivity of our tax avoidance and tax aggressiveness results to the inclusion and exclusion of baseline covariates. Table A7 shows that when we remove a partial set or the complete set of covariates, our results remain robust for our primary measure (Cash ETR) and, to a lesser extent, for our TAI [38].
8. Conclusion
This paper studies the impact of mandatory stress tests on bank tax planning. We find that the stress test implementation results in higher tax planning in large banks using our tax avoidance and tax aggressiveness measures. However, such an increase in tax planning is not optimally maximized, resulting in lower effective tax planning for treated banks relative to non-stress-test banks. Our results are robust to alternative measures of tax planning and a battery of robustness checks such as pre-tests, falsification tests, bank-size manipulation tests and covariate sensitivity tests. We find strong evidence that bank stress tests increase bank-level IRS attention and regulatory uncertainty affecting bank tax planning activities, supporting our theoretical mechanism.
Using the subgroup of banks with increased intensity in tax avoidance compared to the pre-DFA period, we find that these banks tax avoid by accelerating the recognition of bad debt through charge-offs and the acceleration of net interest payments and tax-deductible expenses, both of which offer tax shield benefits. Additionally, we find some indication that these banks engage in more debt financing, which has the advantage of the tax treatment of interest payments. However, we find no evidence that financially constrained or low-performing banks drive bank tax avoidance under DFA. Contrary to the objectives of CCAR stress tests, these banks take on more risk and hold on to riskier assets. Subsequently, these banks spend more on legal fees, suggesting increased DFA-related regulatory hurdles and tax planning activities.
Our findings have a direct policy implication. They show that stress testing has the unintended consequences of increasing tax planning activities and consequently increasing risk-taking on banks with high tax avoidance, which goes against the goals of stress testing regulations.
Figures
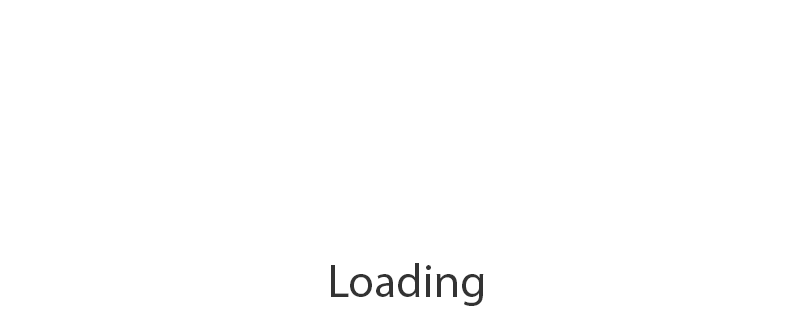
Figure 2
McCrary (2008) test graphs around the policy thresholds for 2011–2016
Key variable definitions
Variable | Variable definition |
---|---|
Panel A: tax avoidance measures | |
Cash ETR | Cash taxes paid (TXPD) divided by Pretax book income (PI) before Special Items (SPI) (defined by Dyreng et al. (2008)) |
GAAP ETR | Total tax expense (TXT) divided by pretax income (PI) |
3-year Cash ETR | Three-year rolling sum of cash taxes paid (TXPD) over years t, t–1 and t–2 scaled by the sum of pretax income (PI) minus special items (SPI) over years t, t–1 and t–2 |
3-year GAAP ETR | Three-year rolling sum of total tax expense (TXT) over years t, t–1 and t–2 scaled by the sum of pretax income (PI) over years t, t–1 and t–2 |
Cash tax aggressiveness (TA) | Bank’s mean asset size by year Cash ETR less the Bank’s Cash ETR, where Cash ETR is the cash taxes paid (TXPD) scaled by the pretax income (PI) minus special items (SPI), following Balakrishnan et al. (2019) |
GAAP TA | Bank’s mean asset size by year GAAP ETR less the firm’s GAAP ETR, where GAAP ETR is the total tax expense (TXT) scaled by the pretax income (PI), following Balakrishnan et al. (2019) |
Net charge-offs | Debt that bank is not likely to recover scaled by Total Assets |
3-year Cash TA | Bank’s mean asset size by year Cash ETR less the Bank’s Cash ETR, where Cash ETR is the three-year rolling sum of cash taxes paid (TXPD) over years t, t–1 and t–2 scaled by the sum of pretax income (PI) minus special items (SPI) over years t, t–1 and t–2, following Balakrishnan et al. (2019) |
3-year GAAP TA | Bank’s mean asset size by year GAAP ETR less the firm’s GAAP ETR, where GAAP ETR is the three-year rolling sum of total tax expense (TXT) over years t, t–1 and t–2 scaled by the sum of pretax income (PI) over years t, t–1 and t–2, following Balakrishnan et al. (2019) |
Tax aggressiveness index | A composite measure that is the sum of the Cash TA and the GAAP TA |
3-yr tax aggressiveness index | A composite measure that is the sum of the 3-year Cash TA and the 3-year GAAP TA |
Effective tax planning score1 | A measure for how efficiently firms maximize their after-tax returns by employing data envelopment analysis (DEA), developed by Schwab et al. (2022) and theoretically aligned with the Scholes-Wolfson paradigm (Scholes et al., 1992). The raw scores increase from zero to one in tax effectiveness |
Effective tax planning score2 | A measure for how efficiently profitable firms maximize their after-tax returns by employing data envelopment analysis (DEA), developed by Schwab et al. (2022) and theoretically aligned with the Scholes–Wolfson paradigm (Scholes et al., 1992). The raw scores increase from zero to one in tax effectiveness |
IRS attention | Tracks the attention that a firm receives from the Internal Revenue Service, calculated by the number of times in a year that a 10-K or a 10-Q document is downloaded from the EDGAR database, by an IP address matching that of the IRS’ IP addresses (Bozanic et al., 2017) |
Post-DFA intensity in tax avoidance | Terciles based on the highest increase in tax avoidance (measured using Cash ETR) after the implementation of Dodd–Frank compared to pre-Dodd–Frank period |
Panel B: bank characteristics | |
Risk density | Risk-weight Assets scaled by Total Assets |
Capital ratio | Total Capital/Risk Weighted Assets |
Tier 1 ratio | Tier 1 Capital/Risk Weighted Assets |
Leverage ratio | Tier 1 Capital/Total Assets |
Total equity/assets | Shareholder Equity to Asset Ratio |
Total deposits/assets | Total Deposits scaled by Total Assets |
Available loan loss reserves/total loans | Reserves available to cover loan losses scaled by Total Loans |
Problem loans % | Percentage of loans that are problem loans |
Loans/assets | Total Loans scaled by Total Assets |
CRE loans/assets | Commercial Real Estate Loans scaled by Total Assets |
RRE loans/assets | Residential Real Estate Loans scaled by Total Assets |
Consolidated agricultural loans/assets | Consolidated Agricultural loans scaled by Total Assets |
C&I loans/assets | Commercial and Industrial Loans scaled by Total Loans |
Consumer loans/assets | Consumer Loans scaled by Total Assets |
Consolidated loans to foreign govts/total loans | Consolidated Loans to foreign governments scaled by Total Loans |
Off balance sheet assets/assets | Off Balance Sheet Assets scaled by Total Assets |
Loans HFS/assets | Held-For-Sale Loans scaled by Total Assets |
AFS securities/assets | Available-For-Sale Securities scaled by Total Assets |
HTM securities/assets | Held-To-Maturity Securities scaled by Total Assets |
Cash and deposits due/assets | Cash and Deposits Due scaled by Total Assets |
Federal funds/assets | Federal Funds scaled by Total Assets |
Other assets/assets | Other Assets scaled by Total Assets |
Return on equity | Profitability measure, calculated as net income by Shareholder Equity |
Return on assets | Profitability measure, calculated as net income by total assets |
Net interest margin | Long-term Profitability measure, measured as the difference between interest received and interest paid |
Net non-interest margin | measured as the difference between non-interest earnings received and non-interest paid. Non-interest earnings include subscription fees, penalty fees, transaction fees and inactivity fees |
Net income | Revenue net of expenses, interest and taxes |
Profitability | EBITDA/Total Assets |
Gross profitability | (Revenue – Cost of Goods Sold)/Total Assets |
Dividend payout ratio | Dividends paid by the bank divided by net income |
Panel C: uncertainty and political risk sentiments | |
Regulatory uncertainty | Hassan et al. (2019) use a pattern-based sequence-classification method on earnings call transcripts to determine the risk surrounding political-related discourse in earnings conference call transcripts |
Regulatory uncertainty sentiment | Hassan et al. (2019) use the positive and negative sentiments from the Loughran and McDonald (2011) Sentiment Word List to extract sentiments related to political risk in earnings calls |
Tax-related regulatory uncertainty | Political risk focused on tax policies, extracted using computational linguistic tools on bank earnings call by Hassan et al. (2019) |
Portfolio uncertainty | The bank uncertainty sentiment related to the communicated “Portfolio” risk culture driver, obtained from Owusu and Gupta (2023) |
Earnings volatility | Our first set of measures for bank uncertainty is the measured as the expectation of future earnings volatility. It is calculated as the standard deviation of the earnings over the periods t+1, t+2 and t+3. The diluted earnings before extraordinary items and the basic earnings per share without extraordinary items are the two measures of earnings that we use Allayannis and Weston (2003) |
Cash flow volatility | Our second set of measures for bank uncertainty is the measured as the expectation of future cash flow volatility. It is calculated as the standard deviation of the cash flow over the periods t+1, t+2 and t+3. The cash flow scaled by the number of shares and the cash flow scaled by assets are the two measures of cash flow that we use Allayannis and Weston (2003) |
Note(s): This table reports definitions for our key independent and dependent variables. Panel A comprises the definitions for our tax avoidance measures, which include the Cash ETR, the GAAP ETR, the Cash and GAAP Tax Aggressiveness, the three-year rolling averages of these four measures, the TAI, the effective tax planning scores (Schwab et al., 2022), the IRS attention (Bozanic et al., 2017) and the post-DFA increase in tax avoidance. Panel B contains our bank's characteristic variables, such as risk ratios, profitability measures, balance sheet items, loans and other financial ratios. Finally, Panel C constitutes the definitions for our tax uncertainty measures, including the firm-level political risk measures obtained from Hassan et al. (2019), which we use as proxies for regulatory uncertainty, and portfolio uncertainty (Owusu & Gupta, 2023)
Source(s): Authors’ own work
Summary statistics for 2011–2016
Variable | All banks | Stress tested | Non-stress tested | ||||||
---|---|---|---|---|---|---|---|---|---|
N | Mean | Std. Dev | N | Mean | Std. Dev | N | Mean | Std. Dev | |
Panel A: tax avoidance measures | |||||||||
Cash ETR | 381 | 0.2229 | 0.2214 | 122 | 0.1985 | 0.2050 | 259 | 0.2343 | 0.2282 |
GAAP ETR | 381 | 0.2545 | 0.2721 | 122 | 0.2481 | 0.2438 | 259 | 0.2575 | 0.2849 |
Net Charge-Offs | 248 | −0.0026 | 0.0033 | 65 | −0.0029 | 0.0027 | 183 | −0.0026 | 0.003 |
3-year Cash ETR | 381 | 0.2363 | 0.2712 | 122 | 0.1663 | 0.1805 | 259 | 0.2693 | 0.2994 |
3-year GAAP ETR | 381 | 0.2414 | 0.3041 | 122 | 0.2033 | 0.2833 | 259 | 0.2593 | 0.3124 |
Tax aggressiveness index | 381 | −0.0022 | 0.2331 | 122 | 0.0315 | 0.2302 | 259 | −0.0181 | 0.2332 |
3-yr tax aggressiveness index | 381 | −0.0011 | 0.2435 | 122 | 0.0867 | 0.1928 | 259 | −0.0424 | 0.2540 |
Cash tax aggressiveness | 381 | −0.0038 | 0.1605 | 122 | 0.0213 | 0.1481 | 259 | −0.0157 | 0.1649 |
GAAP tax aggressiveness | 381 | 0.0016 | 0.1219 | 122 | 0.0101 | 0.1454 | 259 | −0.0024 | 0.1092 |
3-year cash tax aggressiveness | 381 | −0.0030 | 0.1749 | 122 | 0.0529 | 0.1289 | 259 | −0.0294 | 0.1873 |
3-year GAAP tax aggressiveness | 381 | 0.0020 | 0.1521 | 122 | 0.0337 | 0.1291 | 259 | −0.0130 | 0.1598 |
Effective tax planning score1 | 242 | 0.4936 | 0.2586 | 51 | 0.5183 | 0.2818 | 191 | 0.4871 | 0.2525 |
Effective tax planning score2 | 238 | 0.5001 | 0.2556 | 50 | 0.5272 | 0.2774 | 188 | 0.4929 | 0.2498 |
IRS attention | 232 | 24.2155 | 47.8326 | 83 | 49.5783 | 67.4752 | 149 | 10.0872 | 22.0635 |
Post-DFA intensity in tax avoidance | 381 | 0.1969 | 0.3981 | 122 | 0.2295 | 0.4223 | 259 | 0.1815 | 0.3862 |
Panel B: bank characteristics | |||||||||
Return on assets | 299 | 0.0278 | 0.0357 | 100 | 0.0281 | 0.0190 | 199 | 0.0277 | 0.0417 |
Total equity/assets | 299 | 0.1209 | 0.0618 | 100 | 0.1101 | 0.0188 | 199 | 0.1263 | 0.0740 |
Total deposits/assets | 299 | 0.6542 | 0.2012 | 100 | 0.5102 | 0.2349 | 199 | 0.7265 | 0.1326 |
Cost to income ratio | 299 | 0.7052 | 0.1267 | 100 | 0.7210 | 0.1452 | 199 | 0.6975 | 0.1162 |
Available loan loss reserves/total loans | 299 | 0.0141 | 0.0073 | 100 | 0.0146 | 0.0085 | 199 | 0.0139 | 0.0066 |
Problem loans % | 299 | 0.0134 | 0.0155 | 100 | 0.0179 | 0.0170 | 199 | 0.0112 | 0.0141 |
CRE loans/loans | 299 | 0.1789 | 0.1398 | 100 | 0.0665 | 0.0712 | 199 | 0.2354 | 0.1315 |
RRE loans/loans | 299 | 0.1443 | 0.0952 | 100 | 0.1114 | 0.0838 | 199 | 0.1609 | 0.0965 |
Consolidated agricultural loans/loans | 299 | 0.0020 | 0.0066 | 100 | 0.0010 | 0.0017 | 199 | 0.0024 | 0.0080 |
C&I loans/loans | 299 | 0.1340 | 0.0879 | 100 | 0.1159 | 0.0906 | 199 | 0.1430 | 0.0853 |
Consumer loans/loans | 299 | 0.0667 | 0.1209 | 100 | 0.1277 | 0.1713 | 199 | 0.0361 | 0.0671 |
Consolidated loans to foreign governments/total loans | 299 | 0.0001 | 0.0006 | 100 | 0.0003 | 0.0008 | 199 | 0.0001 | 0.0003 |
Risk density | 299 | 0.7495 | 0.1756 | 100 | 0.7217 | 0.2164 | 199 | 0.7635 | 0.1496 |
Capital ratio | 299 | 15.8416 | 5.4749 | 100 | 15.5727 | 2.3519 | 199 | 15.9768 | 6.5033 |
Tier 1 ratio | 299 | 13.9438 | 5.5440 | 100 | 13.1251 | 2.2225 | 199 | 14.3551 | 6.5789 |
Leverage ratio | 299 | 9.5064 | 1.6231 | 100 | 9.1493 | 1.3966 | 199 | 9.6332 | 1.6815 |
Loans/assets | 299 | 0.5189 | 0.1959 | 100 | 0.4225 | 0.2299 | 199 | 0.5673 | 0.1557 |
Off balance sheet assets/assets | 299 | 0.1563 | 0.1425 | 100 | 0.2621 | 0.1794 | 199 | 0.1031 | 0.0772 |
Loans HFS/assets | 299 | 0.0081 | 0.0210 | 100 | 0.0068 | 0.0112 | 199 | 0.0087 | 0.0245 |
AFS securities/assets | 299 | 0.1410 | 0.0993 | 100 | 0.1051 | 0.0571 | 199 | 0.1590 | 0.1106 |
HTM securities/assets | 299 | 0.0413 | 0.0754 | 100 | 0.0222 | 0.0259 | 199 | 0.0508 | 0.0891 |
Cash and deposits due/assets | 299 | 0.0555 | 0.0469 | 100 | 0.0769 | 0.0530 | 199 | 0.0447 | 0.0396 |
Federal funds/assets | 299 | 0.0147 | 0.0521 | 100 | 0.0379 | 0.0846 | 199 | 0.0031 | 0.0101 |
Other assets/assets | 299 | 0.0614 | 0.0435 | 100 | 0.0622 | 0.0417 | 199 | 0.0611 | 0.0445 |
Return on equity | 299 | 0.2179 | 0.1133 | 100 | 0.2503 | 0.1427 | 199 | 0.2017 | 0.0914 |
Net interest margin | 299 | 0.0682 | 0.0283 | 100 | 0.0625 | 0.0398 | 199 | 0.0710 | 0.0196 |
Net non-interest margin | 299 | −0.0225 | 0.0557 | 100 | −0.0144 | 0.0294 | 199 | −0.0265 | 0.0647 |
Net income | 299 | 5.4e+06 | 1.2e+07 | 100 | 1.5e+07 | 1.7e+07 | 199 | 6.3e+05 | 7.7e+05 |
Dividend payout ratio | 299 | 0.5437 | 3.7832 | 100 | 0.9438 | 6.5323 | 199 | 0.3426 | 0.2908 |
Panel C: uncertainty and political risk sentiment | |||||||||
Regulatory uncertainty | 299 | −0.0163 | 0.7718 | 65 | −0.0131 | 0.6018 | 234 | −0.0171 | 0.8139 |
Regulatory uncertainty sentiment | 299 | 0.2950 | 0.7481 | 65 | 0.2690 | 0.5542 | 234 | 0.3022 | 0.7945 |
Tax-related regulatory uncertainty | 299 | −0.0173 | 0.7301 | 65 | −0.0785 | 0.3518 | 234 | −0.0003 | 0.8040 |
Portfolio uncertainty | 299 | −0.1019 | 0.5574 | 65 | −0.0660 | 0.5882 | 234 | −0.1118 | 0.5494 |
Volatility of diluted earnings per share | 299 | 5.6075 | 48.3604 | 65 | 14.8984 | 80.8811 | 234 | 0.5447 | 0.9820 |
Volatility of basic earnings per share | 299 | 5.6155 | 48.3598 | 65 | 14.9116 | 80.8791 | 234 | 0.5500 | 0.9843 |
Volatility of cash flow by assets | 299 | 18.0201 | 149.2024 | 65 | 47.36125 | 249.2026 | 234 | 2.0318 | 8.1260 |
Volatility of cash flow by shares | 299 | 0.0094 | 0.0354 | 65 | 0.0076 | 0.0063 | 234 | 0.0104 | 0.0438 |
Note(s): Summary statistics for 2011–2016. This table reports summary statistics for publicly traded BHCs over the period 2011–2016 for tax planning measures in Panel A, for bank characteristics in Panel B and the uncertainty and political risk sentiment measures in Panel C. Regulatory Uncertainty, Regulatory Uncertainty sentiment and the tax-related Regulatory Uncertainty, which are proxies for the standardized firm-level political risk, political risk sentiment and the political tax risk, are obtained from the dataset provided by Hassan et al. (2019). Portfolio Uncertainty is constructed using the standardized measure of the uncertainty sentiment related to the portfolio risk culture driver, obtained from Owusu and Gupta (2023), it captures the uncertainty as it pertains to the characteristics of the banks’ balance sheet. The sample is restricted to banks that have at least $10B in assets, the Federal Reserve cutoff for community banks. Balance sheet data comes from FR Y-9C reports. Dollar amounts are in 2010 thousands of dollars, and equity price data/forecasts come from CSPS and I/B/E/S databases. Banks are stress-tested if they have at least $100B in assets in years 2011 and 2012, and if they have at least $50B in assets in the following years
Source(s): Authors’ own work
Effect of stress tests on bank tax planning
Variable | All relevant banks | MSE optimal | ||
---|---|---|---|---|
Linear | Quadratic | Linear | Quadratic | |
Panel A: tax avoidance and tax aggressiveness measures | ||||
Cash ETR | −0.188*** | −0.467*** | −0.660*** | −2.386*** |
(0.047) | (0.056) | (0.036) | (0.183) | |
[223] | [223] | [67] | [67] | |
GAAP ETR | 0.034 | −0.078 | −0.670*** | −1.395*** |
(0.052) | (0.085) | (0.090) | (0.189) | |
[226] | [226] | [69] | [69] | |
Tax aggressiveness index | −0.001 | 0.216** | 0.067 | 1.001*** |
(0.063) | (0.092) | (0.059) | (0.166) | |
[223] | [223] | [67] | [67] | |
Panel B: tax avoidance and tax aggressiveness 3-yr M.A. | ||||
3yr Cash ETR | −0.169** | −0.238 | −0.033 | 0.110 |
(0.085) | (0.145) | (0.084) | (0.176) | |
[223] | [223] | [67] | [67] | |
3yr GAAP ETR | 0.068 | 0.055 | −0.577*** | −0.874*** |
(0.076) | (0.099) | (0.057) | (0.085) | |
[226] | [226] | [69] | [82] | |
3yr tax aggressiveness | 0.017 | 0.014 | 0.491*** | 0.934*** |
(0.058) | (0.084) | (0.052) | (0.075) | |
[223] | [223] | [67] | [67] | |
Controls | Y | Y | Y | Y |
Fixed-effects | Y | Y | Y | Y |
Note(s): This table reports the regression discontinuity coefficients for the tax planning variables of interest. The table reports both the local linear and quadratic regression discontinuity coefficients first by specifying a sub-sample of “All Relevant Banks,” which includes banks between $10B and $200B in total asset size, and second, by using the MSE optimal bandwidth measure. The table reports the results for the annual Cash and GAAP Effective Tax Rate and the TAIin Panel A. Panel B reports the 3-year Cash (GAAP) Effective Tax Rate and the 3-year TAI. A negative Cash and GAAP ETR indicates an increase in tax avoidance. Regressions are triangular kernel weighted and are allowed to capture more banks in a larger bandwidth in the first two columns and are restricted to having at least 65 observations within the selected optimal bandwidth in the last two columns. Control variables include the first 12 bank characteristics shown in Table 2. All regressions include bank and year-fixed effects. Standard errors are clustered at the bank level and are reported in parentheses while observation counts are reported in brackets. ***, ** and * indicate significance at the 1%, 5% and 10% levels, respectively
Source(s): Authors’ own work
Effect of bank stress tests on bank-specific tax planning activities
Variable | All relevant banks | MSE optimal | ||
---|---|---|---|---|
Linear | Quadratic | Linear | Quadratic | |
Panel A: net charge-offs and loan and lease losses | ||||
Net charge-offs | 0.001** | 0.002*** | 0.001** | 0.001** |
(0.000) | (0.001) | (0.000) | (0.000) | |
[193] | [193] | [74] | [62] | |
Loan and lease loss provision | −0.013 | −0.185** | −0.069 | −0.044 |
(0.076) | (0.086) | (0.047) | (0.099) | |
[256] | [256] | [74] | [74] | |
Loan and lease loss allowance | 0.018 | −0.001 | 0.024 | −0.025 |
(0.036) | (0.051) | (0.038) | (0.047) | |
[256] | [256] | [74] | [114] | |
Panel B: interest expenses | ||||
Net interest paid | 0.076** | 0.092** | 0.226*** | 0.386*** |
(0.032) | (0.045) | (0.022) | (0.042) | |
[226] | [226] | [66] | [66] | |
Non-interest expenses | 0.246* | 0.429*** | 1.115*** | 1.342*** |
(0.128) | (0.135) | (0.094) | (0.224) | |
[256] | [256] | [74] | [88] | |
Controls | Y | Y | Y | Y |
Fixed-effects | Y | Y | Y | Y |
Note(s): This table reports the regression discontinuity coefficients for the bank-specific tax planning activities. The table reports both the local linear and quadratic regression discontinuity coefficients first by specifying a sub-sample of “All Relevant Banks,” which includes banks between $10B and $200B in total asset size, and second, by using the MSE optimal bandwidth measure. The table reports the results for the Loan and Lease Loss Provision and Allowance, the Net Charge-Offs in Panel A, and the Interest Paid and Non-Interest Expenses in Panel B. Regressions are triangular kernel weighted and are allowed to capture more banks in a larger bandwidth in the first two columns and are restricted to having at least 64 observations within the selected optimal bandwidth in the last two columns. Control variables include the first 12 bank characteristics shown in Table 2. All regressions include bank and year-fixed effects. Standard errors are clustered at the bank level and are reported in parentheses while observation counts are reported in brackets. ***, ** and * indicate significance at the 1%, 5% and 10% levels, respectively
Source(s): Authors’ own work
Effect of bank stress tests on bank effective tax planning
Variable | All relevant banks | MSE optimal | ||
---|---|---|---|---|
Linear | Quadratic | Linear | Quadratic | |
Effective tax planning score1 | −0.176** | −0.212* | −0.284*** | −0.856*** |
(0.068) | (0.125) | (0.064) | (0.124) | |
[226] | [226] | [68] | [68] | |
Effective tax planning score2 | −0.198*** | −0.241* | −0.373*** | −1.437*** |
(0.072) | (0.138) | (0.080) | (0.120) | |
[222] | [222] | [64] | [64] | |
Controls | Y | Y | Y | Y |
Fixed-effects | Y | Y | Y | Y |
Note(s): This table reports the regression discontinuity coefficients for bank effective tax planning. The table reports both the local linear and quadratic regression discontinuity coefficients first by specifying a sub-sample of “All Relevant Banks,” which includes banks between $10B and $200B in total asset size, and second, by using the MSE optimal bandwidth measure. The table reports the results for the Effective Tax Planning measures from Schwab et al. (2022) based on the tax planning paradigm described in Scholes et al. (1992). Effective Tax Planning Scoreit was created using (Schwab et al., 2022)’s full sample of firms while Effective Tax Planning Score2 uses only profitable firms in the sample. Regressions are triangular kernel weighted and are allowed to capture more banks in a larger bandwidth in the first two columns and are restricted to having at least 65 observations within the selected optimal bandwidth in the last two columns. Control variables include the first 12 bank characteristics shown in Table 2. All regressions include bank and year-fixed effects. Standard errors are clustered at the bank level and are reported in parentheses while observation counts are reported in brackets. ***, ** and * indicate significance at the 1%, 5% and 10% levels, respectively
Source(s): Authors’ own work
Effect of bank stress tests on IRS attention
Variable | All relevant banks | MSE optimal | ||
---|---|---|---|---|
Linear | Quadratic | Linear | Quadratic | |
IRS attention | 15.076*** | 2.068 | 11.282* | 7.695 |
(4.038) | (5.859) | (5.881) | (11.420) | |
[129] | [129] | [58] | [58] | |
Controls | Y | Y | Y | Y |
Fixed-effects | Y | Y | Y | Y |
Note(s): This table reports the regression discontinuity coefficients for IRS attention. The table reports both the local linear and quadratic regression discontinuity coefficients first by specifying a sub-sample of “All Relevant Banks,” which includes banks between $10B and $200B in total asset size, and second, by using the MSE optimal bandwidth measure. The table reports the results for the IRS attention received by banks, the measure is obtained from Bozanic et al. (2017). Regressions are triangular kernel weighted and are allowed to capture more banks in a larger bandwidth in the first two columns and are restricted to having at least 65 observations within the selected optimal bandwidth in the last two columns. Control variables include the first 12 bank characteristics shown in Table 2. All regressions include bank and year-fixed effects. Standard errors are clustered at the bank level and are reported in parentheses while observation counts are reported in brackets. ***, ** and * indicate significance at the 1%, 5% and 10% levels, respectively
Source(s): Authors’ own work
Effect of bank stress tests on bank uncertainty measures
Variable | All relevant banks | MSE optimal | ||
---|---|---|---|---|
Linear | Quadratic | Linear | Quadratic | |
Regulatory uncertainty | 0.006 | 0.029*** | 0.026*** | 0.049*** |
(0.008) | (0.011) | (0.006) | (0.013) | |
[192] | [192] | [66] | [106] | |
Regulatory uncertainty sentiment | −0.008 | −0.005 | −0.083*** | −0.079** |
(0.024) | (0.032) | (0.015) | (0.031) | |
[192] | [192] | [66] | [78] | |
Tax-related regulatory uncertainty | −0.001 | 0.004 | 0.008 | 0.024** |
(0.006) | (0.007) | (0.005) | (0.010) | |
[192] | [192] | [66] | [97] | |
Portfolio uncertainty | 0.295*** | 0.527*** | 0.336*** | 1.415*** |
(0.066) | (0.070) | (0.040) | (0.107) | |
[222] | [222] | [74] | [58] | |
Controls | Y | Y | Y | Y |
Fixed-effects | Y | Y | Y | Y |
Note(s): This table reports the regression discontinuity coefficients for the bank uncertainty measures. The table reports both the local linear and quadratic regression discontinuity coefficients first by specifying a sub-sample of “All Relevant Banks,” which includes banks between $10B and $200B in total asset size, and second, by using the MSE optimal bandwidth measure. The table reports the results for the following tax risk measures: Regulatory Uncertainty, Regulatory Uncertainty sentiment and tax-related Regulatory Uncertainty, which are proxies for the standardized firm-level political risk, political risk sentiment and political tax risk, obtained from the dataset provided by Hassan et al. (2019), and the Portfolio Uncertainty, which is the uncertainty sentiment captured in the “Portfolio” risk culture driver. Regressions are triangular kernel weighted and are allowed to capture more banks in a larger bandwidth in the first two columns and are restricted to having at least 65 observations within the selected optimal bandwidth in the last two columns. Control variables include the first 12 bank characteristics shown in Table 2. All regressions include bank and year-fixed effects. Standard errors are clustered at the bank level and are reported in parentheses while observation counts are reported in brackets. ***, ** and * indicate significance at the 1%, 5% and 10% levels, respectively
Source(s): Authors’ own work
Effect of bank stress tests on bank performance-related uncertainty measures
Variable | All relevant banks | MSE optimal | ||
---|---|---|---|---|
Linear | Quadratic | Linear | Quadratic | |
Earnings volatility1 | −0.119 | 0.080 | 0.304*** | −0.163 |
(0.127) | (0.183) | (0.070) | (0.200) | |
[168] | [168] | [50] | [59] | |
Earnings volatility2 | −0.122 | 0.075 | 0.295*** | −0.177 |
(0.127) | (0.183) | (0.069) | (0.199) | |
[168] | [168] | [50] | [59] | |
Cash flow volatility1 | −0.068 | −0.805 | 0.618*** | 1.081** |
(0.318) | (0.613) | (0.169) | (0.531) | |
[168] | [112] | [50] | [59] | |
Cash flow volatility2 | −0.001 | −0.002 | 0.004*** | 0.003*** |
(0.001) | (0.001) | (0.000) | (0.001) | |
[168] | [168] | [50] | [59] | |
Controls | Y | Y | Y | Y |
Fixed-effects | Y | Y | Y | Y |
Note(s): This table reports the regression discontinuity coefficients for the bank performance-related uncertainty measures. The table reports both the local linear and quadratic regression discontinuity coefficients first by specifying a sub-sample of “All Relevant Banks,” which includes banks between $10B and $200B in total asset size, and second, by using the MSE optimal bandwidth measure. The table reports the results for the bank earnings volatility and the cash flow volatility. Regressions are triangular kernel weighted and are allowed to capture more banks in a larger bandwidth in the first two columns and are restricted to having at least 50 observations within the selected optimal bandwidth in the last two columns. Control variables include the first 12 bank characteristics shown in Table 2. All regressions include bank and year-fixed effects. Standard errors are clustered at the bank level and are reported in parentheses while observation counts are reported in brackets. ***, ** and * indicate significance at the 1%, 5% and 10% levels, respectively
Source(s): Authors’ own work
Post-DFA increase in regulatory uncertainty and tax planning
Cash ETR | 3-year cash ETR | TA.I. | 3-yr TA.I. | |
---|---|---|---|---|
Panel A: regulatory uncertainty (R.U.) | ||||
Post-DFA Intensity in R.U. = 1 × StressTest = 1 | −0.132** | −0.241*** | 0.048 | 0.287*** |
(0.065) | (0.059) | (0.075) | (0.100) | |
Observations | 319 | 319 | 319 | 319 |
Adjusted R2 | 0.123 | 0.268 | 0.267 | 0.190 |
Panel B: regulatory uncertainty sentiment (R.U.S.) | ||||
Post-DFA Intensity in R.U.S. = 1 × StressTest = 1 | 0.018 | −0.412** | 0.042 | 0.202 |
(0.043) | (0.157) | (0.113) | (0.129) | |
Observations | 319 | 319 | 319 | 319 |
Adjusted R2 | 0.120 | 0.279 | 0.264 | 0.191 |
Panel C: tax-related regulatory uncertainty | ||||
Post-DFA Intensity in Tax-Related R.U. = 1 × StressTest = 1 | −0.132** | −0.241*** | 0.048 | 0.287*** |
(0.065) | (0.059) | (0.075) | (0.100) | |
Observations | 319 | 319 | 319 | 319 |
Adjusted R2 | 0.123 | 0.268 | 0.267 | 0.190 |
Panel D: portfolio uncertainty | ||||
Post-DFA Intensity in Portfolio Uncertainty = 1 × StressTest = 1 | 0.110 | −0.066 | −0.074 | 0.035 |
(0.079) | (0.051) | (0.067) | (0.093) | |
Observations | 319 | 319 | 319 | 319 |
Adjusted R2 | 0.123 | 0.268 | 0.267 | 0.190 |
Note(s): The table exhibits the marginal impact of the Dodd–Frank stress tests for banks with a Post-DFA increase in regulatory uncertainty post-Dodd–Frank compared to the pre-Dodd–Frank period on tax avoidance (Cash ETR) and TAI, using our four different measures of bank uncertainty. Post-DFA Intensity in R.U., Post-DFA intensity in R.U.S., Post-DFA Intensity in tax-related R.U. and Post-DFA Intensity in portfolio uncertainty are dummy variables equal to 1 for categories of large banks and regional banks in the top tercile increase in the regulatory uncertainty, regulatory uncertainty sentiment, the tax-related regulatory uncertainty and the portfolio-related uncertainty sentiment, respectively, in the post-implementation of Dodd–Frank period (2011–2016) compared to the years 2005–2010. StressTest is a dummy variable equal to 1 for CCAR stress test banks and zero for Non-CCAR stress test banks. The dependent variables are the contemporaneous and the three-year rolling average cash effective tax rate and the TAI. Control variables include the bank characteristics as shown in Table 2. Bank and year-fixed effects are included in the regressions. Standard errors are clustered at the bank level and are reported in parentheses. ***, ** and * indicate significance at the 1%, 5% and 10% levels, respectively
Source(s): Authors’ own work
Post-DFA increase in tax avoidance and risk ratios, lending and portfolio characteristics
Panel A: risk ratios | Risk density | Slack | Capital ratio | Tier 1 ratio | Leverage ratio |
---|---|---|---|---|---|
Post-DFA Intensity in Tax Avoidance = 1 × StressTest = 1 | 0.042** | 0.502 | 0.313 | −0.674 | −0.114 |
(0.020) | (0.380) | (0.501) | (0.571) | (0.406) | |
Observations | 340 | 340 | 340 | 340 | 340 |
Adjusted R2 | 0.939 | 0.806 | 0.928 | 0.935 | 0.963 |
Controls | Y | Y | Y | Y | Y |
Panel B: risk weights | 100% risk weight assets/assets | 50% risk weight assets/assets | 20% risk weight assets/assets | 0% risk weight assets/assets |
---|---|---|---|---|
Post-DFA Intensity in Tax Avoidance = 1 × StressTest = 1 | 0.041* | −0.014* | −0.022 | −0.022 |
(0.022) | (0.007) | (0.014) | (0.017) | |
Observations | 265 | 265 | 265 | 265 |
Adjusted R2 | 0.940 | 0.956 | 0.910 | 0.952 |
Controls | Y | Y | Y | Y |
Panel C: bank loans | Loans/assets | CRE loans/assets | RRE loans/assets | C&I loans/assets | Consumer loans/assets |
---|---|---|---|---|---|
Post-DFA Intensity in Tax Avoidance = 1 × StressTest = 1 | 0.004 | −0.029** | 0.008 | 0.017* | 0.004 |
(0.017) | (0.011) | (0.012) | (0.009) | (0.010) | |
Observations | 335 | 436 | 436 | 436 | 436 |
Adjusted R2 | 0.946 | 0.968 | 0.958 | 0.911 | 0.987 |
Controls | Y | Y | Y | Y | Y |
Panel D: portfolio characteristics | Off balance sheet assets | Loans HFS | AFS securities | HTM securities | Cash and deposits due | Federal funds | Other assets |
---|---|---|---|---|---|---|---|
Post-DFA Intensity in Tax Avoidance = 1 × StressTest = 1 | 0.050*** | −0.000 | 0.046*** | −0.024*** | −0.054*** | −0.015*** | −0.007 |
(0.017) | (0.007) | (0.015) | (0.008) | (0.015) | (0.001) | (0.004) | |
Observations | 335 | 335 | 335 | 335 | 335 | 335 | 335 |
Adjusted R2 | 0.879 | 0.515 | 0.897 | 0.923 | 0.819 | 0.535 | 0.948 |
Controls | Y | Y | Y | Y | Y | Y | Y |
Note(s): The table exhibits the marginal impact of the Dodd–Frank stress tests for banks with a Post-DFA increase in tax avoidance post-Dodd–Frank compared to the pre-Dodd Frank period on risk ratios, bank loans and balance sheet items. The portfolio characteristic variables are scaled by total assets. The risk ratios in Panel A include Risk Density (risk-weighted assets/assets), Capital Ratio, Tier 1 Ratio, Leverage Ratio and Panel B shows the impact on risk weight asset categories (100%, 50%, 20% and 0% Risk Weights) over total assets. Panel C shows the marginal impact of stress tests when interacted with a large increase in tax avoidance in the Dodd–Frank era on Total Loans, Commercial and Residential Real Estate Loans, Commercial and Industrial (C&I) Loans and Consumer loans over total assets. Finally, Panel D shows the portfolio characteristics such as Off Balance Sheet Assets, Held for Sale Loans, Available for Sale Securities, Held to Maturity Securities, Cash and Deposits Due and Federal Funds. The portfolio characteristic variables are scaled by total assets. Post-DFA Intensity in Tax Avoidance is a dummy variable equal to 1 for categories of large banks and regional banks in the top tercile increase in tax avoidance activities in the post-implementation of Dodd–Frank period (2011–2016) compared to the years 2005–2010. StressTest is a dummy variable equal to 1 for CCAR stress test banks and zero for Non-CCAR stress test banks. Control variables include the first 12 bank characteristics shown in Table 2. Bank and year-fixed effects are included in the regressions. Standard errors are clustered at the bank level and are reported in parentheses. ***, ** and * indicate significance at the 1%, 5% and 10% levels, respectively
Source(s): Authors’ own work
Post-DFA increase in tax avoidance and profitability, cost of capital and restatement and advisory fees
Panel A: profitability | Tobin-Q | Profitability | Return on equity | Return on assets | Net interest margin | Net non-interest margin |
---|---|---|---|---|---|---|
Post-DFA Intensity in Tax Avoidance = 1 × StressTest = 1 | −0.027 | 0.001 | −0.017 | −0.001 | −0.006 | −0.000 |
(0.018) | (0.002) | (0.031) | (0.005) | (0.006) | (0.009) | |
Observations | 321 | 326 | 436 | 436 | 436 | 436 |
Adjusted R2 | 0.947 | 0.966 | 0.584 | 0.871 | 0.898 | 0.938 |
Controls | Y | Y | Y | Y | Y | Y |
Panel B: cost of capital | Cost of capital | Cost of equity | Cost of debt | Dividend payout ratio |
---|---|---|---|---|
Post-DFA Intensity in Tax Avoidance = 1 × StressTest = 1 | 0.002 | 0.019** | −0.002 | 0.023 |
(0.004) | (0.008) | (0.004) | (0.051) | |
Observations | 337 | 337 | 436 | 310 |
Adjusted R2 | 0.868 | 0.897 | 0.780 | 0.699 |
Controls | Y | Y | Y | Y |
Panel C: restatements and advisory-related fees | Restatement_dummy | Acct audit expenses | Legal expense | Consult advisory expenses |
---|---|---|---|---|
Post-DFA Intensity in Tax Avoidance = 1 × StressTest = 1 | −0.038 | 0.466 | 6.592*** | −0.111 |
(0.043) | (0.402) | (0.835) | (1.228) | |
Observations | 360 | 436 | 436 | 436 |
Adjusted R2 | 0.309 | 0.727 | 0.625 | 0.678 |
Controls | Y | Y | Y | Y |
Note(s): The table below exhibits the marginal impact of the Dodd–Frank stress tests for banks with a Post-DFA increase in tax avoidance post-Dodd–Frank compared to the pre-Dodd–Frank period on profitability, Cost of Capital and restatements and advisory-related fees. The profitability measures in Panel A include Tobin’s Q, gross profitability, Return on Equity, Return on Assets, Net Interest Margin and Net Non-Interest Margin. Panel B reports our findings for Cost of Capital-cost of debt and equity and the Dividend Payout Ratio. Finally, Panel C displays the marginal impact of stress tests over a large increase in tax avoidance in the Dodd–Frank era on bank reporting quality (restatements), Accounting and Auditing Expenses, Legal Expenses, Consulting and Advisory Expenses. Post-DFA Intensity in Tax Avoidance is a dummy variable equal to 1 for categories of large banks and regional banks in the top tercile increase in tax avoidance activities in the post-implementation of Dodd–Frank period (2011–2016) compared to the years 2005–2010. StressTest is a dummy variable equal to 1 for CCAR stress test banks and zero for Non-CCAR stress test banks. Control variables include the first 12 bank characteristics shown in Table 2. Bank and year-fixed effects are included in the regressions. Standard errors are clustered at the bank level and are reported in parentheses. ***, ** and * indicate significance at the 1%, 5% and 10% levels, respectively
Source(s): Authors’ own work
Effect of bank stress tests on bank tax planning: baseline results
Variable | All relevant banks | MSE optimal | ||
---|---|---|---|---|
Linear | Quadratic | Linear | Quadratic | |
Panel A: tax avoidance | ||||
Cash ETR | −0.188*** | −0.467*** | −0.660*** | −2.386*** |
(0.047) | (0.056) | (0.036) | (0.183) | |
[223] | [223] | [67] | [67] | |
Panel B: tax aggressiveness | ||||
Tax aggressiveness index | −0.001 | 0.216** | 0.067 | 1.001*** |
(0.063) | (0.092) | (0.059) | (0.166) | |
[223] | [223] | [67] | [67] | |
Controls | Y | Y | Y | Y |
Fixed-effects | Y | Y | Y | Y |
Note(s): This table reports the regression discontinuity coefficients for the baseline results. The table reports both the local linear and quadratic regression discontinuity coefficients first by specifying a sub-sample of “All Relevant Banks,” which includes banks between $10B and $200B in total asset size, and second, by using the MSE optimal bandwidth measure. The table reports the results for the tax avoidance measure (Cash ETR) in Panel A, the TAI (composite measure by equally weighting the cash tax aggressiveness and the GAAP Tax aggressiveness) in Panel B. Regressions are triangular kernel weighted and are allowed to capture more banks in a larger bandwidth in the first two columns and are restricted to having at least 65 observations within the selected optimal bandwidth in the last two columns. Control variables include the first 12 bank characteristics shown in Table 2. All regressions include bank and year-fixed effects. Standard errors are clustered at the bank level and are reported in parentheses while observation counts are reported in brackets. ***, ** and * indicate significance at the 1%, 5% and 10% levels, respectively
Source(s): Authors’ own work
Baseline results – $50B policy threshold (2013–2016)
Variable | Linear | Quadratic |
---|---|---|
Panel A: tax avoidance | ||
Cash ETR | −0.657*** | −2.347*** |
(0.054) | (0.188) | |
[61] | [61] | |
Panel B: tax aggressiveness | ||
Tax aggressiveness index | −0.002 | 0.956*** |
(0.073) | (0.182) | |
[61] | [61] | |
Controls | Y | Y |
Fixed-effects | Y | Y |
Note(s): The table shows the regression discontinuity coefficients for the treatment years of 2013–2016. The table reports the Localized Effects for both the local linear and quadratic regression discontinuity coefficients using the MSE optimal bandwidth measure. The table reports the results for the tax avoidance measure (Cash ETR) in Panel A and the TAI (composite measure by equally weighting the cash tax aggressiveness and the GAAP Tax aggressiveness) in Panel B. Regressions are triangular kernel weighted and are restricted to having at least 50 observations within the selected optimal bandwidth. Control variables include the first 12 bank characteristics shown in Table 2. All regressions include bank and year-fixed effects. Standard errors are clustered at the bank level and are reported in parentheses while observation counts are reported in brackets. ***, ** and * indicate significance at the 1%, 5% and 10% levels, respectively
Source(s): Authors’ own work
Baseline results – pre-test periods
Variable | Pre-CCAR period (’01–’10) | Pre-recession period (’01–’07) | ||
---|---|---|---|---|
Linear | Quadratic | Linear | Quadratic | |
Panel A: tax avoidance | ||||
Cash ETR | −0.161 | 0.108 | −0.051 | −0.038 |
(0.205) | (0.293) | (0.039) | (0.045) | |
[69] | [78] | [59] | [59] | |
Panel B: tax aggressiveness | ||||
Tax aggressiveness index | 0.221 | 0.121 | 0.082 | 0.041 |
(0.145) | (0.216) | (0.059) | (0.066) | |
[64] | [78] | [50] | [59] | |
Controls | Y | Y | Y | Y |
Fixed-effects | Y | Y | Y | Y |
Note(s): This table reports the regression discontinuity coefficients for the pre-test periods. The table reports the Localized Effects for both the local linear and quadratic regression discontinuity coefficients using the MSE optimal bandwidth measure. The table reports the pre-CCAR (2001–2010) and the pre-Recession (2001–2007) results for the annual Cash Effective Tax Rate in Panel A and the TAI (composite measure by equally weighting the cash tax aggressiveness and the GAAP Tax aggressiveness) in Panel B. Regressions are triangular kernel weighted and are restricted to having at least 50 observations within the selected optimal bandwidth. Control variables include the first 12 bank characteristics shown in Table 2. All regressions include bank and year-fixed effects. Standard errors are clustered at the bank level and are reported in parentheses while observation counts are reported in brackets. ***, ** and * indicate significance at the 1%, 5% and 10% levels, respectively
Source(s): Authors’ own work
Baseline results – falsification test at ± 10% false cutoffs
Variable | 10% false cutoff below | 10% false cutoff above | ||
---|---|---|---|---|
Linear | Quadratic | Linear | Quadratic | |
Panel A: tax avoidance | ||||
Cash ETR | 1.296*** | 1.677*** | 0.053 | 0.157** |
(0.164) | (0.219) | (0.045) | (0.076) | |
[79] | [79] | [60] | [60] | |
Panel B: tax aggressiveness | ||||
Tax aggressiveness index | −0.912*** | −1.106*** | −0.003 | 0.043 |
(0.091) | (0.133) | (0.073) | (0.095) | |
[79] | [79] | [60] | [60] | |
Controls | Y | Y | Y | Y |
Fixed-effects | Y | Y | Y | Y |
Note(s): This table reports the regression discontinuity coefficients for the falsification tests. The table reports the Localized Effects for both the local linear and quadratic regression discontinuity coefficients using the MSE optimal bandwidth measure. The table reports the results of the threshold falsification tests (10% below and 10% above the natural logarithm of the bank-size policy thresholds) for the annual Cash Effective Tax Rate in Panel A and the TAI in Panel B. Regressions are triangular kernel weighted and are restricted to having at least 50 observations within the selected optimal bandwidth. Control variables include the first 12 bank characteristics shown in Table 2. All regressions include bank and year-fixed effects. Standard errors are clustered at the bank level and are reported in parentheses while observation counts are reported in brackets. ***, ** and * indicate significance at the 1%, 5%, and 10% levels, respectively
Source(s): Authors’ own work
Notes
Additional research highlights banks’ role in client profit-shifting activities (Meeks & Meeks, 2014; Merz & Overesch, 2016; Schandlbauer, 2017; Langenmayr & Reiter, 2022).
There are only a few exceptions, see Gawehn and Müller (2020) and Gawehn (2020) for a summary of the literature.
We distinguish between bank tax avoidance and tax evasion, the former being a legal practice and the latter a fraudulent one as defined by Hanlon and Slemrod (2009).
Our argument is supported by Hanlon et al. (2017), who find that firms with higher uncertainty, particularly, tax-related uncertainty, increase cash savings for future tax requirements.
See The Dodd–Frank Wall Street Reform and Consumer Protection Act of 2010 for more details. Retrieved June 15, 2021 from https://www.govinfo.gov/content/pkg/COMPS-9515/uslm/COMPS-9515.xml.
The tax avoidance literature establishes that financially constrained firms are more likely to use tax avoidance (Graham et al., 2014; Law & Mills, 2015; Edwards et al., 2016; Bayar, Huseynov, & Sardarli, 2018), we make a similar argument for stress-test banks that can use tax planning to meet their capital ratio requirements.
Our findings corroborate the results of Dyreng et al. (2019), who find that higher levels of tax uncertainty are associated with higher tax avoidance.
See the Federal Reserve’s report: The Supervisory Capital Assessment Program: Design and Implementation (April 24, 2009).
Our analysis focuses on the years 2000 to 2016 to avoid concerns related to the drastic policy threshold changes rolled out and subsequently pulled back, as well as other policy changes implemented starting in 2017 as part of Basel III, as explained later in this section.
The five required ones being the capital ratio, tier 1 ratio, common equity tier 1 (CET1) ratio, the leverage ratio and supplemental leverage ratio.
There is some evidence of stress test scenario predictability, despite the alterations to the requirements made every year (Sahin, de Haan, & Neretina, 2020; Goldstein, 2017; Ahnert et al., 2020; Schuermann, 2014).
We extend and contribute to the literature beyond the findings of Jin et al. (2022) by demonstrating that under the DFA and stress testing, regulatory uncertainty rather than financial constraints moderates tax avoidance.
Additionally, the new FAS 166/167 ruling which mandates consolidation of assets and securitization can also impact mortgage risk (Dou, Ryan, & Xie, 2018)
During our main period of analysis (2011–2016), all US financial institutions were required to follow current US GAAP along with the related supervisory guidance on the allowance for loan and lease losses. In June 2016, the Financial Accounting Standards Board (FASB) issued a new current expected credit loss (CECL) accounting standard for estimating allowances for credit losses. The bar is effective for large SEC filers beginning after December 15, 2019, and for all other banks, it takes effect beginning after December 15, 2022. Therefore, the change in the standard does not affect our results.
See Foster (2017): Retrieved June 22, 2023 from https://www.forvis.com/article/2017/12/yes-cecl-affects-taxes-too.
In our empirical results, we focus on banks around the stress test thresholds, using only banks with total assets between $10B and $200B. See Table A1 for the full list of relevant banks.
Schwab et al. (2022) data are available on the authors’ websites. They provide two effective tax planning scores, one generated using all firms available in Compustat and the second created only with firms with positive profits. In our analysis, we provide the results for both measures.
While major US bank regulatory agencies (such as the Federal Reserve, which conducts the CCAR stress tests) have statutory independence from direct political control by the executive or legislative branches, these agencies do not work in a vacuum. The rules regarding the policy cutoffs for the stress tests, which the Fed has to comply with, come from Congress – which means that the choice of the policy threshold may be politically driven. For instance, the Economic Growth, Regulatory Relief and Consumer Protection Act (or the EGRRCPA, see https://www.congress.gov/115/plaws/publ174/PLAW-115publ174.pdf for more details) passed in 2018 under the presidency of Donald J. Trump, increased the policy threshold for stress test banks to $250B from the previous $50B threshold. In 2020, under the new Joseph R. Biden administration, this cutoff was brought back down to include $50B banks in the stress tests.
GAAP ETR is defined as the income tax scaled by pre-tax income.
By focusing on Cash ETR, we avoid potential overstatement of current tax expense due to accounting for income tax benefits such as employee stock options (Chen et al., 2010).
Blouin (2014) concurs with this view and opines that tax aggressiveness should only encompass those tax avoidance activities beyond “legislated” means, activities that lack clarity, or tax avoidance activities that are considered to be “risky” due to the uncertainty of its outcomes.
While banks engage in little R&D and PP&E, Schwab et al. (2022) provide scores for financial institutions as well. Our analysis compares effective tax planning scores across banks and not across industries.
Refer to Table A2 for the summary statistics for the entire period of study between 2000 and 2016.
We changed the threshold p from $100B in 2011–2012 to $50B in 2013–2016, following changes to the policy (Cornett et al., 2020).
We also included state-year fixed effects, and the results remain consistent. Due to redundancy, the robustness check results are not displayed in the paper.
We do not employ high-order polynomials to avoid misleading estimations (Gelman & Imbens, 2014).
We implement our optimal bandwidth measures for the MSE specifications in Stata, using the rdrobust command (part of the rdrobust package). msecomb1 and cercomb1 are specified in the model as the bandwidth selectors.
A bandwidth with 65 observations is slightly larger than the ±15% bandwidth of the natural log of the bank size policy threshold, which corresponds to an asset range of -$25B to +$40B around a bank size threshold of $50B (García & Steele, 2022).
BHCs with less than $10B in assets are considered to be community banks hence we do not include them as part of our analysis. Our sample is comprised of only regional and large banks. We do not include banks above $200B in assets to avoid the confounding effects of Basel III on G-SIBs.
The MSE optimal specification employs banks closer to the cut-off and thus the effects are more linear, but as we expand our sample to employ more banks, there are both linearity as well as non-linearity effects, thus resulting in a loss of significance.
It is possible that bank stress-test failing banks are the ones driving tax planning within stress-test banks. Hence, we conduct an additional analysis using only stress-test banks and find that while failing banks are more likely to tax avoid, their effect is not statistically significant. In other words, we find no difference in tax planning between failing stress-test banks and passing stress-test banks. The results are not shown in this manuscript for redundancy purposes. It is important to note that only a few banks (9 unique banks) failed at least one of the stress tests during our period of study, see Table A3.
Given that we obtained our main baseline results using the “rdrobust” package, a standard RD package commonly used in the literature, which reports only the RD coefficient and not the control or interaction terms, we implement a “manual” standard rectangular RDD for all relevant banks (between $10B and $200B in assets) to present the signs and magnitudes of the control variables that might be of interest to the reader. The results show statistically significant decreases in the Cash ETR (by 0.064 standard deviations), the 3-year Cash and GAAP ETRs and an increase in the tax aggressiveness indices, see Table A4 in the Online Appendix. The results corroborate our baseline findings of an increase in tax planning in stress test banks after the passage of the DFA. However, the effect is smaller in magnitude using the rectangular RDD as it is linear and includes all the possible relevant banks opposed to the optimal bandwidth and triangular kernel automatically implemented in “rdrobust,” thus making the “rdrobust” methodology more robust than the rectangular RDD.
As we explained earlier pertaining to the results in Table 3, the banks included in the optimal bandwidth specification are those closest to the cutoff where the RDD effects are linear. When we relax the optimal bandwidth assumption to include more banks, we experience linear and non-linear effects, resulting in a loss in the significance of our results with an expanded sample.
For example, Boissay and Collard (2016), Acharya et al. (2018) and Cortés et al. (2020) find that the bank loans taken may decrease or remain unchanged. However, banks shift lending away from risky borrowers, indicating that the quality significantly improves.
As in García and Steele (2022), Figure 2 and Table A5 indicate that the standard RDD assumption of no manipulation of the running variable holds in this setting as well.
See McCrary (2008) and García and Steele (2022) for a description of the procedure of the test.
Table A5 presents results for the McCrary manipulation test from 2011 to 2016.
While the Cash ETR is robust to the exclusion of the partial list and the complete list of covariates, the TAI is more sensitive to bank characteristics and displays more significant results with the inclusion of all the control variables.
We thank Brian A. Clark, Christine Dobridge (discussant), Kevin (Yong Kyu) Gam (discussant), Miguel García-Posada Gómez (discussant), John Graham, Martin Jacob, Edward Maydew, Pradeep Muthukrishnan (discussant), Juan Carlos Suárez Serrato, Shasta Shakya (discussant), Anjan Thakor, Rohan G. Williamson, Ryan Wilson, Qiang Wu and session and seminar participants at Rensselaer Polytechnic Institute, the 2022 Academy of Behavioral Finance and Economics Annual Meeting, the 2022 and 2023 Financial Management Association Annual Meetings, the 2021 Vietnam Symposium in Banking and Finance and the 2021 National Tax Association for helpful comments. We are also thankful for participating in the 2019 and 2020 Financial Management Association (FMA) Diversity Emerging Scholar Initiative (DESI). Raffi E. García is grateful for the financial research support received from the American Economic Association Mentoring Program and the Diversity Initiative for Tenure in Economics, both in conjunction with the National Science Foundation. All errors are our own.
The supplementary material for this article can be found online.
References
Acharya, V., Berger, A., & Roman, R. (2018). Lending implications of u.s. bank stress tests: Costs or benefits?. Journal of Financial Intermediation, 34, 58–90. doi: 10.1016/j.jfi.2018.01.004.
Ahnert, L., Vogt, P., Vonhoff, V., & Weigert, F. (2020). Regulatory stress testing and bank performance. European Financial Management, 26(5), 1449–1488. doi: 10.1111/eufm.12267.
Allayannis, G., & Weston, J. P. (2003). Earnings volatility, cash flow volatility, and firm value.
Allen, A., Francis, B. B., Wu, Q., & Zhao, Y. (2016). Analyst coverage and corporate tax aggressiveness. Journal of Banking and Finance, 73, 84–98. doi: 10.1016/j.jbankfin.2016.09.004.
Ananou, F., Chronopoulos, D. K., Tarazi, A., & Wilson, J. O. (2021). Liquidity regulation and bank lending. Journal of Corporate Finance, 69, 101997. doi: 10.1016/j.jcorpfin.2021.101997.
Andries, K., Gallemore, J., & Jacob, M. (2017). The effect of corporate taxation on bank transparency: Evidence from loan loss provisions. Journal of Accounting and Economics, 63(2-3), 307–328. doi: 10.1016/j.jacceco.2017.03.004.
Armstrong, C. S., Blouin, J. L., Jagolinzer, A. D., & Larcker, D. F. (2015). Corporate governance, incentives, and tax avoidance. Journal of Accounting and Economics, 60(1), 1–17. doi: 10.1016/j.jacceco.2015.02.003.
Bahaj, S., & Malherbe, F. (2020). The forced safety effect: How higher capital requirements can increase bank lending. The Journal of Finance, 75(6), 3013–3053. doi: 10.1111/jofi.12958.
Baker, S., Bloom, N., & Davis, S. (2016). Measuring economic policy uncertainty. Quarterly Journal of Economics, 131(4), 1593–1636. doi: 10.1093/qje/qjw024.
Balakrishnan, K., Blouin, J. L., & Guay, W. R. (2019). Tax aggressiveness and corporate transparency. The Accounting Review, 94(1), 45–69. doi: 10.2308/accr-52130.
Balla, E., Romero, J. S., & Rose, M. J. (2012). Loan loss reserve accounting and bank behavior. Richmond Fed Economic Brief, (Mar).
Ballew, H., Iselin, M., & Nicoletti, A. (2021). Accounting-based thresholds and growth decisions in the banking industry. Review of Accounting Studies, 27, 1–43. doi: 10.1007/s11142-021-09589-6.
Bankman, J. (2004). The tax shelter battle. The crisis in tax administration, 9, 13.
Bassett, W., & Berrospide, J. (2017). The impact of stress tests on bank lending. Technical report, Working Paper, Board of Governors of the Federal Reserve System (US) 2017.
Bayar, O., Huseynov, F., & Sardarli, S. (2018). Corporate governance, tax avoidance, and financial constraints. Financial Management, 47(3), 651–677. doi: 10.1111/fima.12208.
Berrospide, J. M., & Edge, R. M. (2019). The effects of bank capital buffers on bank lending and firm activity: What can we learn from five years of stress-test results? Board of Governors of the Federal Reserve System, Finance and Economics Discussion Series 2019-050. 2019.0, 50. doi: 10.17016/feds.2019.050.
Blaylock, B. S. (2016). Is tax avoidance associated with economically significant rent extraction among us firms?. Contemporary Accounting Research, 33(3), 1013–1043. doi: 10.1111/1911-3846.12174.
Blouin, J. (2014). Defining and measuring tax planning aggressiveness. National Tax Journal, 67(4), 875–899. doi: 10.17310/ntj.2014.4.06.
Boissay, F., & Collard, F. (2016). Macroeconomics of bank capital and liquidity regulations.
Boubaker, S., Derouiche, I., & Nguyen, H. (2021). Voluntary disclosure, tax avoidance and family firms. Journal of Management and Governance, 26, 1–30. doi: 10.1007/s10997-021-09601-w.
Bozanic, Z., Hoopes, J. L., Thornock, J. R., & Williams, B. M. (2017). Irs attention. Journal of Accounting Research, 55(1), 79–114. doi: 10.1111/1475-679x.12154.
Calem, P., Correa, R., & Lee, S. J. (2020). Prudential policies and their impact on credit in the United States. Journal of Financial Intermediation, 42(1186), 100826–100854. doi: 10.17016/ifdp.2016.1186.
Calonico, S., Cattaneo, M. D., & Titiunik, R. (2014). Robust nonparametric condence intervals for regression-discontinuity designs. Econometrica, 82(6), 2295–2326. doi: 10.3982/ecta11757.
Chen, S., Chen, X., Cheng, Q., & Shevlin, T. (2010). Are family firms more tax aggressive than non-family firms?. Journal of Financial Economics, 95(1), 41–61. doi: 10.1016/j.jfineco.2009.02.003.
Chen, Y., Huang, S., Pereira, R., & Wang, J. (2011). Corporate tax avoidance and firm opacity. Unpublished Working Paper University of Missouri.
Chen, M.-C., Chang, C.-W., & Lee, M.-C. (2020). The effect of chief financial officers’ accounting expertise on corporate tax avoidance: The role of compensation design. Review of Quantitative Finance and Accounting, 54(1), 273–296. doi: 10.1007/s11156-019-00789-5.
Cheng, C. A., Huang, H. H., Li, Y., & Stanfield, J. (2012). The effect of hedge fund activism on corporate tax avoidance. The Accounting Review, 87(5), 1493–1526. doi: 10.2308/accr-50195.
Cheng, C. A., Francis, B. B., Li, Z., Shen, Y., & Wu, Q. (2023). Do creditor control rights impact corporate tax aggressiveness? Evidence from debt covenant violations. Journal of Business Finance and Accounting. doi: 10.2139/ssrn.4510822.
Chu, Y., Zhang, D., & Zhao, Y. E. (2019). Bank capital and lending: Evidence from syndicated loans. Journal of Financial and Quantitative Analysis, 54(2), 667–694. doi: 10.1017/s0022109018000698.
Clark, B., Francis, B., Garcia, R. E., & Steele, S. (2019). The hawthorne effect in banking. Working Paper.
Cornett, M. M., Minnick, K., Schorno, P. J., & Tehranian, H. (2020). An examination of bank behavior around federal reserve stress tests. Journal of Financial Intermediation, 41, 100789. doi: 10.1016/j.jfi.2018.05.001.
Cortés, K. R., Demyanyk, Y., Li, L., Loutskina, E., & Strahan, P. E. (2020). Stress tests and small business lending. Journal of Financial Economics, 136(1), 260–279. doi: 10.1016/j.jfineco.2019.08.008.
Desai, M. A., & Dharmapala, D. (2006). Corporate tax avoidance and high-powered incentives. Journal of Financial Economics, 79(1), 145–179. doi: 10.1016/j.jfineco.2005.02.002.
Desai, M. A., & Dharmapala, D. (2009). Corporate tax avoidance and firm value. The Review of Economics and Statistics, 91(3), 537–546. doi: 10.1162/rest.91.3.537.
Donohoe, M. P., & Robert Knechel, W. (2014). Does corporate tax aggressiveness influence audit pricing?. Contemporary Accounting Research, 31(1), 284–308. doi: 10.1111/1911-3846.12027.
Dou, Y., Ryan, S. G., & Xie, B. (2018). The real effects of fas 166/167 on banks’ mortgage approval and sale decisions. Journal of Accounting Research, 56(3), 843–882. doi: 10.1111/1475-679x.12204.
Drake, K. D., Lusch, S. J., & Stekelberg, J. (2019). Does tax risk affect investor valuation of tax avoidance?. Journal of Accounting, Auditing and Finance, 34(1), 151–176. doi: 10.1177/0148558x17692674.
Dyreng, S. D., Hanlon, M., & Maydew, E. L. (2008). Long-run corporate tax avoidance. The Accounting Review, 83(1), 61–82. doi: 10.2308/accr.2008.83.1.61.
Dyreng, S. D., Hanlon, M., & Maydew, E. L. (2019). When does tax avoidance result in tax uncertainty?. The Accounting Review, 94(2), 179–203. doi: 10.2308/accr-52198.
Dyreng, S., Jacob, M., Jiang, X., & Müller, M. A. (2021). Tax incidence and tax avoidance.
Edwards, A., Schwab, C., & Shevlin, T. (2016). Financial constraints and cash tax savings. The Accounting Review, 91(3), 859–881. doi: 10.2308/accr-51282.
El-Gazzar, S. M., Pastena, V., & Lilien, S. B. (1989). The use of off-balance sheet financing to circumvent financial covenant restrictions. School of Business and Public Administration, Bernard M. Baruch College.
Fernandes, M., Igan, D., & Pinheiro, M. (2020). Madness in wall street:(what) does the market learn from stress tests?. Journal of Banking and Finance, 112, 105250. doi: 10.1016/j.jbankfin.2017.11.005.
Foster, K. (2017). Yes, cecl affects taxes too. BKD, LLP, pages 1–5.
Gallemore, J., & Labro, E. (2015). The importance of the internal information environment for tax avoidance. Journal of Accounting and Economics, 60(1), 149–167. doi: 10.1016/j.jacceco.2014.09.005.
Gallemore, J., Gipper, B., & Maydew, E. (2019). Banks as tax planning intermediaries. Journal of Accounting Research, 57(1), 169–209. doi: 10.1111/1475-679x.12246.
Gambacorta, L., & Shin, H. S. (2016). Why bank capital matters for monetary policy. BIS Working Paper.
García, R. E., & Steele, S. (2022). Stress testing and bank business patterns: A regression discontinuity study. Journal of Banking and Finance, 135, 105964. doi: 10.1016/j.jbankfin.2020.105964.
García, R. E., Harithsa, J., & Owusu, A. (2024). Adding stress in banking: Stress tests and risk-taking sentiments. Journal of Corporate Finance, 87, 102596. doi: 10.1016/j.jcorpfin.2024.102596.
Garrett, D., & Suárez Serrato, J. C. (2019). How elastic is the demand for tax havens? Evidence from the us possessions corporations tax credit. AEA Papers and Proceedings, 109, 493–99. doi: 10.1257/pandp.20191044.
Gawehn, V. (2020). Banks and corporate income taxation: A review. SSRN 3498843.
Gawehn, V., & Müller, J. (2020). Tax avoidance-are banks any different? SSRN 3418506.
Gelman, A., & Imbens, G. (2014). Why high order polynomials should not Be used in regression discontinuity designs. NBER Working Paper Series. Working Paper 20405.
Goh, B. W., Lee, J., Lim, C. Y., & Shevlin, T. (2016). The effect of corporate tax avoidance on the cost of equity. The Accounting Review, 91(6), 1647–1670. doi: 10.2308/accr-51432.
Goldstein, M. (2017). Banking’s final exam: Stress testing and bank capital reform. Peterson Institute for International Economics.
Goldstein, I., & Sapra, H. (2014). Should banks’ stress test results be disclosed? An analysis of the costs and benefits. Foundations and Trends® in Finance, 8(1), 1–54. doi: 10.1561/0500000038.
Graham, J. R., Hanlon, M., Shevlin, T., & Shroff, N. (2014). Incentives for tax planning and avoidance: Evidence from the field. The Accounting Review, 89(3), 991–1023. doi: 10.2308/accr-50678.
Gulen, H., & Ion, M. (2016). Policy uncertainty and corporate investment. Review of Financial Studies, 29(3), 523–564.
Hanlon, M., & Heitzman, S. (2010). A review of tax research. Journal of Accounting and Economics, 50(2-3), 127–178. doi: 10.1016/j.jacceco.2010.09.002.
Hanlon, M., & Slemrod, J. (2009). What does tax aggressiveness signal? Evidence from stock price reactions to news about tax shelter involvement. Journal of Public Economics, 93(1-2), 126–141. doi: 10.1016/j.jpubeco.2008.09.004.
Hanlon, M., Maydew, E. L., & Saavedra, D. (2017). The taxman cometh: Does tax uncertainty affect corporate cash holdings?. Review of Accounting Studies, 22(3), 1198–1228. doi: 10.1007/s11142-017-9398-y.
Hasan, I., Hoi, C. K. S., Wu, Q., & Zhang, H. (2014). Beauty is in the eye of the beholder: The effect of corporate tax avoidance on the cost of bank loans. Journal of Financial Economics, 113(1), 109–130. doi: 10.1016/j.jfineco.2014.03.004.
Hassan, T. A., Hollander, S., Van Lent, L., & Tahoun, A. (2019). Firm-level political risk: Measurement and effects. Quarterly Journal of Economics, 134(4), 2135–2202. doi: 10.1093/qje/qjz021.
He, G., Ren, H. M., & Taffler, R. (2020). The impact of corporate tax avoidance on analyst coverage and forecasts. Review of Quantitative Finance and Accounting, 54(2), 447–477. doi: 10.1007/s11156-019-00795-7.
Hirtle, B., Kovner, A., & Plosser, M. (2020). The impact of supervision on bank performance. The Journal of Finance, 57(5), 2765–2808. doi: 10.1111/jofi.12964.
Hutchens, M., & Rego, S. O. (2015). Does greater tax risk lead to increased firm risk?. SSRN 2186564.
Hutchens, M., Rego, S. O., & Williams, B. (2020). Tax avoidance, uncertainty, and firm risk. Uncertainty, and Firm Risk, (June 2020).
Imbens, G. W., & Kalyanaraman, K. (2012). Optimal bandwidth choice for the regression discontinuity estimator. The Review of Economic Studies, 19(3), 933–959. doi: 10.1093/restud/rdr043.
Inger, K. K., Meckfessel, M. D., Zhou, M., & Fan, W. (2018). An examination of the impact of tax avoidance on the readability of tax footnotes. Journal of American Taxation Association, 40(1), 1–29. doi: 10.2308/atax-51812.
Jin, J., Liu, Y., Zhang, Z., & Zhao, R. (2022). The impact of financial constraints on banks’ cash tax avoidance. Review of Accounting and Finance, 21(3), 109–129. doi: 10.1108/raf-04-2021-0096.
Kalmenovitz, J. (2019). Regulatory intensity and firm-specific exposure. NYU Stern School of Business.
Kerr, J. N. (2019). Transparency, information shocks, and tax avoidance. Contemporary Accounting Research, 36(2), 1146–1183. doi: 10.1111/1911-3846.12449.
Kohn, D., & Liang, N. (2019). Understanding the effects of the us stress tests. In prepared for the Federal Reserve System Conference, July, volume 9.
Kupiec, P. H. (2020). Policy uncertainty and bank stress testing. Journal of Financial Stability, 51, 100761. doi: 10.1016/j.jfs.2020.100761.
Langenmayr, D., & Reiter, F. (2022). Trading offshore: Evidence on banks’ tax avoidance. The Scandinavian Journal of Economics, 124(3), 797–837. doi: 10.1111/sjoe.12507.
Law, K. K., & Mills, L. F. (2015). Taxes and financial constraints: Evidence from linguistic cues. Journal of Accounting Research, 53(4), 777–819. doi: 10.1111/1475-679x.12081.
Lee, D. S., & Lemieux, T. (2010). Regression discontinuity designs in economics. Journal of Economic Literature, 48(2), 281–355. doi: 10.1257/jel.48.2.281.
Loughran, T., & McDonald, B. (2011). When is a liability not a liability? Textual analysis, dictionaries, and 10-ks. The Journal of Finance, 66(1), 35–65. doi: 10.1111/j.1540-6261.2010.01625.x.
Ludwig, J., & Miller, D. L. (2007). Does head start improve children’s life chance? Evidence from a regression discontinuity design. Quarterly Journal of Economics, 122(1), 159–208. doi: 10.1162/qjec.122.1.159.
Mansi, S., Qi, J., & Shi, H. (2020). Advertising and tax avoidance. Review of Quantitative Finance and Accounting, 54(2), 479–516. doi: 10.1007/s11156-019-00796-6.
McCrary, J. (2008). Manipulation of the running variable in the regression discontinuity design: A density test. Journal of Econometrics, 142(2), 698–714. doi: 10.1016/j.jeconom.2007.05.005.
Meeks, G., & Meeks, J. G. (2014). Why are banks paying so little UK corporation tax?. Fiscal Studies, 35(4), 511–533. doi: 10.1111/j.1475-5890.2014.12040.x.
Merz, J., & Overesch, M. (2016). Profit shifting and tax response of multinational banks. Journal of Banking and Finance, 68, 57–68. doi: 10.1016/j.jbankfin.2016.03.015.
Miller, R., & Ann Ruane, K. (2012). The Dodd-Frank wall street reform and consumer protection act: Title VII, derivatives. [Working paper]. YPFS Documents (Series 1). 511. Available from: https://elischolar.library.yale.edu/ypfs-documents/511/ (accessed 29 June 2023).
Minton, B. A., & Schrand, C. (1999). The impact of cash flow volatility on discretionary investment and the costs of debt and equity financing. Journal of Financial Economics, 54(3), 423–460. doi: 10.1016/s0304-405x(99)00042-2.
Nguyen, J. H. (2020). Tax avoidance and financial statement readability. European Accounting Review, 30(5), 1–24. doi: 10.1080/09638180.2020.1811745.
Nguyen, M., & Nguyen, J. H. (2020). Economic policy uncertainty and firm tax avoidance. Accounting and Finance, 3635–3978.
Nguyen, D. D., Nguyen, L., & Sila, V. (2019). Does corporate culture affect bank risk-taking? Evidence from loan-level data. British Journal of Management, 30(1), 106–133. doi: 10.1111/1467-8551.12300.
Owusu, A., & Gupta, A. (2023). Identifying the risk culture of banks using machine learning. International Journal of Managerial Finance, 20(2), 377–405. doi: 10.1108/IJMF-09-2022-0422.
Paskelian, O. G., & Bell, S. (2013). The tale of two regulations—Dodd-Frank Act and Basel III: A review and comparison of the two regulatory frameworks. Review of Futures Markets, 21(5), 7–29.
Pettersson-Lidbom, P. (2012). Does the size of the legislature affect the size of government? Evidence from two natural experiments. Journal of Public Economics, 96(3-4), 269–279. doi: 10.1016/j.jpubeco.2011.07.004.
Rego, S. O., & Wilson, R. (2012). Equity risk incentives and corporate tax aggressiveness. Journal of Accounting Research, 50(3), 775–810. doi: 10.1111/j.1475-679x.2012.00438.x.
Robinson, J. R., Sikes, S. A., & Weaver, C. D. (2010). Performance measurement of corporate tax departments. The Accounting Review, 85(3), 1035–1064. doi: 10.2308/accr.2010.85.3.1035.
Sahin, C., de Haan, J., & Neretina, E. (2020). Banking stress test effects on returns and risks. Journal of Banking and Finance, 117, 105843. doi: 10.1016/j.jbankfin.2020.105843.
Schandlbauer, A. (2017). How do financial institutions react to a tax increase?. Journal of Financial Intermediation, 30, 86–106. doi: 10.1016/j.jfi.2016.08.002.
Schepens, G. (2016). Taxes and bank capital structure. Journal of Financial Economics, 120(3), 585–600. doi: 10.1016/j.jfineco.2016.01.015.
Scholes, M. S., Wilson, G. P., & Wolfson, M. A. (1992). Firms’ responses to anticipated reductions in tax rates: The tax reform act of 1986, Journal of Accounting Research, 30, 161. doi: 10.2307/2491200.
Schuermann, T. (2014). Stress testing banks. International Journal of Forecasting, 30(3), 717–728. doi: 10.1016/j.ijforecast.2013.10.003.
Schwab, C. M., Stomberg, B., & Williams, B. M. (2022). Effective tax planning. The Accounting Review, 97(1), 413–437. doi: 10.2308/tar-2019-0020.
Shin, H., & Woo, Y. (2017). The effect of tax avoidance on cost of debt capital: Evidence from korea. South African Journal of Business Management, 48(4), 83–89. doi: 10.4102/sajbm.v48i4.45.
Tang, T. Y. (2019). The value implications of tax avoidance across countries. Journal of Accounting, Auditing and Finance, 34(4), 615–638. doi: 10.1177/0148558x17742821.
Trueman, B., & Titman, S. (1988). An explanation for accounting income smoothing. Journal of Accounting Research, 26, 127–139. doi: 10.2307/2491184.
Wahab, N. S. A., & Holland, K. (2012). Tax planning, corporate governance and equity value. The British Accounting Review, 44(2), 111–124. doi: 10.1016/j.bar.2012.03.005.
Wilson, R. J. (2009). An examination of corporate tax shelter participants. The Accounting Review, 84(3), 969–999. doi: 10.2308/accr.2009.84.3.969.